Full-Reference Metric Adaptive Image Denoising
2019 IEEE INTERNATIONAL CONFERENCE ON IMAGE PROCESSING (ICIP)(2019)
摘要
The performance of image denoising algorithms generally depends largely on the selection of the parameters. We address the problem of optimizing the denoising parameters to achieve maximum denoising performance. Most existing methods for no-reference denoising parameter optimization either use the estimated image noise or individual no-reference image quality evaluation metrics. In the paper, we introduce a natural image statistics based on the generalized Gaussian distribution and an elastic net regularization regression model. Consequently, we propose their use for performing no-reference parameter optimization in the state-of-the-art BM3D denoising algorithm, adaptively depending on which of the following most widely used full-reference image quality evaluation metrics is optimized: FSIM, SSIM, MS-SSIM, without any knowledge of the noise distribution.
更多查看译文
关键词
Denoising parameter optimization, BM3D, full-reference image quality, Kullback-Leibler divergence
AI 理解论文
溯源树
样例
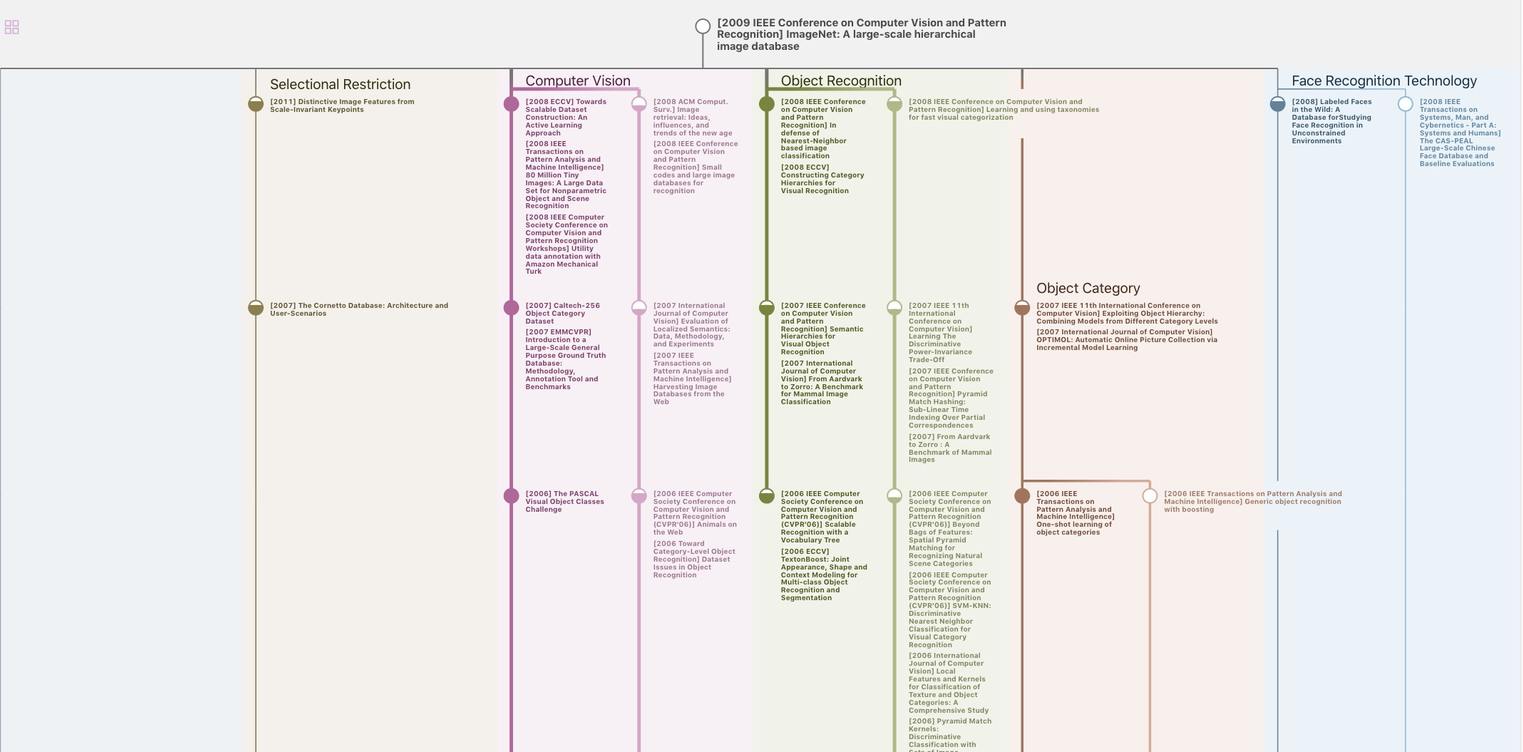
生成溯源树,研究论文发展脉络
Chat Paper
正在生成论文摘要