Multiple Kernel Learning By Empirical Target Kernel
INTERNATIONAL JOURNAL OF WAVELETS MULTIRESOLUTION AND INFORMATION PROCESSING(2020)
摘要
Multiple kernel learning (MKL) aims at learning an optimal combination of base kernels with which an appropriate hypothesis is determined on the training data. MKL has its flexibility featured by automated kernel learning, and also reflects the fact that typical learning problems often involve multiple and heterogeneous data sources. Target kernel is one of the most important parts of many MKL methods. These methods find the kernel weights by maximizing the similarity or alignment between weighted kernel and target kernel. The existing target kernels implement a global manner, which (1) defines the same target value for closer and farther sample pairs, and inappropriately neglects the variation of samples; (2) is independent of training data, and is hardly approximated by base kernels. As a result, maximizing the similarity to the global target kernel could make these pre-specified kernels less effectively utilized, further reducing the classification performance. In this paper, instead of defining a global target kernel, a localized target kernel is calculated for each sample pair from the training data, which is flexible and able to well handle the sample variations. A new target kernel named empirical target kernel is proposed in this research to implement this idea, and three corresponding algorithms are designed to efficiently utilize the proposed empirical target kernel. Experiments are conducted on four challenging MKL problems. The results show that our algorithms outperform other methods, verifying the effectiveness and superiority of the proposed methods.
更多查看译文
关键词
Multiple kernel learning, target kernel, classification
AI 理解论文
溯源树
样例
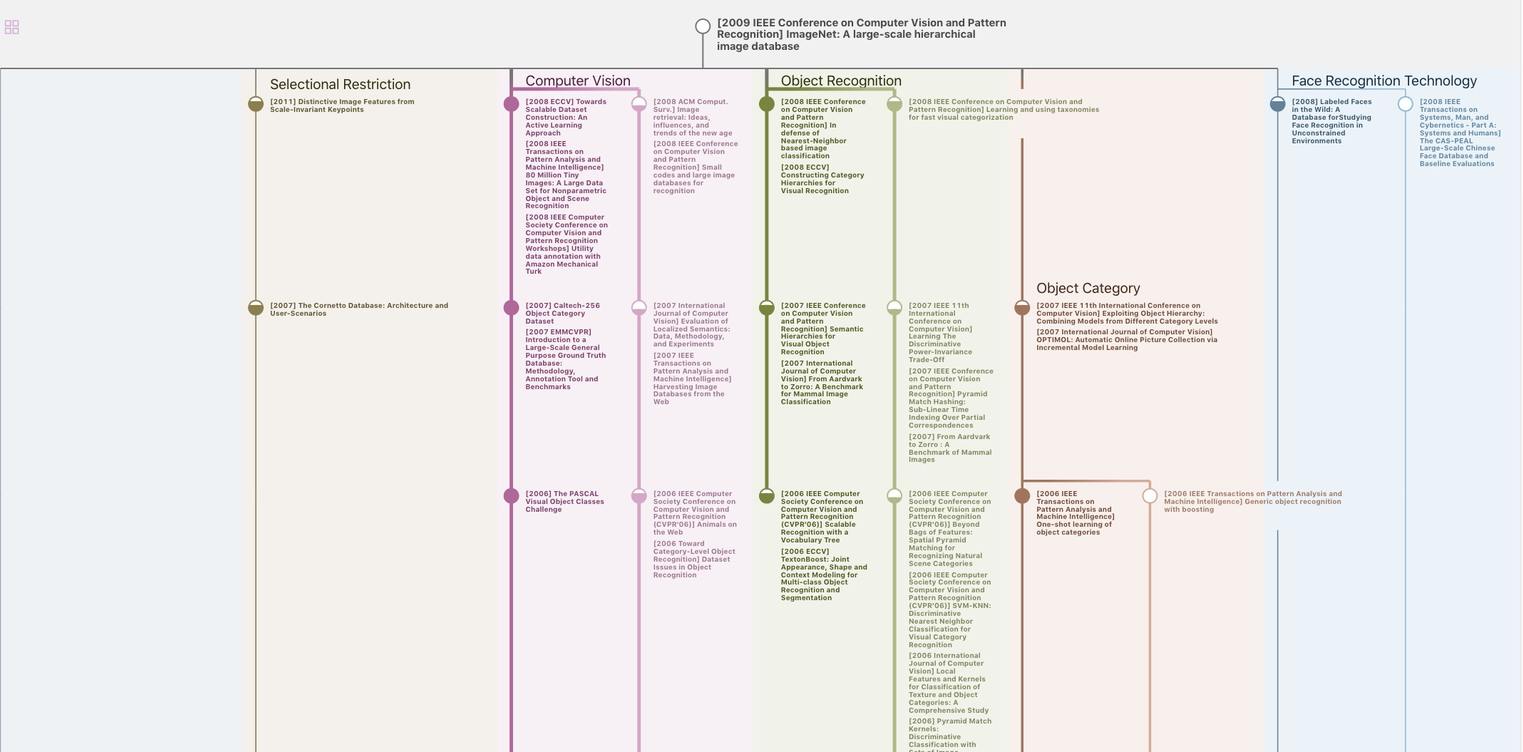
生成溯源树,研究论文发展脉络
Chat Paper
正在生成论文摘要