Probabilistic Active Learning: an Online Framework for Structural Health Monitoring
Journal of physics Conference series(2019)
Abstract
A novel, probabilistic framework for the classification, investigation and labelling of data is suggested as an online strategy for Structural Health Monitoring (SHM). A critical issue for data-based SHM is a lack of descriptive labels (for measured data), which correspond to the condition of the monitored system. For many applications, these labels are costly and/or impractical to obtain, and as a result, conventional supervised learning is not feasible. This fact forces a dependence on outlier analysis, or one-class classifiers, in practical applications, as a means of damage detection. The model suggested in this work, however, allows for the definition of a multi-class classifier, to aid both damage detection and identification, while using a limited number of the most informative labelled data. The algorithm is applied to three datasets in the online setting; the Z24 bridge data, a machining (acoustic emission) dataset, and measurements from ground vibration aircraft tests. In the experiments, active learning is shown to improve the online classification performance for damage detection and classification. (C) 2019 The Authors. Published by Elsevier Ltd. This is an open access article under the CC BY license (http://creativecommons.org/licenses/by/4.0/).
MoreTranslated text
Key words
Damage detection,Pattern recognition,Semi-supervised learning,Structural health monitoring
AI Read Science
Must-Reading Tree
Example
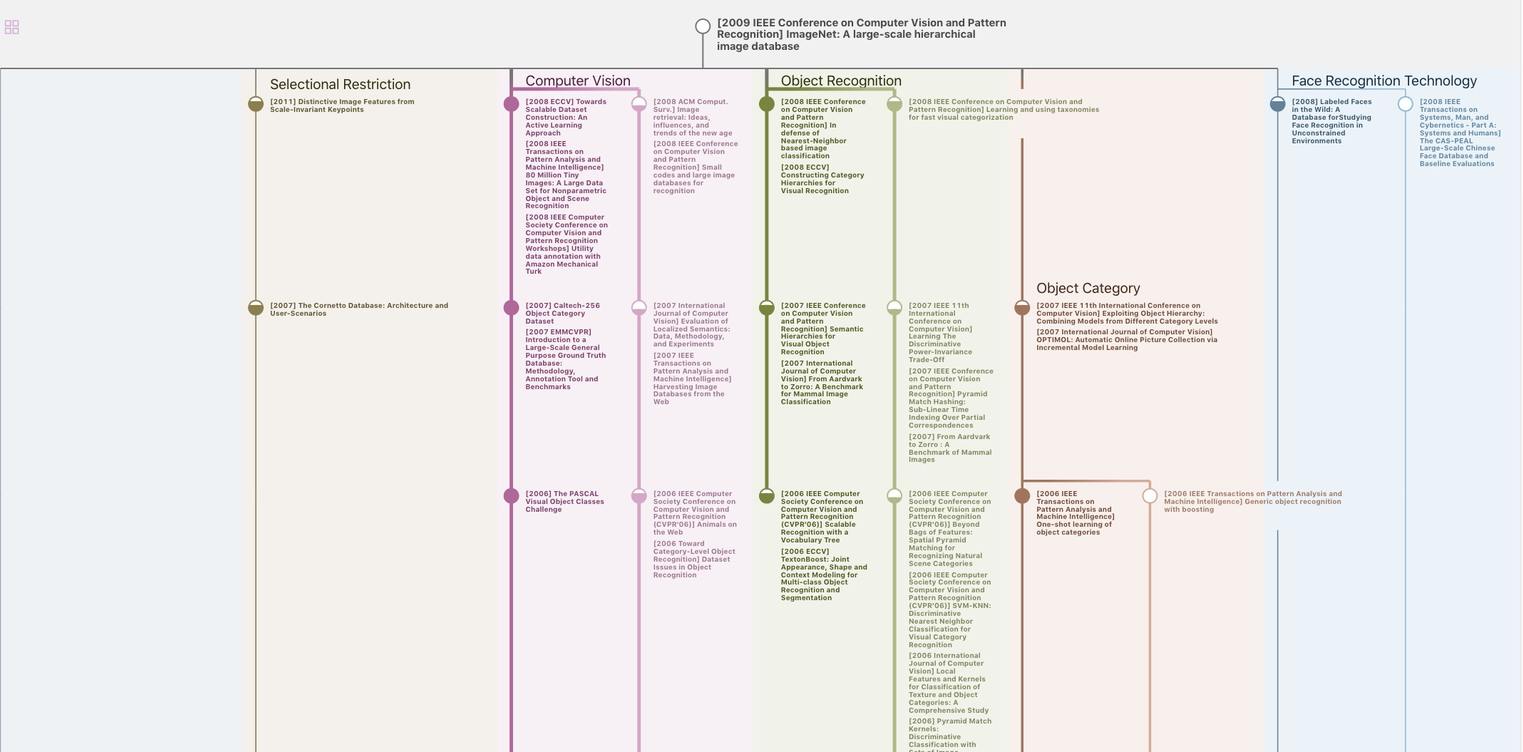
Generate MRT to find the research sequence of this paper
Chat Paper
Summary is being generated by the instructions you defined