Towards a Fault-Tolerant Speaker Verification System: A Regularization Approach to Reduce the Condition Number
INTERSPEECH(2019)
摘要
Large-scale deployment of speech interaction devices makes it possible to harvest tremendous data quickly, which also introduces the problem of wrong labeling during data mining. Mislabeled training data has a substantial negative effect on the performance of speaker verification system. This study aims to enhance the generalization ability and robustness of the model when the training data is contaminated by wrong labels. Several regularization approaches are proposed to reduce the condition number of the speaker verification problem, making the model less sensitive to errors in the inputs. They are validated on both NIST SRE corpus and far-field smart speaker data. The results suggest that the performance deterioration caused by mislabeled training data can be significantly ameliorated by proper regularization.
更多查看译文
关键词
Speaker Verification, Mislabeled Data, Entropy Minimization, Loss Regularization, Co-Training, Condition Number
AI 理解论文
溯源树
样例
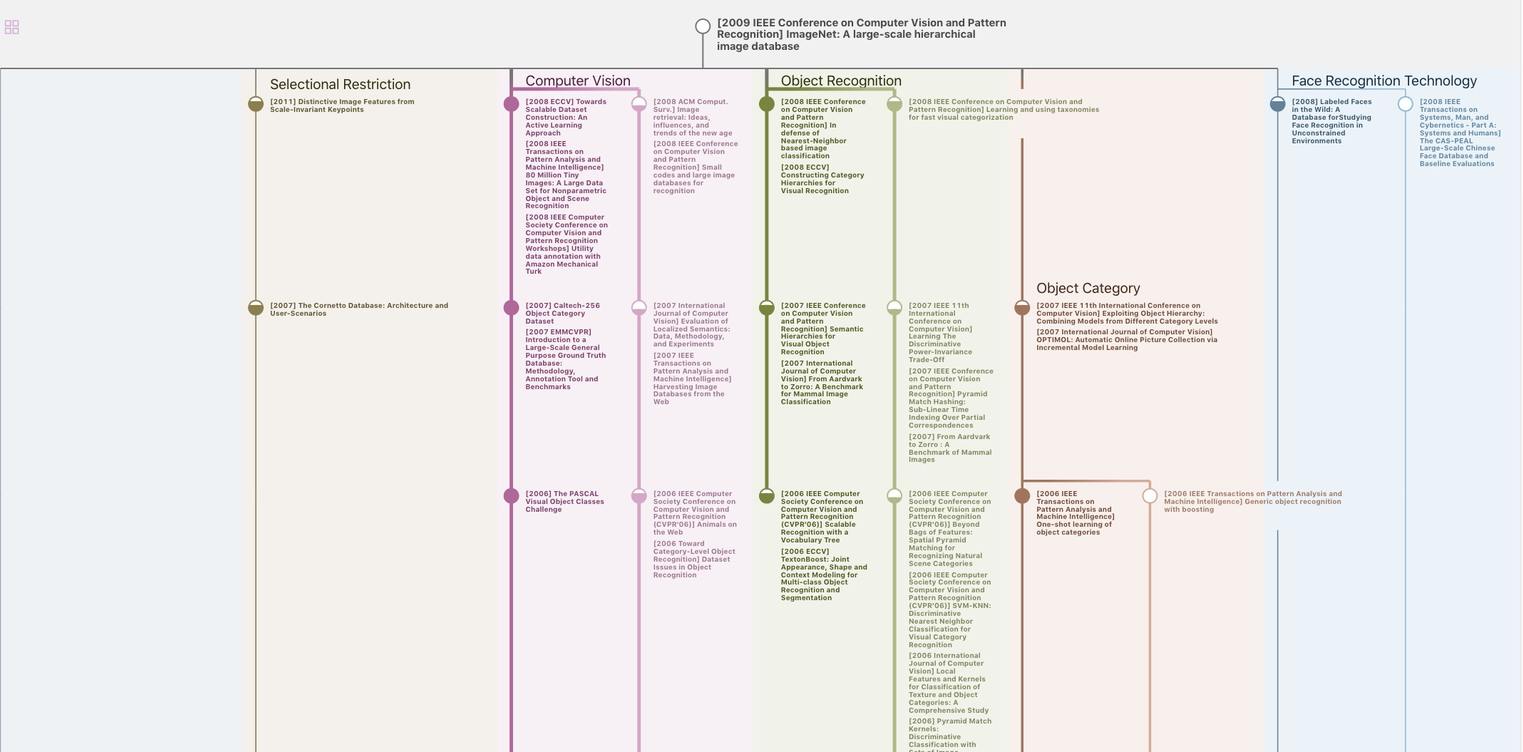
生成溯源树,研究论文发展脉络
Chat Paper
正在生成论文摘要