Compression Artifact Removal With Stacked Multi-Context Channel-Wise Attention Network
2019 IEEE INTERNATIONAL CONFERENCE ON IMAGE PROCESSING (ICIP)(2019)
摘要
Image compression plays an important role in saving disk storage and transmission bandwidth. Among traditional compression standards, JPEG is one of the commonly used standards in lossy image compression. However, the decompressed JPEG images usually have inevitable artifacts due to the quantization step, especially at low bitrate. Many recent works leverage deep learning networks to remove the JPEG artifacts and have achieved notable progress. In this paper, we propose a stacked multi-context channel-wise attention model. The channel-wise attention adaptively integrates features along the channel dimension given a set of feature maps. We apply multiple context-based channel attentions to enable the network to capture features from different resolutions. The entire architecture is trained progressively from the image space of low quality factor to that of high quality factor. Experiments show that we can achieve the state-of-the-art performance with lower complexity.
更多查看译文
关键词
Compression artifact removal, image restoration, hourglass network, attention
AI 理解论文
溯源树
样例
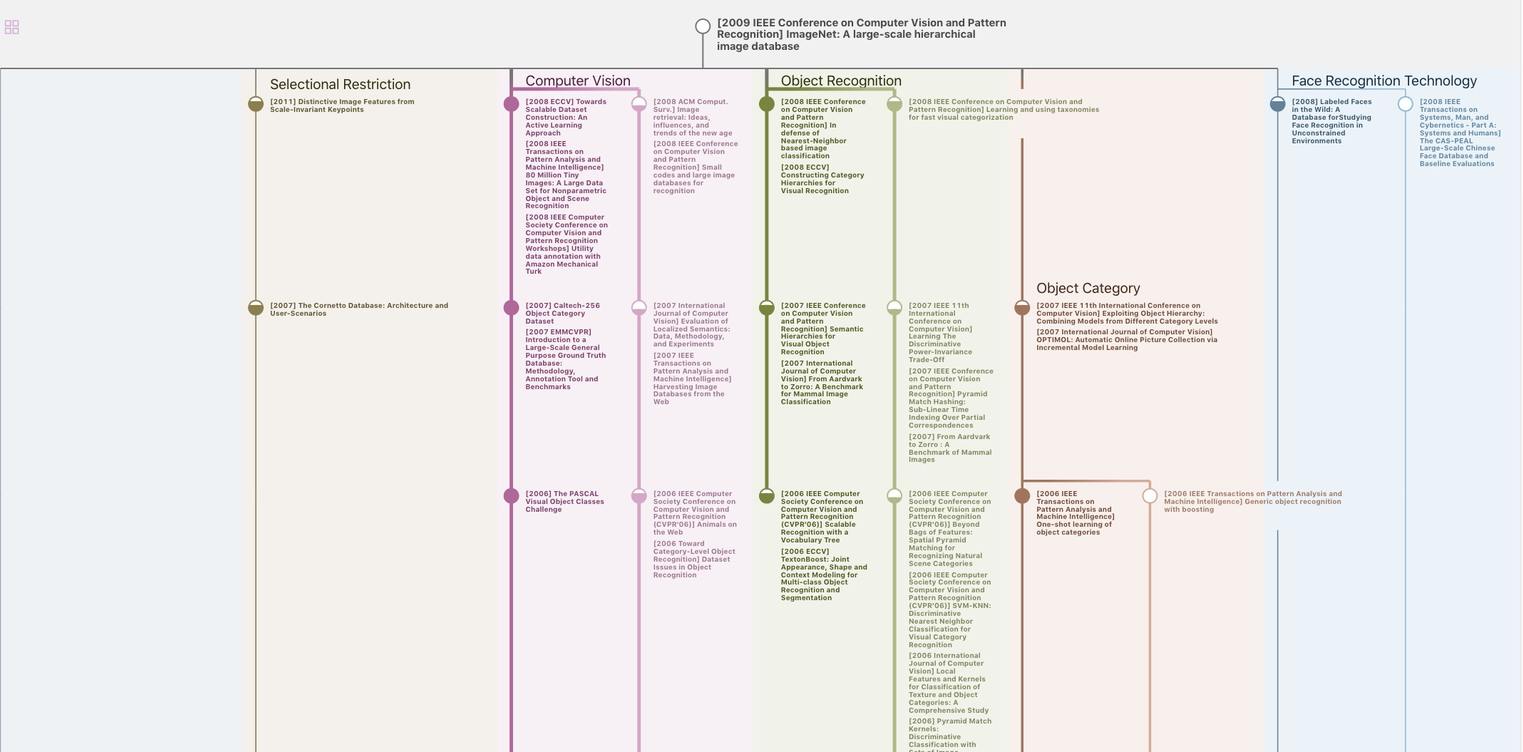
生成溯源树,研究论文发展脉络
Chat Paper
正在生成论文摘要