CoEvil: A Co-Evolutionary Model for Crime Inference Based on Fuzzy Rough Feature Selection
IEEE Transactions on Fuzzy Systems(2020)
摘要
Millions of crimes arise each year, which threatens public safety and harms the victims. Precise crime inference is of great significance in preventing crimes. The sharply increasing large-scale heterogeneous data provide a chance to reveal the patterns and trends in crimes. Several approaches employing feature-based regression or spatiotemporal distribution fitting are proposed but lack of some considerations: 1) ignore the dynamic mutual influences among crimes and locations; and 2) overlook the large scale, incompleteness, uncertainty, and vagueness in the heterogeneous data. This article comprehensively investigates the reliability and applicability of proposing a coevolutionary model to formulate the interaction pattern among the crimes and locations and develops a fuzzy-rough-set-based feature selection method to discover the distinctiveness and permanence properties of the crimes and locations with different latent features. Extensive experiments show that our algorithm achieves the mean absolute error of 1.529 (hour) in the crime time inference and the accuracy of 0.653 and 0.633 in the crime type and location inference, which surpass the state of the arts more than 6.5, 1.9, and 1.8 times, respectively. Additional experiments on different parameter settings of our model are provided to further explore its effectiveness and scalability.
更多查看译文
关键词
Feature extraction,Road transportation,Safety,Rough sets,Proposals,Market research,Reliability
AI 理解论文
溯源树
样例
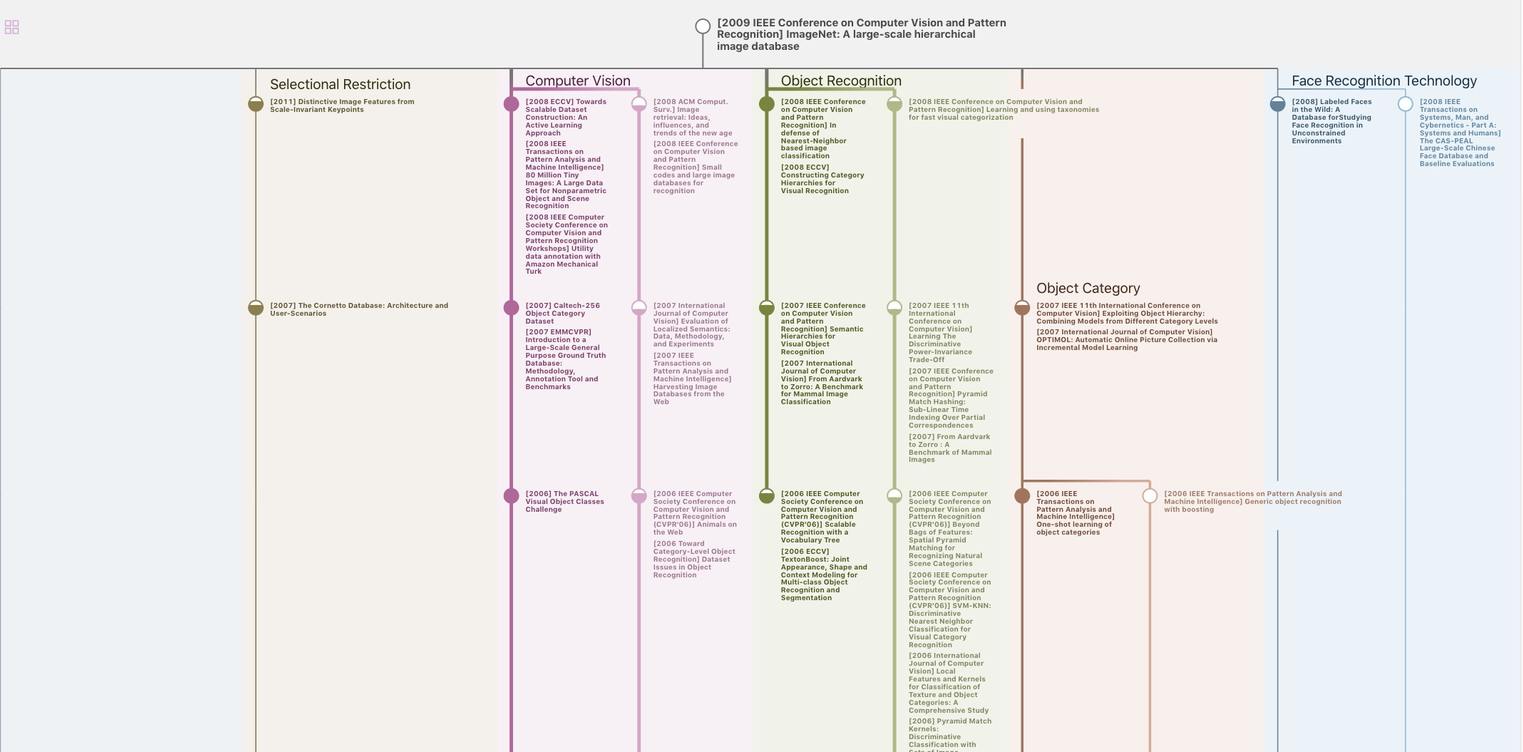
生成溯源树,研究论文发展脉络
Chat Paper
正在生成论文摘要