Learning Super-Resolution Coherent Facial Features Using Nonlinear Multiset Pls For Low-Resolution Face Recognition
2019 IEEE INTERNATIONAL CONFERENCE ON IMAGE PROCESSING (ICIP)(2019)
摘要
Face hallucination (FH) is an effective technique for super-resolving low-resolution (LR) face images. In real-world applications, a face image usually has multiple distinct low resolutions. Most existing FH methods can not effectively deal with multiple LR views simultaneously. To solve this issue, we present a multi-set partial least squares (MPLS) approach and its kernel extension for jointly learning the nonlinear consistency of multi-resolution facial features. With nonlinear MPLS, we present a novel simultaneous super-resolution coherent facial feature method for the face images with multiple LRs, which has capacity of jointly learning the nonlinear relationships between multiple facial resolutions. Experimental results demonstrate the effectiveness and robustness of our proposed FH method.
更多查看译文
关键词
Face Hallucination, Partial Least Squares, Face Recognition, Nonlinear Feature
AI 理解论文
溯源树
样例
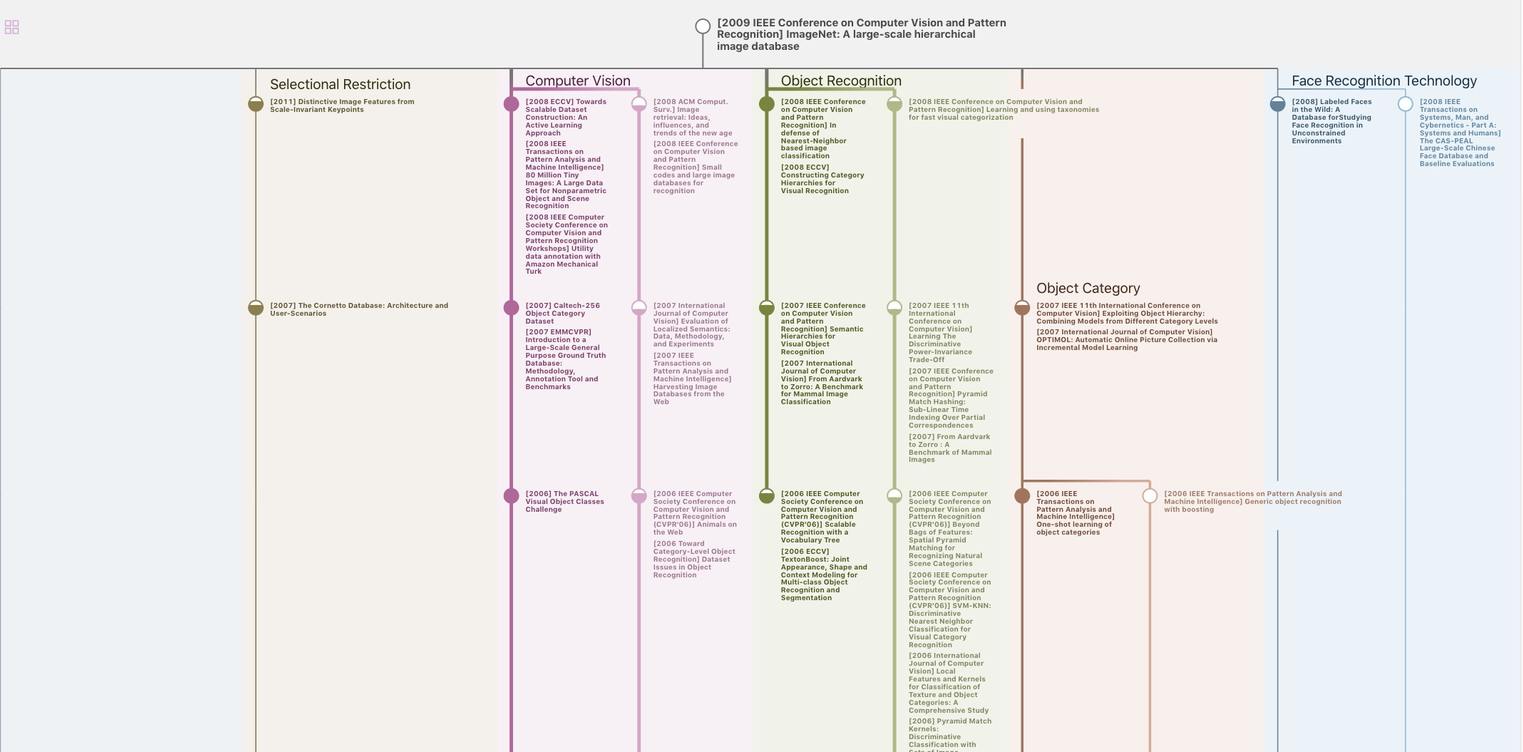
生成溯源树,研究论文发展脉络
Chat Paper
正在生成论文摘要