Two Generator Game: Learning to Sample via Linear Goodness-of-Fit Test
ADVANCES IN NEURAL INFORMATION PROCESSING SYSTEMS 32 (NIPS 2019)(2019)
摘要
Learning the probability distribution of high-dimensional data is a challenging problem. To solve this problem, we formulate a deep energy adversarial network (DEAN), which casts the energy model learned from real data into an optimization of a goodness-of-fit (GOF) test statistic. DEAN can be interpreted as a GOF game between two generative networks, where one explicit generative network learns an energy-based distribution that fits the real data, and the other implicit generative network is trained by minimizing a GOF test statistic between the energy-based distribution and the generated data, such that the underlying distribution of the generated data is close to the energy-based distribution. We design a two-level alternative optimization procedure to train the explicit and implicit generative networks, such that the hyper-parameters can also be automatically learned. Experimental results show that DEAN achieves high quality generations compared to the state-of-the-art approaches.
更多查看译文
关键词
probability distribution,probability distributions,test statistic,test statistics
AI 理解论文
溯源树
样例
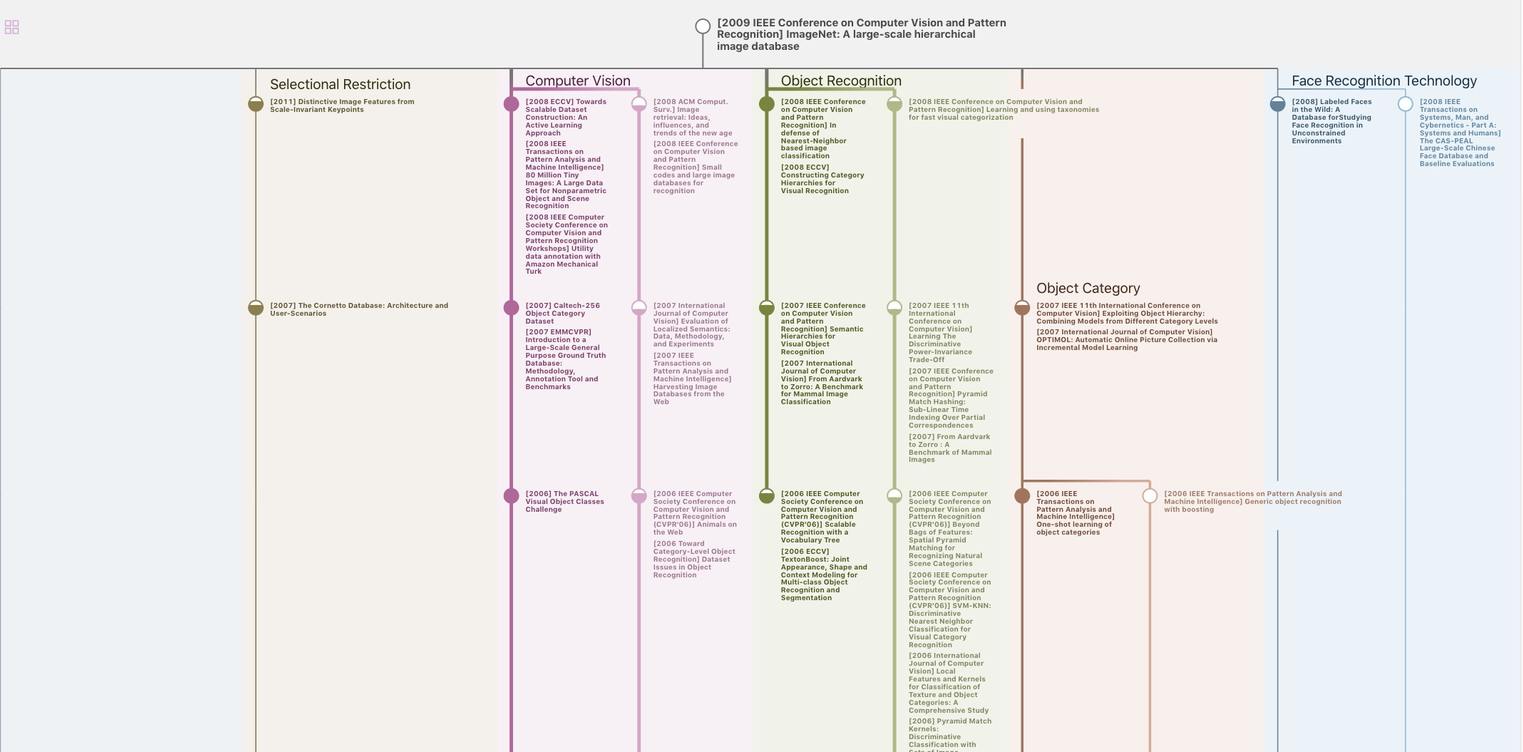
生成溯源树,研究论文发展脉络
Chat Paper
正在生成论文摘要