Predicting Planning Delays Using Machine Learning of Radiation Therapy Workflow Data from a Record and Verify System
International journal of radiation oncology, biology, physics(2019)
摘要
To develop a machine learning model based on radiation therapy (RT) workflow data extracted from a record and verify (RV) system to classify and predict radiotherapy plans that could lead to potential patient delays downstream in the external beam planning process. Task assignment tickets, dose delivery history, beam configuration, and plan demographics (i.e. site, technique) were extracted from the RV database resulting in a total initial data set consisting of 75741 records each representing a portion of a treatment plan. Following cross-linking of data sets and filtering for only external beam plans, all data items were compiled into 6724 records of individual treatments that were planned and delivered over a 12-month period. These records were then used as a training set with six different machine learning algorithms to develop a model capable of identifying the following ground-truth classes: 1) rushed plans ( defined as planning completed a day or less before treatment commences) and; 2) exceptional plans (defined as taking longer than a standard 3 business days to complete). Model validation was performed using 10 passes through the training data, each in turn using 10-fold cross validation to split the dataset into training and testing data. The AUC (area under the curve), overall accuracy, and Kappa statistic were calculated for each iteration of the models. The average value for each statistic was collected and used as the final output for each classifier to be compared. Splitting the data set into trivial events (aka plans that did not require prediction) and applying a Random Forest algorithm to the non-trivial events gave the best performance for both classes. The machine learning element handled planning events requiring prediction and achieved an AUC (area under the curve) of 0.83 for the rush class (n=5870) and 0.89 for the exception class (n=5438). The total combination of both trivial and non-trivial classes gave an AUC of 0.86 for the rush class and 0.92 for the exception class giving excellent discriminatory power for both categories. Overall prediction accuracy and Kappa statistics were, respectively, 78% and 0.54 for the rush class and 85% and 0.67 for the exception class. We have developed a machine learning model that has the potential to predict issues that could occur downstream in the planning process. The resulting model can be used in conjunction with real-time workflow data and scheduling algorithms to aid RT managers in optimizing task assignments and reducing the potential for sub-optimal plans and/or patient delays.
更多查看译文
AI 理解论文
溯源树
样例
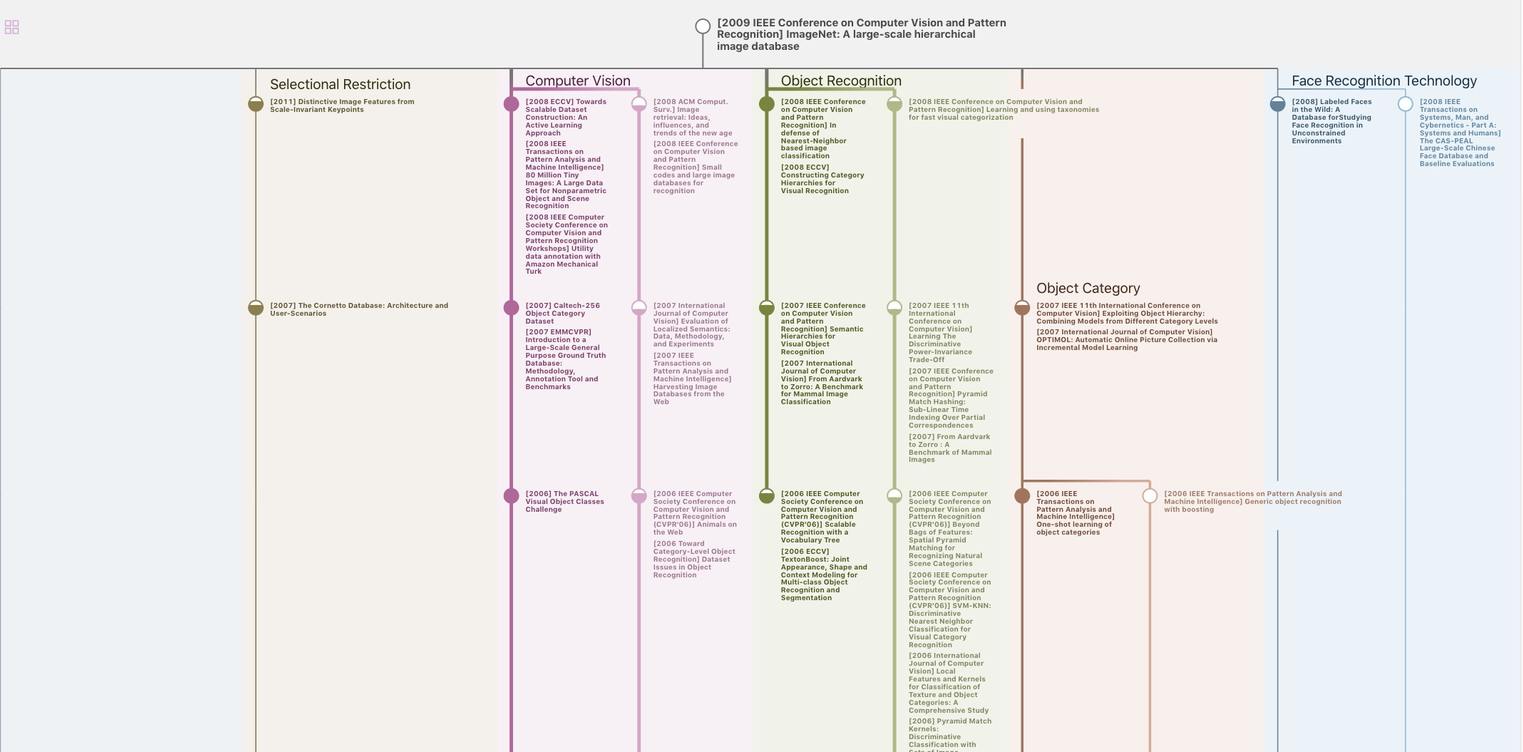
生成溯源树,研究论文发展脉络
Chat Paper
正在生成论文摘要