Bayesian Optimization With Unknown Search Space
ADVANCES IN NEURAL INFORMATION PROCESSING SYSTEMS 32 (NIPS 2019)(2019)
摘要
Applying Bayesian optimization in problems wherein the search space is unknown is challenging. To address this problem, we propose a systematic volume expansion strategy for the Bayesian optimization. We devise a strategy to guarantee that in iterative expansions of the search space, our method can find a point whose function value within epsilon of the objective function maximum. Without the need to specify any parameters, our algorithm automatically triggers a minimal expansion required iteratively. We derive analytic expressions for when to trigger the expansion and by how much to expand. We also provide theoretical analysis to show that our method achieves c-accuracy after a finite number of iterations. We demonstrate our method on both benchmark test functions and machine learning hyper-parameter tuning tasks and demonstrate that our method outperforms baselines.
更多查看译文
关键词
bayesian optimization
AI 理解论文
溯源树
样例
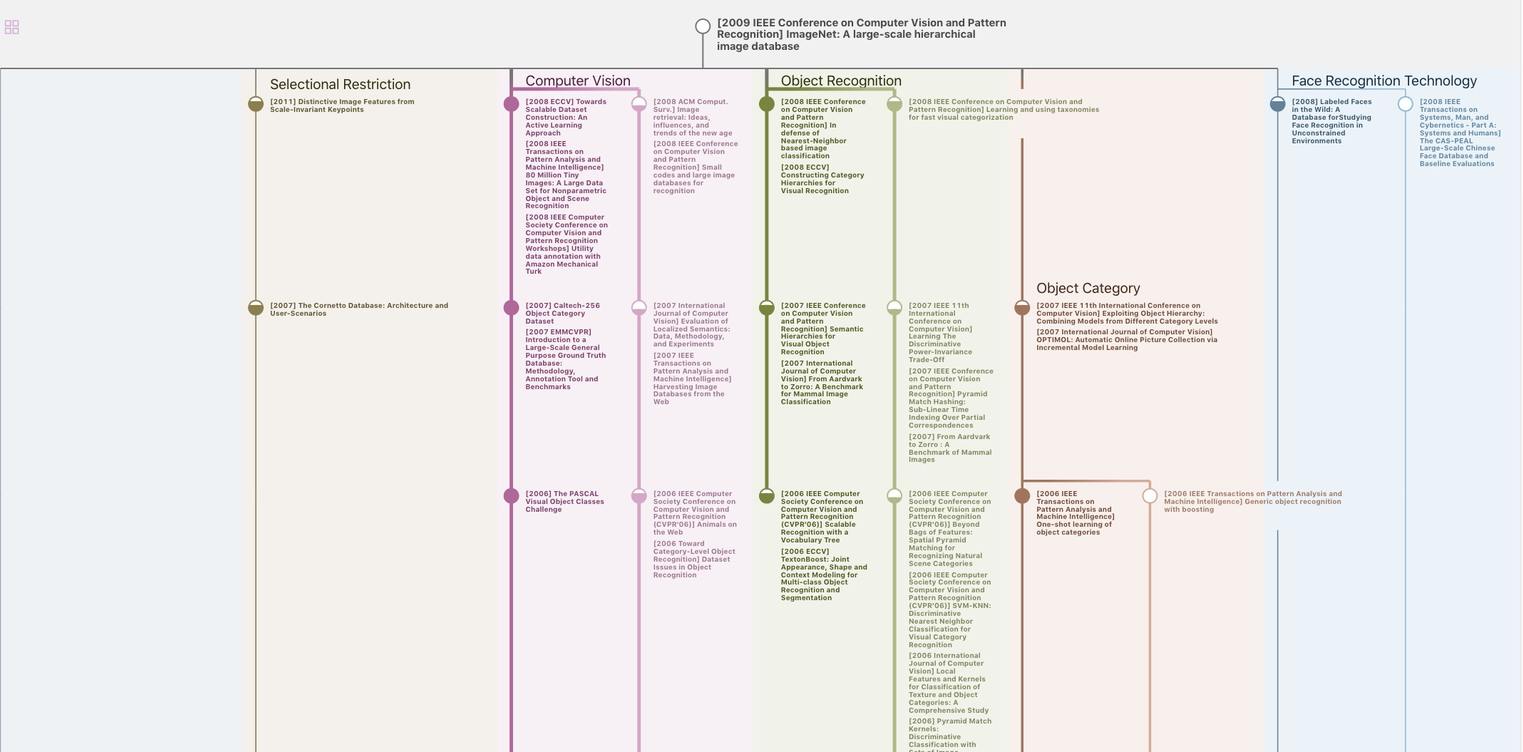
生成溯源树,研究论文发展脉络
Chat Paper
正在生成论文摘要