Improving Facial Emotion Recognition Systems With Crucial Feature Extractors
IMAGE ANALYSIS AND PROCESSING - ICIAP 2019, PT I(2019)
摘要
In this work, we have proposed enhancements that improve the performance of state-of-the-art facial emotion recognition (FER) systems. We believe that the changes in the positions of the fiducial points and the intensities capture the crucial information regarding the emotion of a face image. We propose the inputting of the gradient and the Laplacian of the input image together with the original into a convolutional neural network (CNN). These modifications help the network learn additional information from the gradient and Laplacian of the images. However, as shown by our results, the CNN in the existing state-of-the-art models is not able to extract this information from the raw images. In addition, we employ spatial transformer network to add robustness to the system against rotation and scaling. We have performed a number of experiments on two well known datasets, namely KDEF and FERplus. Our approach enhances the already high performance of the state-of-theart FER systems by 3 to 5%.In another contribution, we have proposed an efficient architecture that performs better than the state-of-the-art system on FERplus dataset, with the number of parameters reduced by a factor of about 24. Here also, the fusion of gradient or Laplacian image with the original image improves the recognition performance of the proposed model.
更多查看译文
关键词
Laplacian, Gradient, Convolutional neural network, Facial emotion recognition
AI 理解论文
溯源树
样例
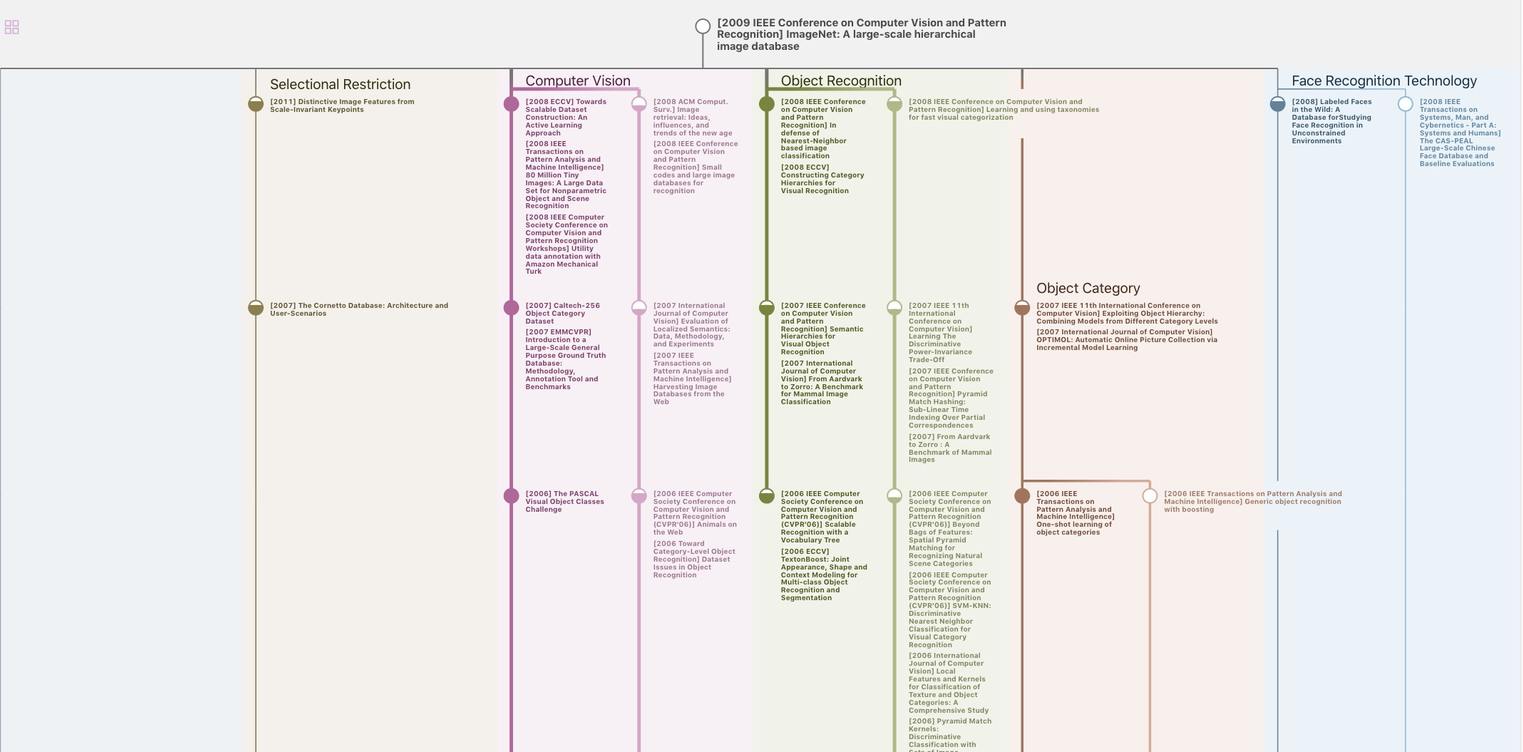
生成溯源树,研究论文发展脉络
Chat Paper
正在生成论文摘要