Latent Ordinary Differential Equations for Irregularly-Sampled Time Series
NeurIPS(2019)
摘要
Time series with non-uniform intervals occur in many applications, and are difficult to model using standard recurrent neural networks (RNNs). We generalize RNNs to have continuous-time hidden dynamics defined by ordinary differential equations (ODEs), a model we call ODE-RNNs. Furthermore, we use ODE-RNNs to replace the recognition network of the recently-proposed Latent ODE model. Both ODE-RNNs and Latent ODEs can naturally handle arbitrary time gaps between observations, and can explicitly model the probability of observation times using Poisson processes. We show experimentally that these ODE-based models outperform their RNN-based counterparts on irregularly-sampled data.
更多查看译文
关键词
time series
AI 理解论文
溯源树
样例
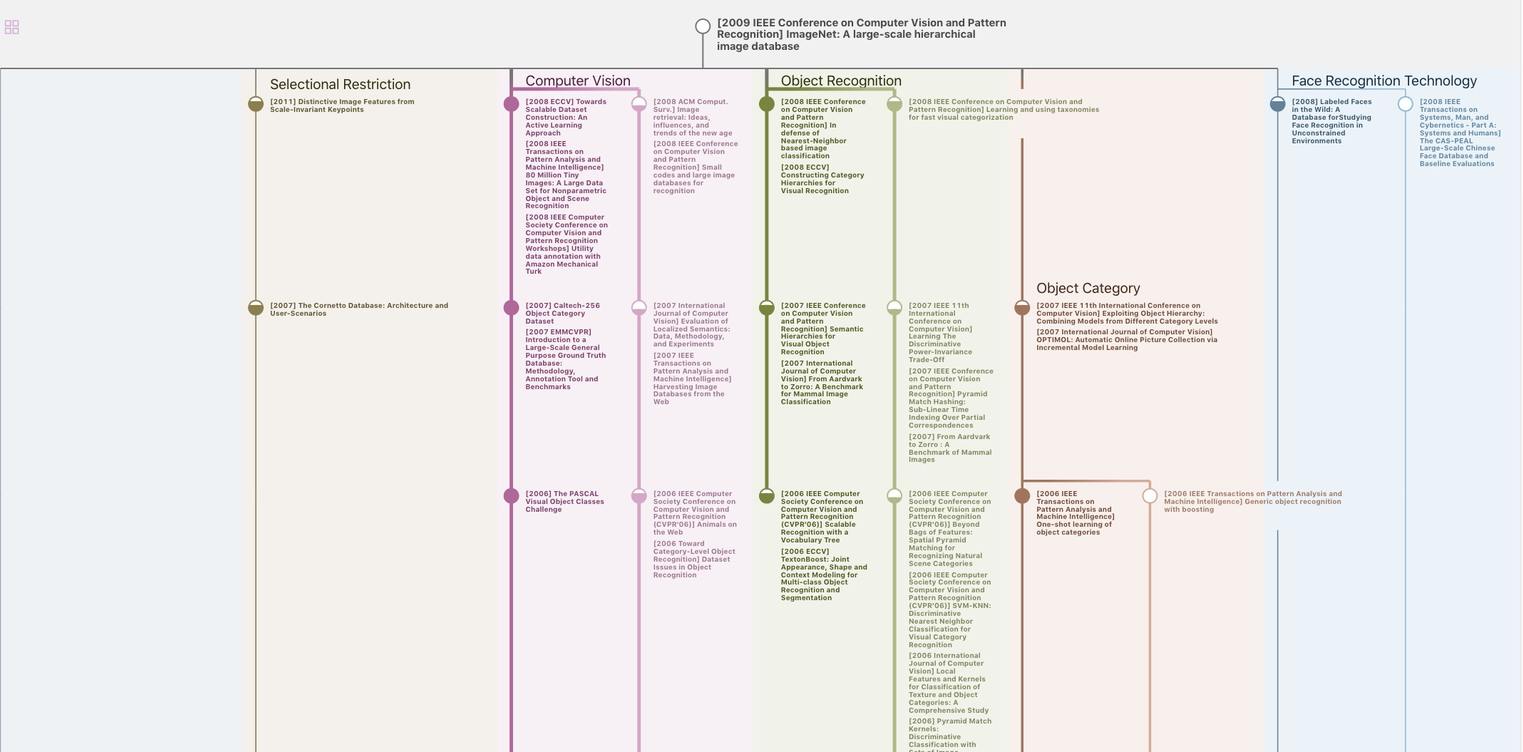
生成溯源树,研究论文发展脉络
Chat Paper
正在生成论文摘要