Development of Robust Automated Scoring Models Using Adversarial Input for Oral Proficiency Assessment
INTERSPEECH(2019)
摘要
In this study, we developed an automated scoring model for an oral proficiency test eliciting spontaneous speech from non-native speakers of English. In a large-scale oral proficiency test, a small number of responses may have atypical characteristics that make it difficult even for state-of-the-art automated scoring models to assign fair scores. The oral proficiency test in this study consisted of questions asking about content in materials provided to the test takers, and the atypical responses frequently had serious content abnormalities. In order to develop an automated scoring system that is robust to these atypical responses, we first developed a set of content features to capture content abnormalities. Next, we trained scoring models using the augmented training dataset, including synthetic atypical responses. Compared to the baseline scoring model, the new model showed comparable performance in scoring normal responses, while it assigned fairer scores for authentic atypical responses extracted from operational test administrations.
更多查看译文
关键词
automated speech scoring, content scoring, speech recognition, non-native speech, adversarial input
AI 理解论文
溯源树
样例
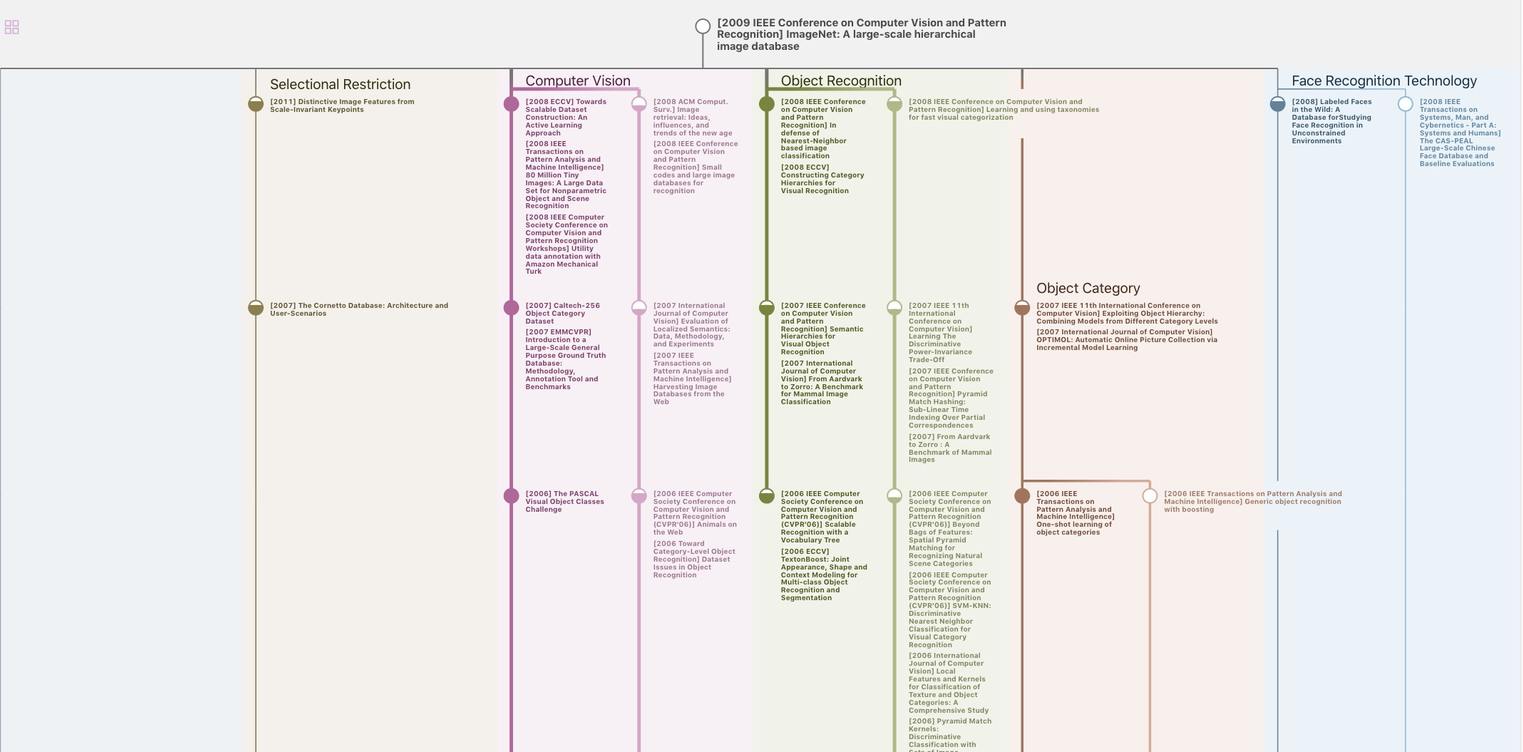
生成溯源树,研究论文发展脉络
Chat Paper
正在生成论文摘要