Breaking The Glass Ceiling For Embedding-Based Classifiers For Large Output Spaces
ADVANCES IN NEURAL INFORMATION PROCESSING SYSTEMS 32 (NIPS 2019)(2019)
摘要
In extreme classification settings, embedding-based neural network models are currently not competitive with sparse linear and tree-based methods in terms of accuracy. Most prior works attribute this poor performance to the low-dimensional bottleneck in embedding-based methods. In this paper, we demonstrate that theoretically there is no limitation to using low-dimensional embedding-based methods, and provide experimental evidence that overfitting is the root cause of the poor performance of embedding-based methods. These findings motivate us to investigate novel data augmentation and regularization techniques to mitigate overfitting. To this end, we propose GLaS, a new regularizer for embedding-based neural network approaches. It is a natural generalization from the graph Laplacian and spread-out regularizers, and empirically it addresses the drawback of each regularizer alone when applied to the extreme classification setup. With the proposed techniques, we attain or improve upon the state-of-the-art on most widely tested public extreme classification datasets with hundreds of thousands of labels.
更多查看译文
关键词
graph laplacian,root cause,glass ceiling
AI 理解论文
溯源树
样例
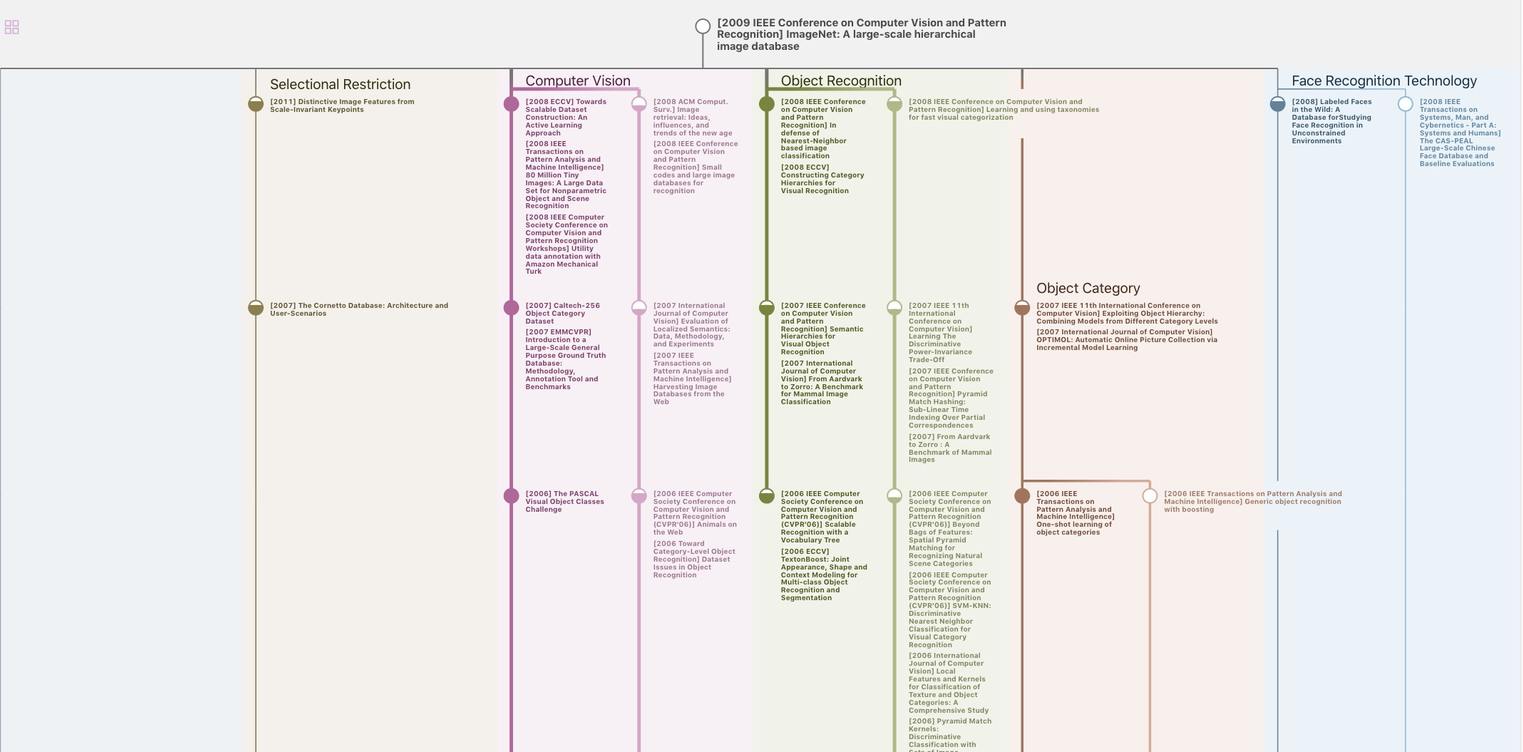
生成溯源树,研究论文发展脉络
Chat Paper
正在生成论文摘要