Classification Of Structural Mri Images In Adhd Using 3d Fractal Dimension Complexity Map
2019 IEEE INTERNATIONAL CONFERENCE ON IMAGE PROCESSING (ICIP)(2019)
摘要
Attention deficit hyperactivity disorder (ADHD) is a common mental-health disorder in adolescent groups. Successful automatic diagnosis of ADHD based on features extracted from magnetic resonance imaging (MRI) data, would provide reference information for treating. Previous researches have shown gray matter (GM) of some anatomical brain structures will increase in ADHD subjects. Fractal analysis has been widely used in texture image processing and fractal dimension is capable of representing intrinsic structural information of images. With large-scale MRI data becoming publicly available, deep-learning methods for ADHD diagnosis become feasible. This paper proposes a novel classification approach using 3D fractal dimension complexity map (FDCM) for ADHD automatic diagnosis. We calculate the Hausdorff fractal dimension of GM density data extracted from structural MRI data. Subsequently, we design a 3 dimensional convolutional neural network (3D-CNN) for extracting features from FDCM then judging ADHD and TDC. Our model is evaluated on the hold-out testing data of the ADHD-200 global competition and performance outperforms previous approaches based on structural MRI data.
更多查看译文
关键词
Attention deficit hyperactive disorder, 3D fractal dimension based complexity map, Structural MRI, 3D convolutional neural network
AI 理解论文
溯源树
样例
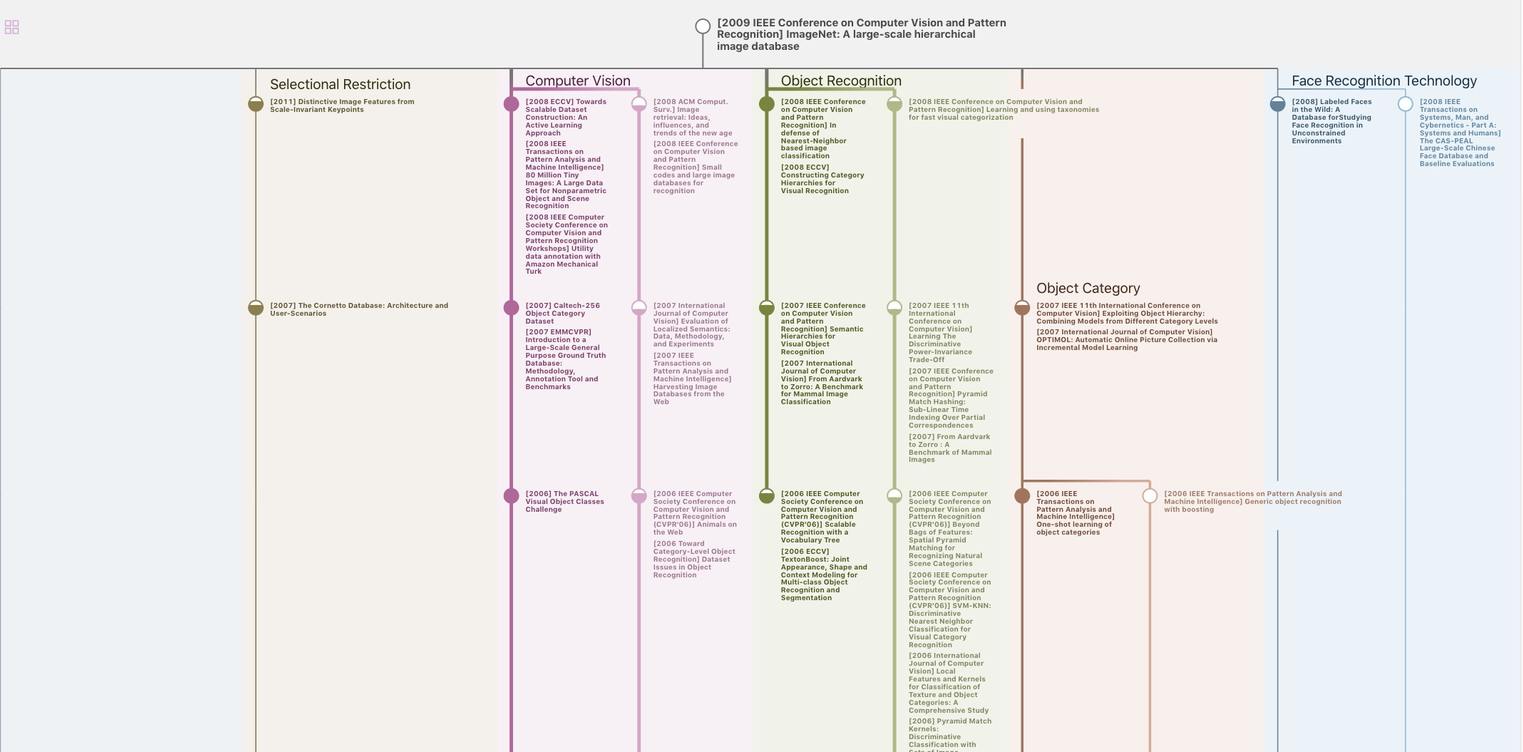
生成溯源树,研究论文发展脉络
Chat Paper
正在生成论文摘要