Source-Constraint Adversarial Domain Adaptation
2019 IEEE INTERNATIONAL CONFERENCE ON IMAGE PROCESSING (ICIP)(2019)
摘要
Adversarial adaptation has made great contributions to transfer learning, while the adversarial training strategy lacks of stability when reducing the discrepancy between domains. In this paper, we propose a novel Source-constraint Adversarial Domain Adaptation (SADA) method, which jointly use adversarial adaptation and maximum mean discrepancy (MMD) so that the method can be easily optimized by gradient descent. Furthermore, motivated by metric learning, our method introduces metric loss to constrain the structure of source domain, which explicitly increases the inter-class distance and decreases the intra-class distance. As a result, SADA can not only reduce the domain discrepancy, but also make the extracted features become more domain-invariant and discriminative. We show that our model yields state of the art results on standard datasets.
更多查看译文
关键词
transfer learning, domain adaptation, adversarial network, metric learning
AI 理解论文
溯源树
样例
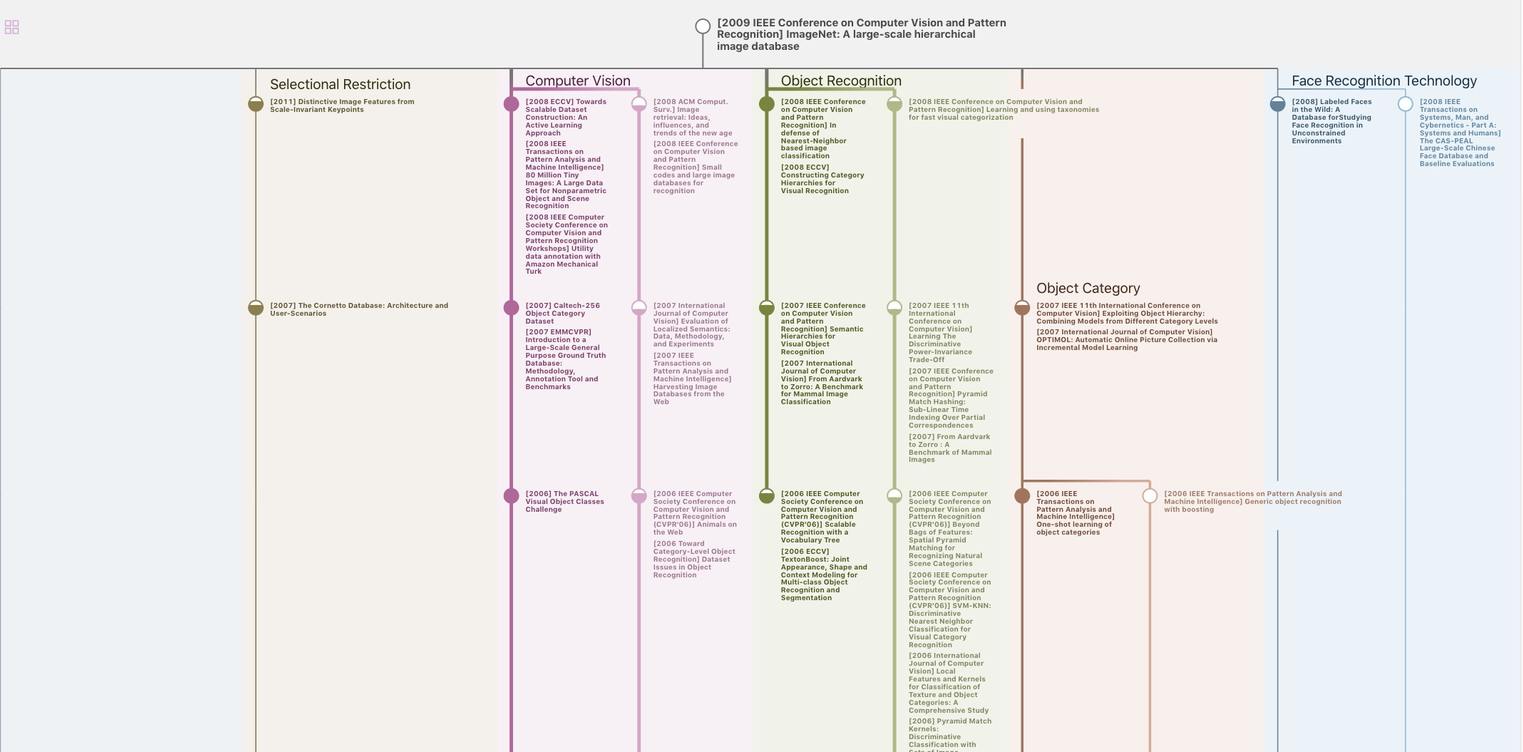
生成溯源树,研究论文发展脉络
Chat Paper
正在生成论文摘要