Longitudinal brain tumor segmentation prediction in MRI using feature and label fusion
Biomedical Signal Processing and Control(2020)
摘要
This work proposes a novel framework for brain tumor segmentation prediction in longitudinal multimodal MRI scans, comprising two methods; feature fusion and joint label fusion (JLF). The first method fuses stochastic multi-resolution texture features with tumor cell density feature to obtain tumor segmentation predictions in follow-up timepoints using data from baseline pre-operative timepoint. The cell density feature is obtained by solving the 3D reaction-diffusion equation for biophysical tumor growth modelling using the Lattice-Boltzmann method. The second method utilizes JLF to combine segmentation labels obtained from (i) the stochastic texture feature-based and Random Forest (RF)-based tumor segmentation method; and (ii) another state-of-the-art tumor growth and segmentation method, known as boosted Glioma Image Segmentation and Registration (GLISTRboost, or GB). We quantitatively evaluate both proposed methods using the Dice Similarity Coefficient (DSC) in longitudinal scans of 9 patients from the public BraTS 2015 multi-institutional dataset. The evaluation results for the feature-based fusion method show improved tumor segmentation prediction for the whole tumor(DSCWT = 0.314, p = 0.1502), tumor core (DSCTC = 0.332, p = 0.0002), and enhancing tumor (DSCET = 0.448, p = 0.0002) regions. The feature-based fusion shows some improvement on tumor prediction of longitudinal brain tumor tracking, whereas the JLF offers statistically significant improvement on the actual segmentation of WT and ET (DSCWT = 0.85 ± 0.055, DSCET = 0.837 ± 0.074), and also improves the results of GB. The novelty of this work is two-fold: (a) exploit tumor cell density as a feature to predict brain tumor segmentation, using a stochastic multi-resolution RF-based method, and (b) improve the performance of another successful tumor segmentation method, GB, by fusing with the RF-based segmentation labels.
更多查看译文
关键词
Tumor segmentation prediction,Longitudinal MRI,Feature and label fusion,Reaction-diffusion equation,Lattice-Boltzmann method,Texture features,BraTS
AI 理解论文
溯源树
样例
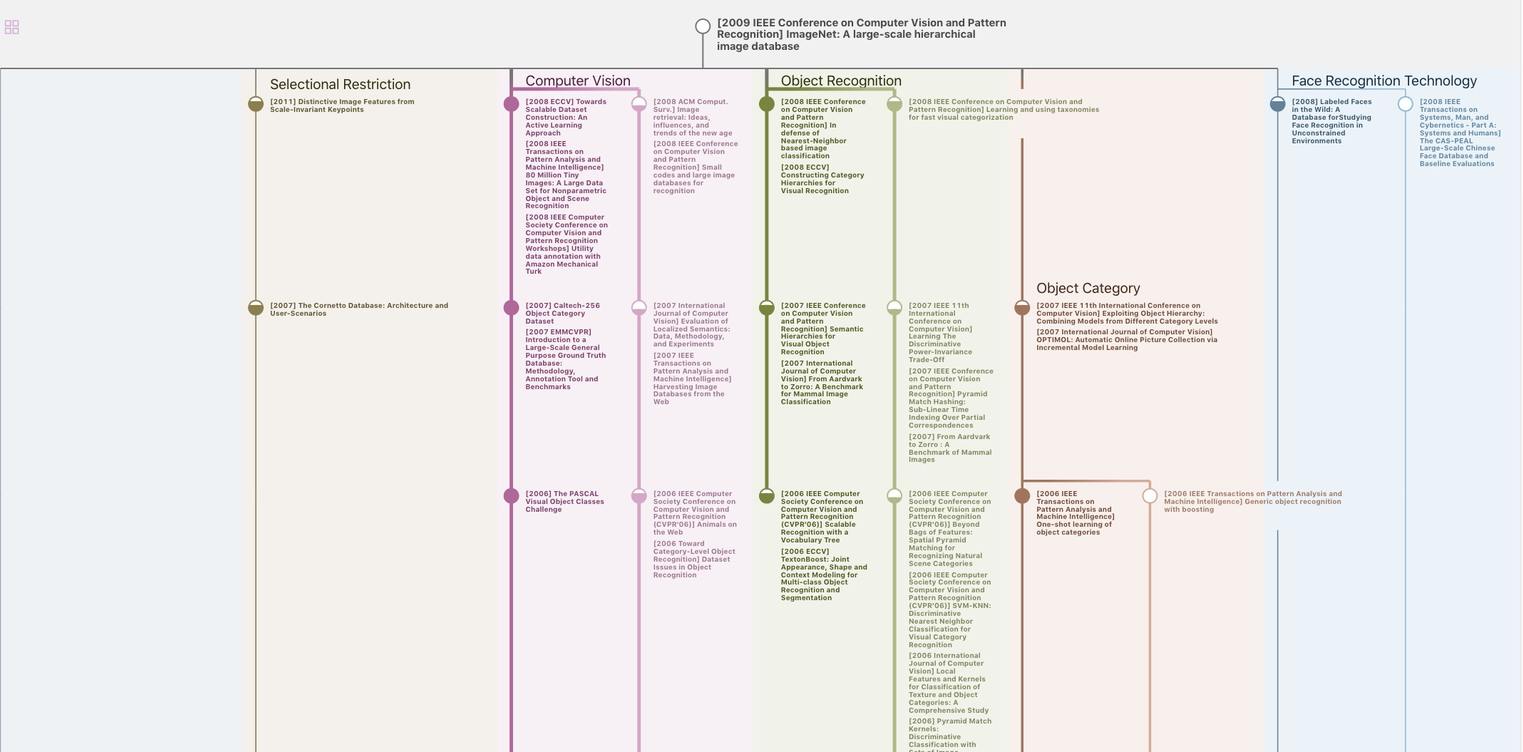
生成溯源树,研究论文发展脉络
Chat Paper
正在生成论文摘要