Predicting the Local Control of Brain Metastatic Tumor after Gamma Knife Radiosurgery from the Treatment Planning Image of Tumor by a Convolution Neural Network Method
International journal of radiation oncology, biology, physics(2019)
摘要
It is known that the heterogeneity or ring enhancement observed in the Gadolinium (Gd) enhanced T1-weighted MR image of tumor is associated with poor local control (LC) and survival. In the current study, we compared the accuracy of a machine learning technique using radiomics features and a visual evaluation method for predicting the LC of brain metastases (BMs) treated by stereotactic radiosurgery (SRS). Data of 115 tumors of 45 patients with BMs, who underwent GKRS at the University of Minnesota from 2006 to 2012 were analyzed. The treatment outcomes of these tumors were known prior to the study. The data were split into two sets: 80 tumors for training of models and 35 tumors for model testing. The LC was classified into two groups by using the RECIST definition. The first group (group I) was a Complete Response (CR) + Partial Response (PR). The second group (group II) was Stable Disease (SD) + Progressive Disease (PD). A total number of 705 radiomics features were extracted from radiotherapy planning MRI scans (Gd-contrast T1-weighted) by using an open infrastructure software program. An experienced radiation oncologist classified the tumors into three types of patterns (homogeneous, heterogeneous, or ring) by visually inspecting the MR images. Based on the well-accepted knowledge, we assigned the predicted LCs of the homogeneous tumors and tumors with heterogeneous or ring enhancement to the success group (group I) and the failure group (group II), respectively. For the machine learning method, a prediction model was constructed by using a convolution neural network (NN) classifier coupled with a least absolute shrinkage and selection operator (LASSO). We trained the model (NN-LASSO) by using the training data set. The test data set was used to evaluate the prediction capability of the NN-LASSO model and the visual evaluation technique. We calculated the precision, accuracy, sensitivity by generating confusion matrices. Furthermore, the area under the receiver operating characteristic curve (AUC) was obtained for the NN-LASSO method. By the LASSO analysis of the training data, we found seven radiomics features (45-7ClusterShade, 225-7ClusterShade, 45-7InformationMeasureCorr1, 225-7InformationMeasureCorr1, 90-4InformationMeasureCorr2, 225-7Energy and 315-5Energy) useful for the classification. The accuracy, specificity and sensitivity of the visual evaluation method were 44.0%, 54.3% and 23.7%. On the other hand, the accuracy, specificity and sensitivity of the NN-LASSO model were 82.8%, 80.0%, 90.2%, and the AUC was 0.78. The NN-LASSO model using the radiomics features of tumor image was more accurate than the visual evaluation method using the image heterogeneity information in predicting the LC of brain metastases after GKRS. Because of the good prediction ability of the method, the method can be used to assist physicians to have more accurate expectation of the treatment outcome than the traditional method.
更多查看译文
AI 理解论文
溯源树
样例
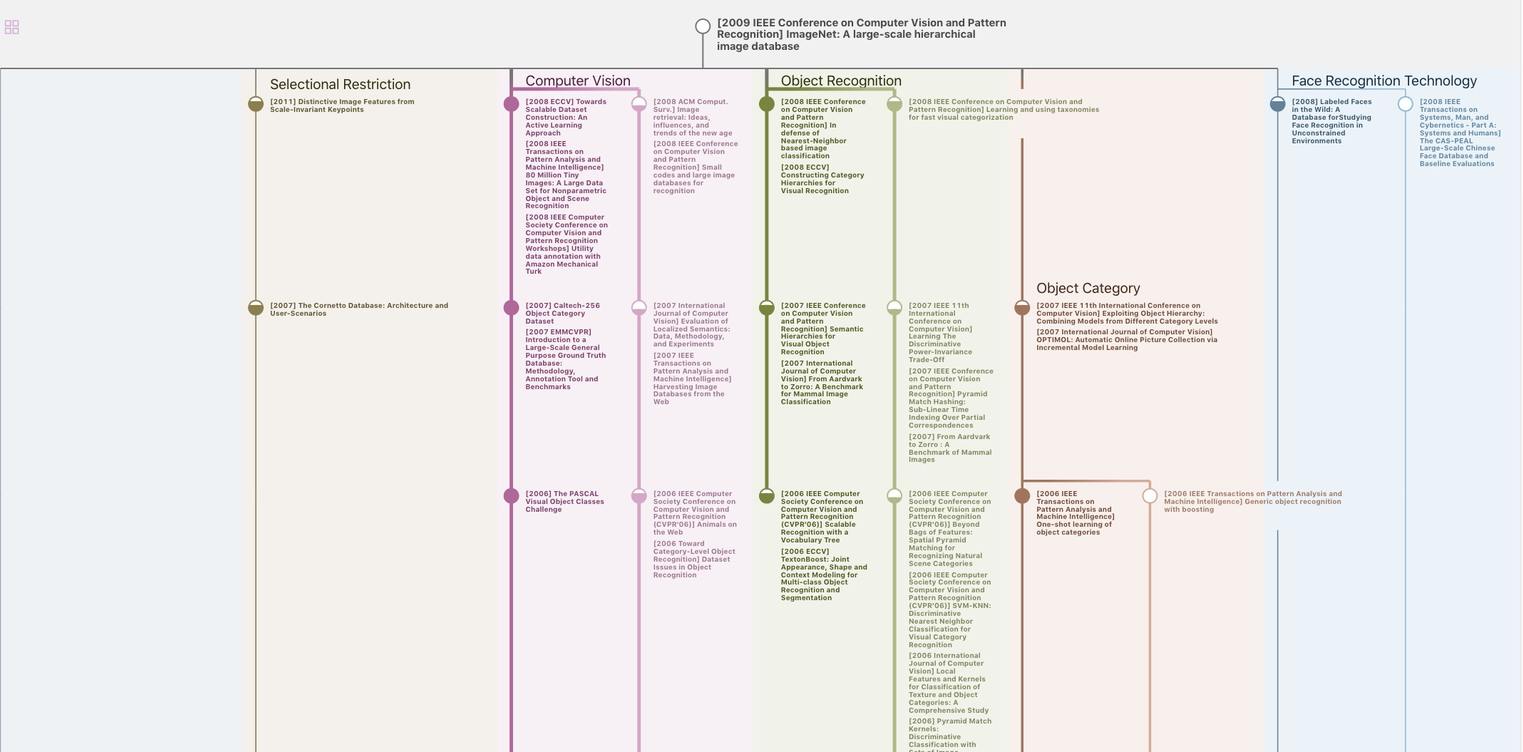
生成溯源树,研究论文发展脉络
Chat Paper
正在生成论文摘要