Evaluating Deep Convolutional Neural Networks As Texture Feature Extractors
IMAGE ANALYSIS AND PROCESSING - ICIAP 2019, PT II(2019)
摘要
Texture is an important visual property which has been largely employed for image characterization. Recently, Convolutional Networks has been the predominant approach on Computer Vision, and their application on texture analysis shows interesting results. However, their popularity steers around object recognition, and several convolutional architectures have been proposed and trained for this task. Therefore, this works evaluates 17 of the most diffused Deep Convolutional Neural Networks when employed for texture analysis as feature extractors. Image descriptors are obtained through Global Average Pooling over the output of the last convolutional layer of networks with random weights or learned from the ImageNet dataset. The analysis is performed under 6 texture datasets and using 3 different supervised classifiers (KNN, LDA, and SVM). Results using networks with random weights indicates that the architecture alone plays an important role in texture characterization, and it can even provide useful features for classification for some datasets. On the other hand, we found that although ImageNet weights usually provide the best results it can also perform similar to random weights in some cases, indicating that transferring convolutional weights learned on ImageNet may not always be appropriate for texture analysis. When comparing the best models, our results corroborate that DenseNet presents the highest overall performance while keeping a significantly small number of hyperparameters, thus we recommend its use for texture characterization.
更多查看译文
关键词
Deep Convolutional Neural Networks, Texture analysis, Feature extraction
AI 理解论文
溯源树
样例
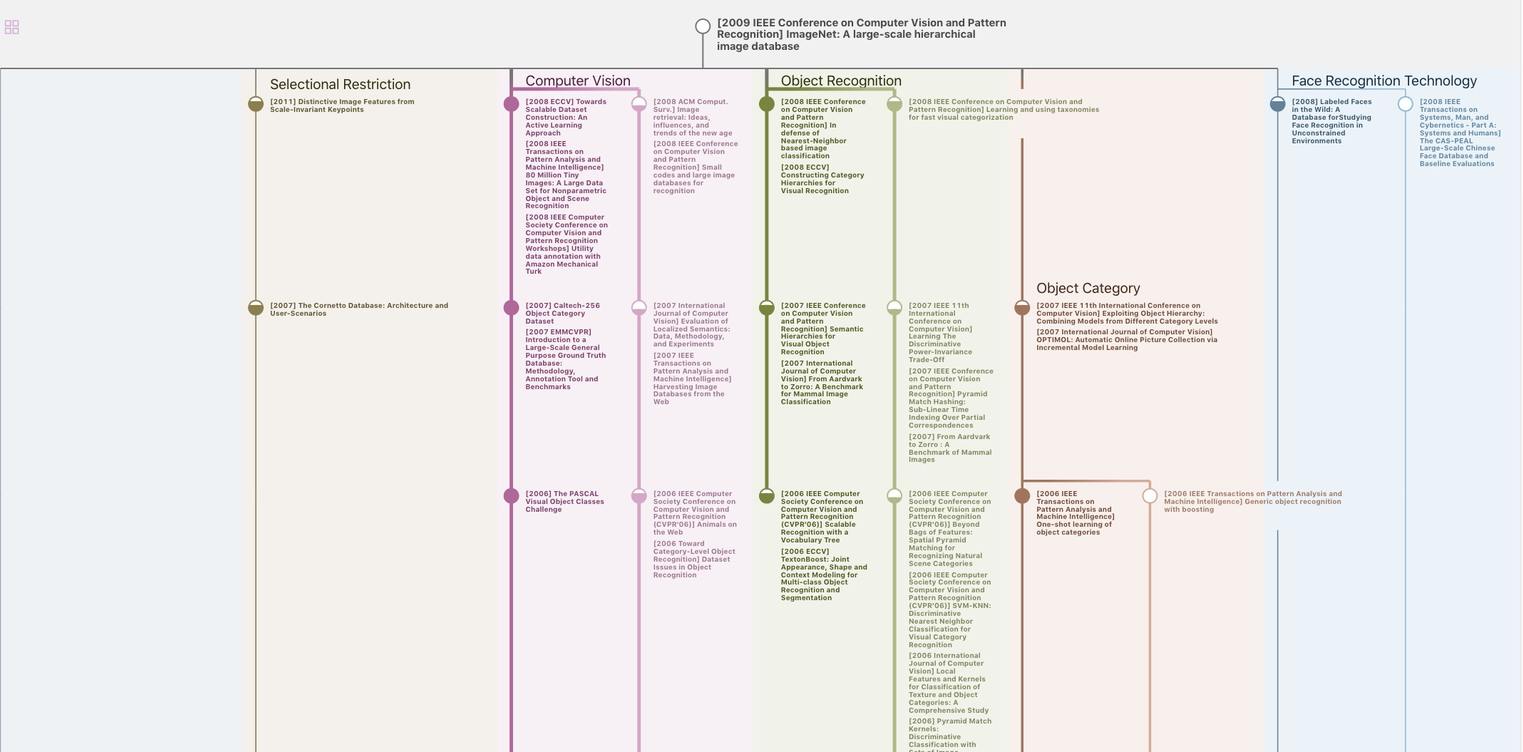
生成溯源树,研究论文发展脉络
Chat Paper
正在生成论文摘要