DEEP U-NET REGRESSION AND HAND-CRAFTED FEATURE FUSION FOR ACCURATE BLOOD VESSEL SEGMENTATION
international conference on image processing(2019)
摘要
Automated curvilinear image segmentation is a crucial step to characterize and quantify the morphology of blood vessels across scale. We propose a dual pipeline RF OFB+U-NET that fuses U-Net deep learning features with a low level image feature filter bank using the random forests classifier for vessel segmentation. We modify the U-Net CNN architecture to provide a foreground vessel regression likelihood map that is used to segment both arteriole and venule blood vessels in mice dura mater tissues. The hybrid approach combining both hand-crafted and learned features was tested on 60 epifluorescence microscopy images and improved the segmentation of thin vessel structures by nearly 5% using the Dice similarity coefficient compared to U-Net.
更多查看译文
关键词
Semantic vessel segmentation, deep learning, histogram equalization, random forests, U-Net
AI 理解论文
溯源树
样例
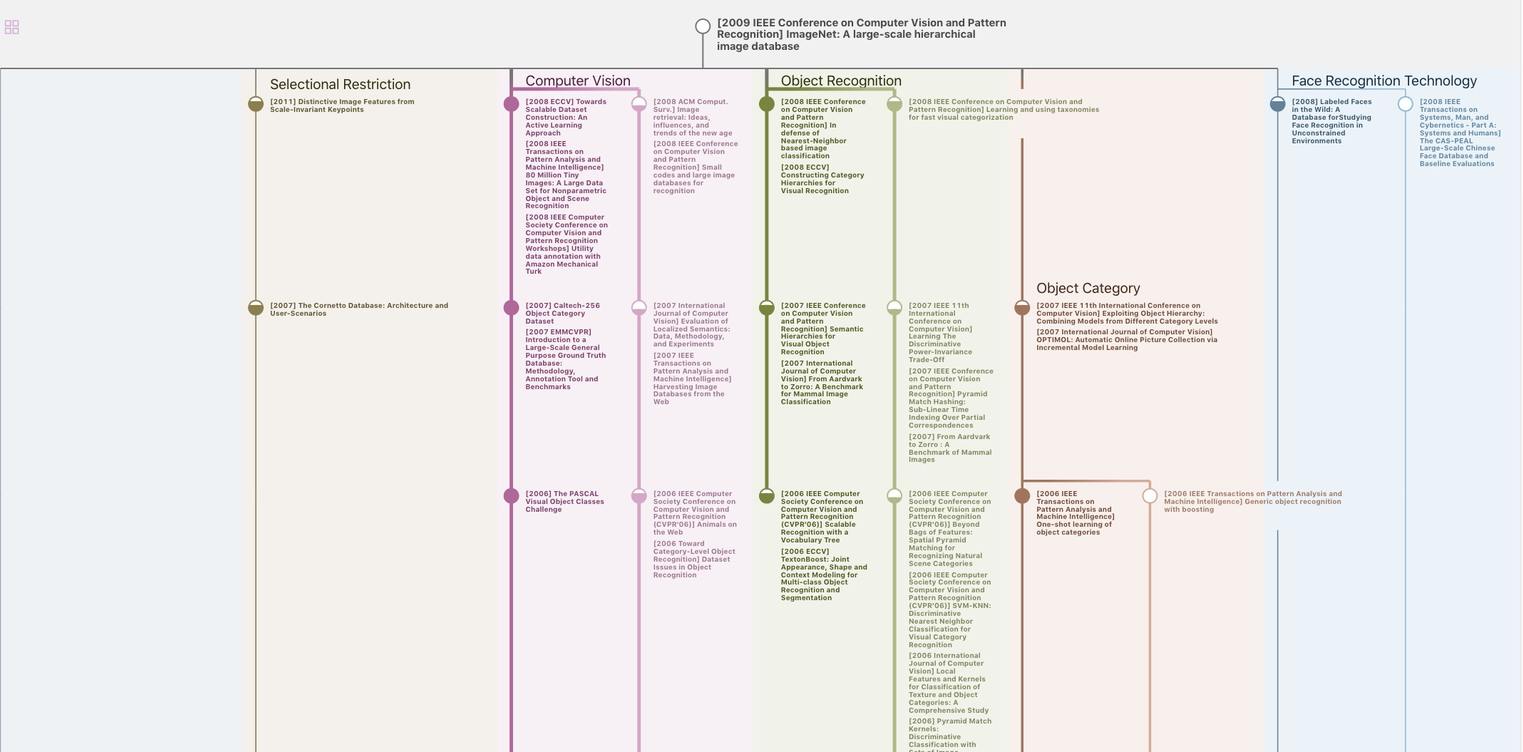
生成溯源树,研究论文发展脉络
Chat Paper
正在生成论文摘要