Can driving patterns predict identity and gender?
JOURNAL OF AMBIENT INTELLIGENCE AND HUMANIZED COMPUTING(2019)
摘要
The advances in vehicle equipment technology enabled us easy and large-scale collection of high-volume vehicle driving data. This data is an important resource for urban area traffic management and vehicle driving support system applications. It has privacy aspects as well. In this study, we are interested in whether machine learning techniques are a real threat to driver re-identification from published CAN (Controller Area Network) bus driving data. To understand, on Uyanik dataset (Takeda et al. in IEEE Trans Intell Transp Syst 12:1609–1623, 2011), we develop machine learning models for driver gender and identity prediction, after a multi step data preprocessing methods of sampling, feature extraction, feature elimination and discretization. Best gender prediction classifiers reached up to 0.97 accuracy rate; and best driver identity prediction classifiers reached up to 0.1 accuracy rate for 105-class and 0.98 accuracy rate for 2-class driver identification tasks. Those high accuracy results, even on a single dataset, suggest that driving patters may indeed act as quasi-identifiers, and hence they should be treated as sensitive personal data. As a result, dissemination of driving data should be done according to non-trivial data privacy protection procedures.
更多查看译文
关键词
Vehicle CAN bus, Machine learning, Privacy, Anonymity, Driver identification, Gender
AI 理解论文
溯源树
样例
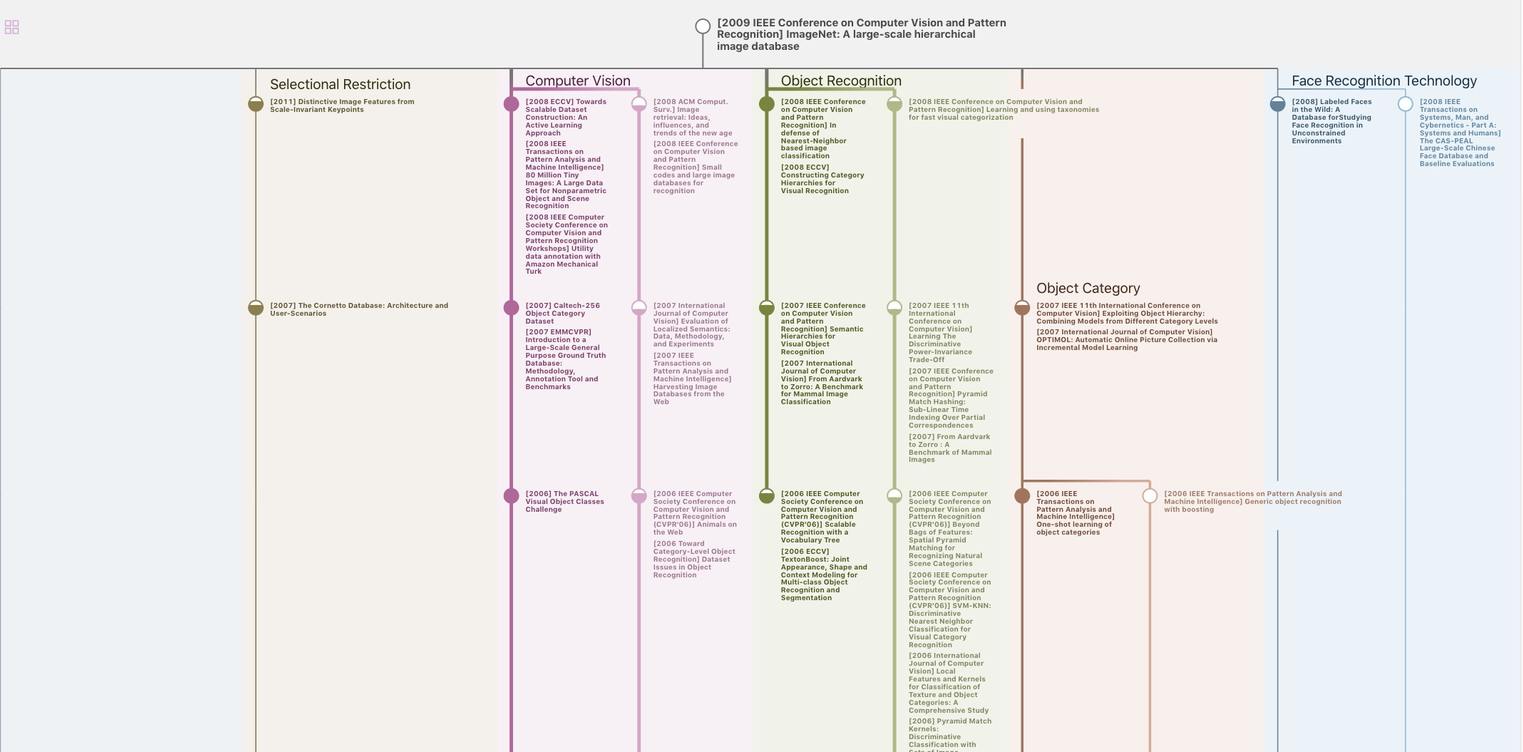
生成溯源树,研究论文发展脉络
Chat Paper
正在生成论文摘要