Adaptive Event-Based Reinforcement Learning Control
PROCEEDINGS OF THE 2019 31ST CHINESE CONTROL AND DECISION CONFERENCE (CCDC 2019)(2019)
摘要
Reinforcement learning (RL) methods have been successfully used to deal with the control and/or decision making problem of many engineering domains, such as industrial manufacturing, power management, industrial robot and rehabilitation robotic system, etc. However, the state-based method comes into difficulty when solving the control and/or decision making of high dimensional systems due to the computation loads and storage requirements. In addition, to acquire better control results, the state based RL method often requests as many states as possible to be exploited and explored, thus this method, in effect, does not suit to solve the control problems of those unknown and/or partial known systems. To solve those problems, a new adaptive event-based reinforcement learning algorithm (ETRL) is proposed in the paper. In the proposed ETRL approach, an event generator is employed firstly to sample a set of states (i.e., event state, shortened to ES in the paper) by effective event sampling strategies from the unknown system state space. Then Q learning controller uses the ES and ES-based reinforcement signal (i.e., reward feedback) to guide and adjust the control law. Moreover, an adaptive weighted nearest neighbor and sample reuse method (WNNSR) to sample the most sensitive actions is proposed to guarantee both control performance and stability of the proposed ETRL during the process of learning. Finally, convergence analysis verifies the proposed ETRL approach.
更多查看译文
关键词
Reinforcement Learning, Event State, ETRL, WNNSR, Sample Reuse
AI 理解论文
溯源树
样例
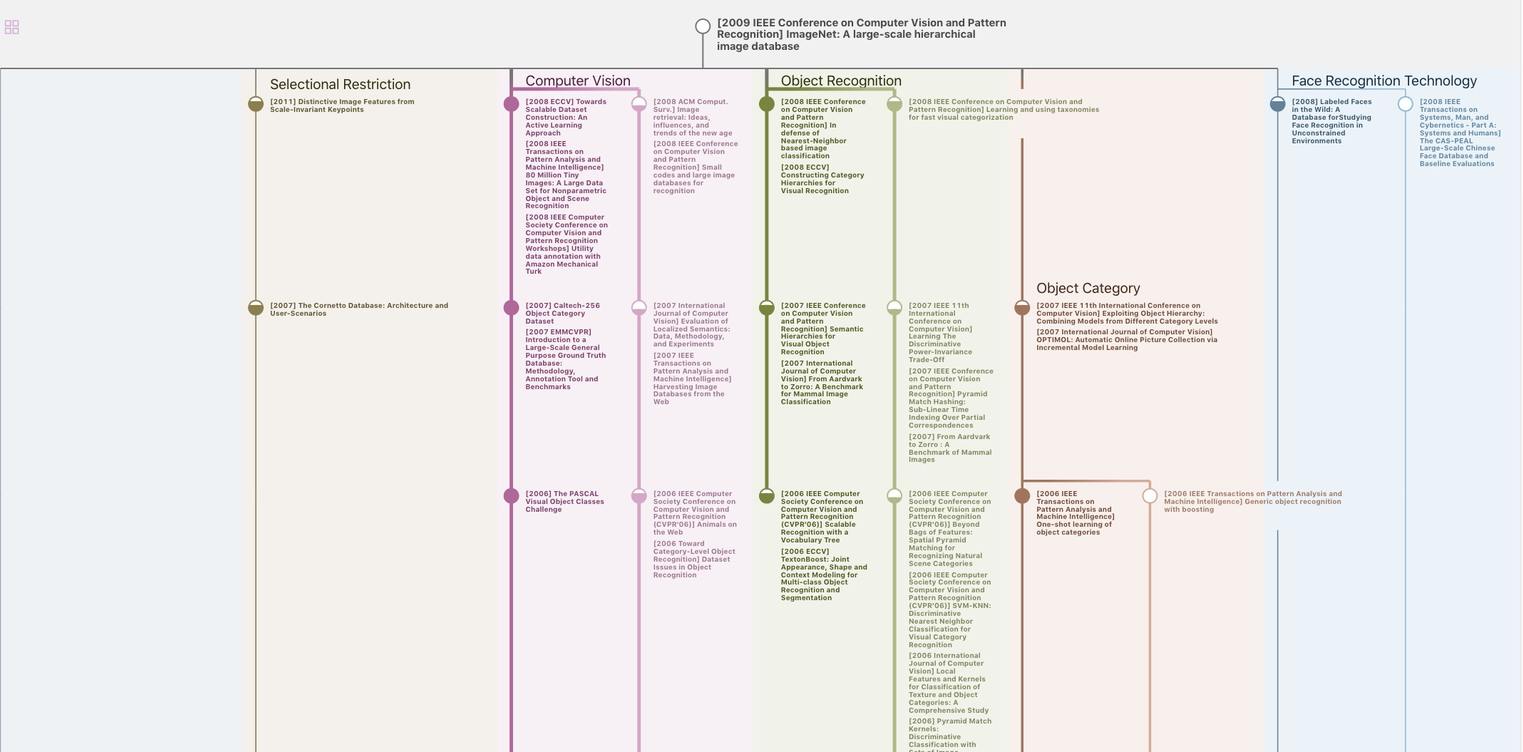
生成溯源树,研究论文发展脉络
Chat Paper
正在生成论文摘要