A Flexible Deep CNN Framework for Image Restoration
IEEE Transactions on Multimedia(2020)
摘要
Image restoration is a long-standing problem in image processing and low-level computer vision. Recently, discriminative convolutional neural network (CNN)-based approaches have attracted considerable attention due to their superior performance. However, most of these frameworks are designed for one specific image restoration task; hence, they seldom show high performance on other image restoration tasks. To address this issue, we propose a flexible deep CNN framework that exploits the frequency characteristics of different types of artifacts. Hence, the same approach can be employed for a variety of image restoration tasks by adjusting the architecture. For reducing the artifacts with similar frequency characteristics, a quality enhancement network that adopts residual and recursive learning is proposed. Residual learning is utilized to speed up the training process and boost the performance; recursive learning is adopted to significantly reduce the number of training parameters as well as boost the performance. Moreover, lateral connections transmit the extracted features between different frequency streams via multiple paths. One aggregation network combines the outputs of these streams to further enhance the restored images. We demonstrate the capabilities of the proposed framework with three representative applications: image compression artifacts reduction (CAR), image denoising, and single image super-resolution (SISR). Extensive experiments confirm that the proposed framework outperforms the state-of-the-art approaches on benchmark datasets for these applications.
更多查看译文
关键词
Training,Image restoration,Image coding,Automobiles,Task analysis,Transform coding,Image denoising
AI 理解论文
溯源树
样例
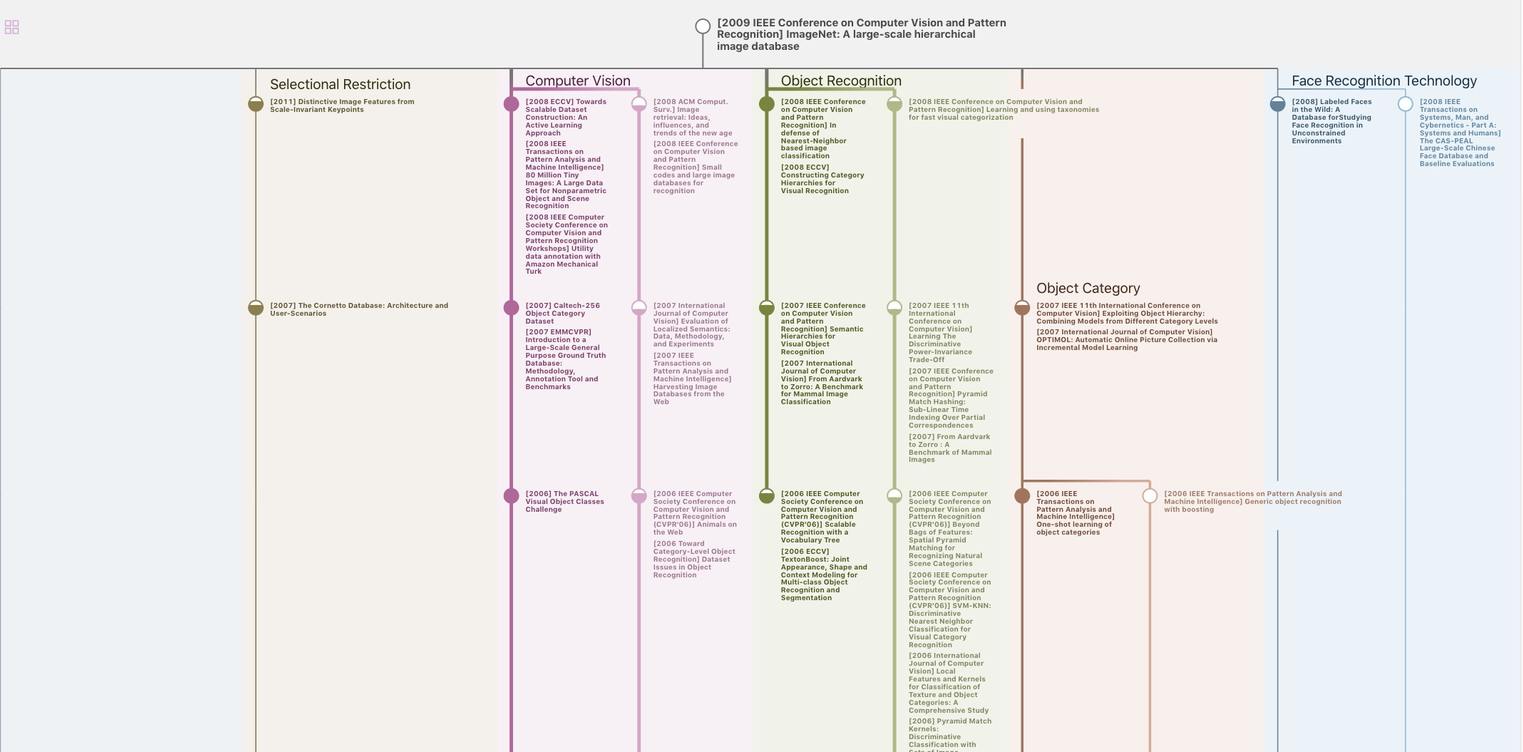
生成溯源树,研究论文发展脉络
Chat Paper
正在生成论文摘要