In-Silico Staining From Bright-Field And Fluorescent Images Using Deep Learning
ARTIFICIAL NEURAL NETWORKS AND MACHINE LEARNING - ICANN 2019: IMAGE PROCESSING, PT III(2019)
摘要
Fluorescent markers are commonly used to characterize single cells and to uncover molecular properties. Unfortunately, fluorescent staining is laborious and costly, it damages tissue and suffers from inconsistencies. Recently deep learning approaches have been successfully applied to predict fluorescent markers from bright-field images [ 1 – 3 ]. These approaches can save costs and time and speed up the classification of tissue properties. However, it is currently not clear how different image channels can be meaningfully combined to improve prediction accuracy. Thus, we investigated the benefits of multi channel input for predicting a specific transcription factor antibody staining. Our image dataset consists of three channels: bright-field, fluorescent GFP reporter and transcription factor antibody staining. Fluorescent GFP is constantly expressed in the genetically modified cells from a particular differentiation step onwards. The cells are additionally stained with a specific transcription factor antibody that marks a subtype of GFP positive cells. For data acquisition we used a Leica SP8 and a Zeiss LSM780 microscope with 20x objectives. We trained a deep neural network, a modified U-Net [ 4 ], to predict the transcription factor antibody staining from bright-field and GFP channels. To this end, we trained on 2432 three-dimensional images containing roughly 7600 single cells and compared the accuracy for prediction of the transcription factor antibody staining using bright-field only, GFP only, and both channels together on a test-set of 576 images with approximately 1800 single cells. The same training- and test-set was used for all experiments (Fig. 1 ). The prediction error, measured as the mean relative pixel-wise error over the test-set, was calculated to 61% for prediction from bright-field, 55% for prediction from GFP and 51% for prediction both bright-field and GFP images. The median pixel-wise Pearson correlation coefficient, increases from 0.12 for prediction from bright-field channels to 0.17 for prediction from GFP channels, to 0.31 for prediction from bright-field and GFP channels (Fig. 2 ). Our work demonstrates that prediction performance can be increased by combining multiple channels for in-silico prediction of stainings. We anticipate this research to be a starting point for further investigations on which stainings could be predicted from other stainings using deep learning. These approaches bear a huge potential in saving laborious and costly work for researchers and clinical technicians and could reveal biological relationships between fluorescent markers.
更多查看译文
关键词
Staining prediction, Multiple input deep learning, Image processing
AI 理解论文
溯源树
样例
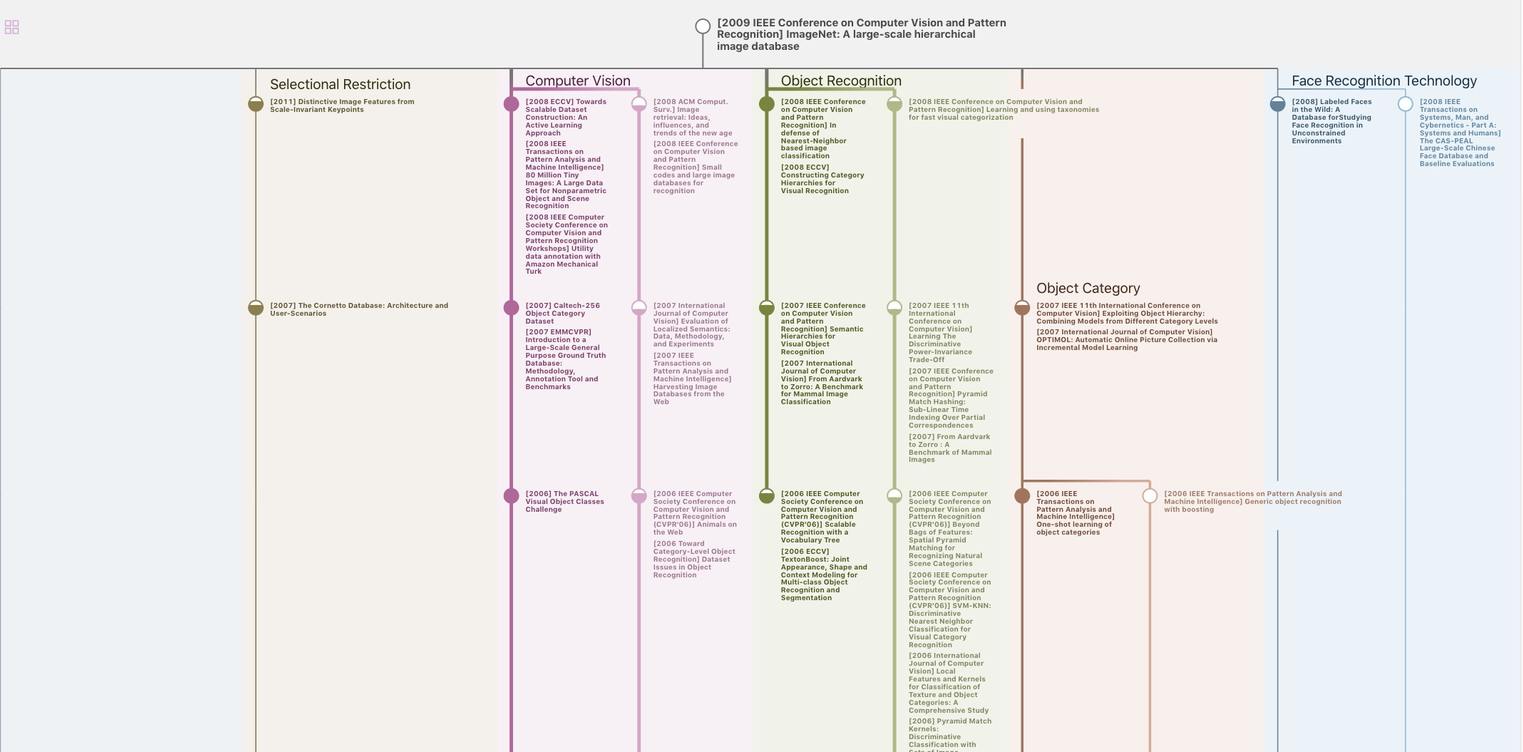
生成溯源树,研究论文发展脉络
Chat Paper
正在生成论文摘要