Compact Representation of a Multi-dimensional Combustion Manifold Using Deep Neural Networks
european conference on machine learning(2019)
摘要
The computational challenges in turbulent combustion simulations stem from the physical complexities and multi-scale nature of the problem which make it intractable to compute scale-resolving simulations. For most engineering applications, the large scale separation between the flame (typically sub-millimeter scale) and the characteristic turbulent flow (typically centimeter or meter scale) allows us to evoke simplifying assumptions–such as done for the flamelet model–to pre-compute all the chemical reactions and map them to a low-order manifold. The resulting manifold is then tabulated and looked-up at run-time. As the physical complexity of combustion simulations increases (including radiation, soot formation, pressure variations etc.) the dimensionality of the resulting manifold grows which impedes an efficient tabulation and look-up. In this paper we present a novel approach to model the multi-dimensional combustion manifold. We approximate the combustion manifold using a neural network function approximator and use it to predict the temperature and composition of the reaction. We present a novel training procedure which is developed to generate a smooth output curve for temperature over the course of a reaction. We then evaluate our work against the current approach of tabulation with linear interpolation in combustion simulations. We also provide an ablation study of our training procedure in the context of over-fitting in our model. The combustion dataset used for the modeling of combustion of H\\(_2\\) and O\\(_2\\) in this work is released alongside this paper.
更多查看译文
关键词
Deep learning, Combustion manifold modelling, Flamelet models
AI 理解论文
溯源树
样例
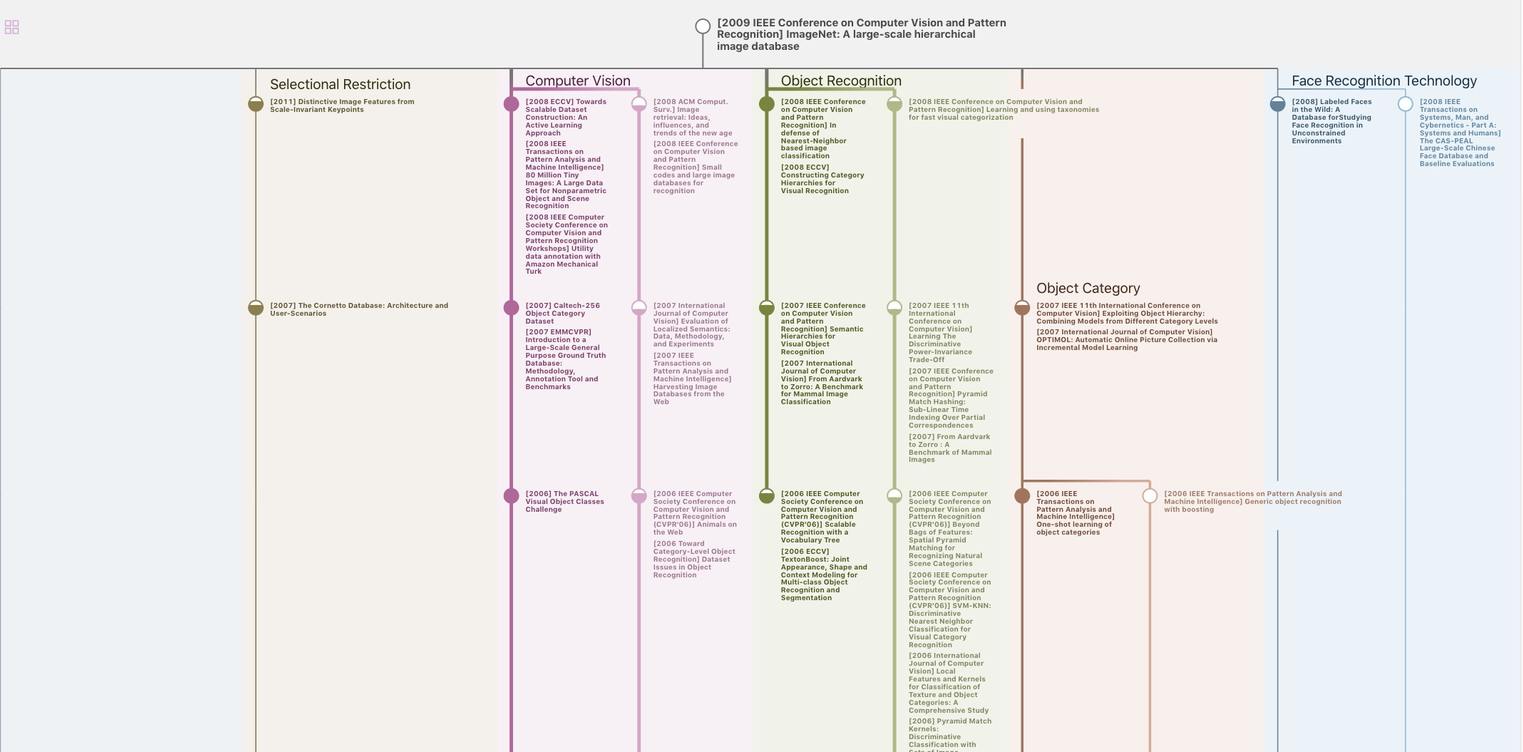
生成溯源树,研究论文发展脉络
Chat Paper
正在生成论文摘要