Scale-aware Monocular SLAM Based on Convolutional Neural Network
2018 IEEE International Conference on Information and Automation (ICIA)(2018)
摘要
Remarkable performance has been achieved using the state-of-the-art monocular Simultaneous Localization and Mapping (SLAM) algorithms. However, due to the scale ambiguity limitation of monocular vision, the existing monocular SLAM systems can not directly restore the absolute scale in unknown environments. Given the amazing results in the field of depth estimation from Convolutional Neural Networks (CNNs), we propose a CNN-based monocular SLAM, where we naturally combine the CNN-predicted depth maps together with the monocular ORB-SLAM, overcoming the scale ambiguity limitation of the monocular SLAM. We test our method using the popular KITTI odometry benchmark, and the experimental results show that the overall performance of average translational and rotational error can reach 2.00% and 0.0051°/m. In addition, our approach can work well under the pure rotation motion, which shows the robustness and high accuracy of the proposed algorithm.
更多查看译文
关键词
Monocular SLAM,Convolutional Neural Networks,scale ambiguity,depth estimation.
AI 理解论文
溯源树
样例
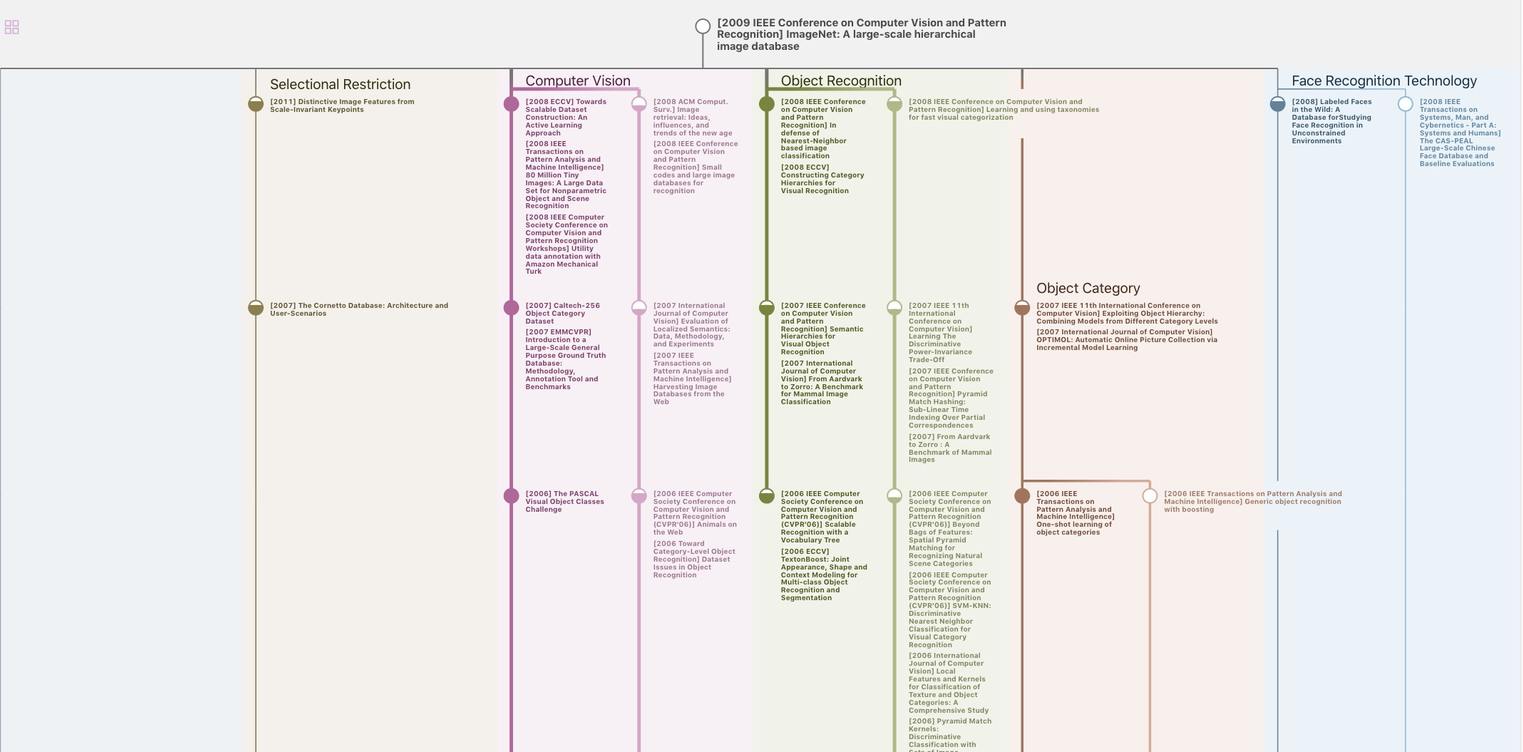
生成溯源树,研究论文发展脉络
Chat Paper
正在生成论文摘要