Distributional Reward Decomposition for Reinforcement Learning
NeurIPS(2019)
Abstract
Many reinforcement learning (RL) tasks have specific properties that can be lever-aged to modify existing RL algorithms to adapt to those tasks and further improve performance, and a general class of such properties is the multiple reward channel. In those environments the full reward can be decomposed into sub-rewards obtained from different channels. Existing work on reward decomposition either requires prior knowledge of the environment to decompose the full reward, or decomposes reward without prior knowledge but with degraded performance. In this paper, we propose Distributional Reward Decomposition for Reinforcement Learning (DRDRL), a novel reward decomposition algorithm which captures the multiple reward channel structure under distributional setting. Empirically, our method captures the multi-channel structure and discovers meaningful reward decomposition, without any requirements on prior knowledge. Consequently, our agent achieves better performance than existing methods on environments with multiple reward channels.
MoreTranslated text
Key words
reinforcement learning,specific properties
AI Read Science
Must-Reading Tree
Example
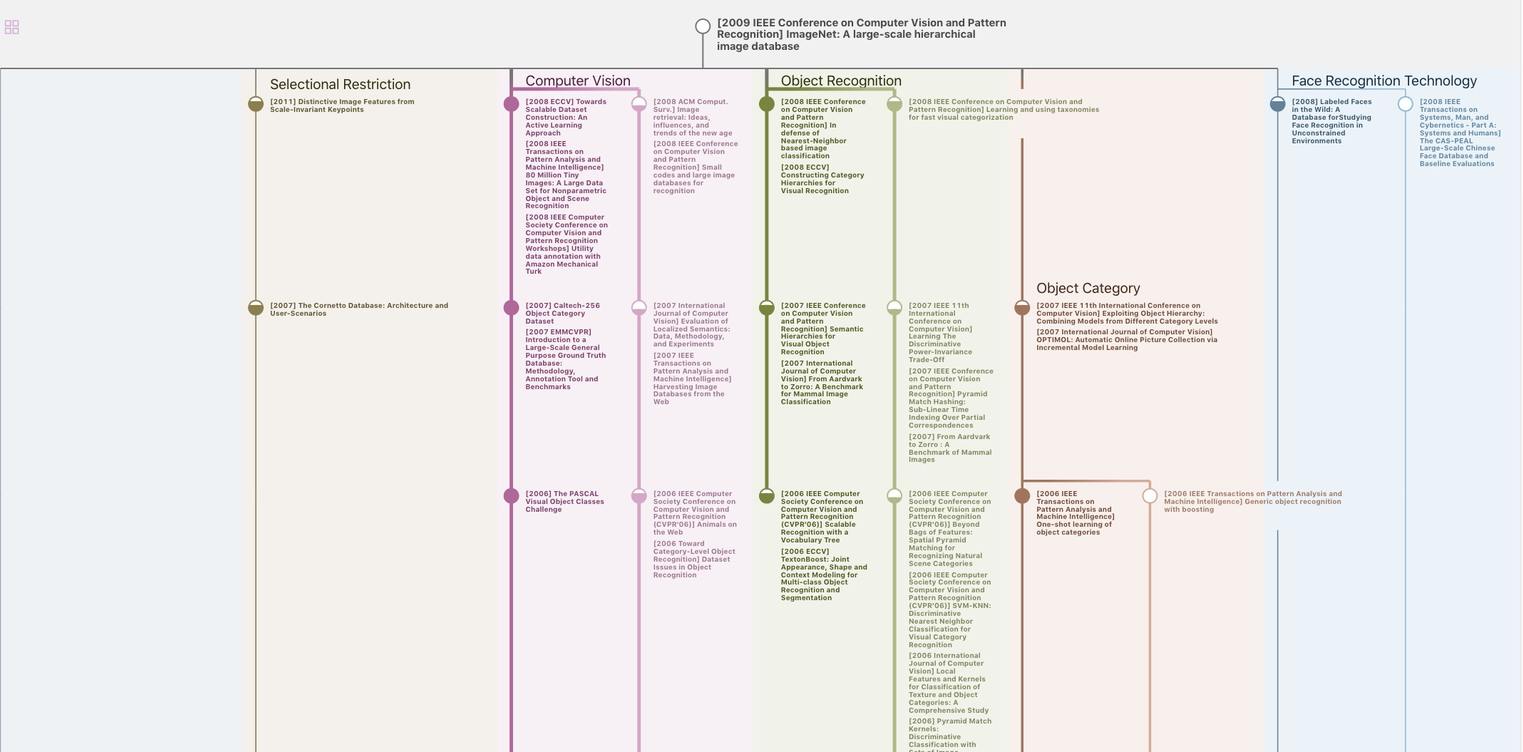
Generate MRT to find the research sequence of this paper
Chat Paper
Summary is being generated by the instructions you defined