Unsupervised Low-Rank Representations for Speech Emotion Recognition
INTERSPEECH(2019)
摘要
We examine the use of linear and non-linear dimensionality reduction algorithms for extracting low-rank feature representations for speech emotion recognition. Two feature sets are used, one based on low-level descriptors and their aggregations (IS10) and one modeling recurrence dynamics of speech (RQA), as well as their fusion. We report speech emotion recognition (SER) results for learned representations on two databases using different classification methods. Classification with low-dimensional representations yields performance improvement in a variety of settings. This indicates that dimensionality reduction is an effective way to combat the curse of dimensionality for SER. Visualization of features in two dimensions provides insight into discriminatory abilities of reduced feature sets.
更多查看译文
关键词
Non-linear, dimensionality reduction, emotion recognition, speech, manifold learning, autoencoder
AI 理解论文
溯源树
样例
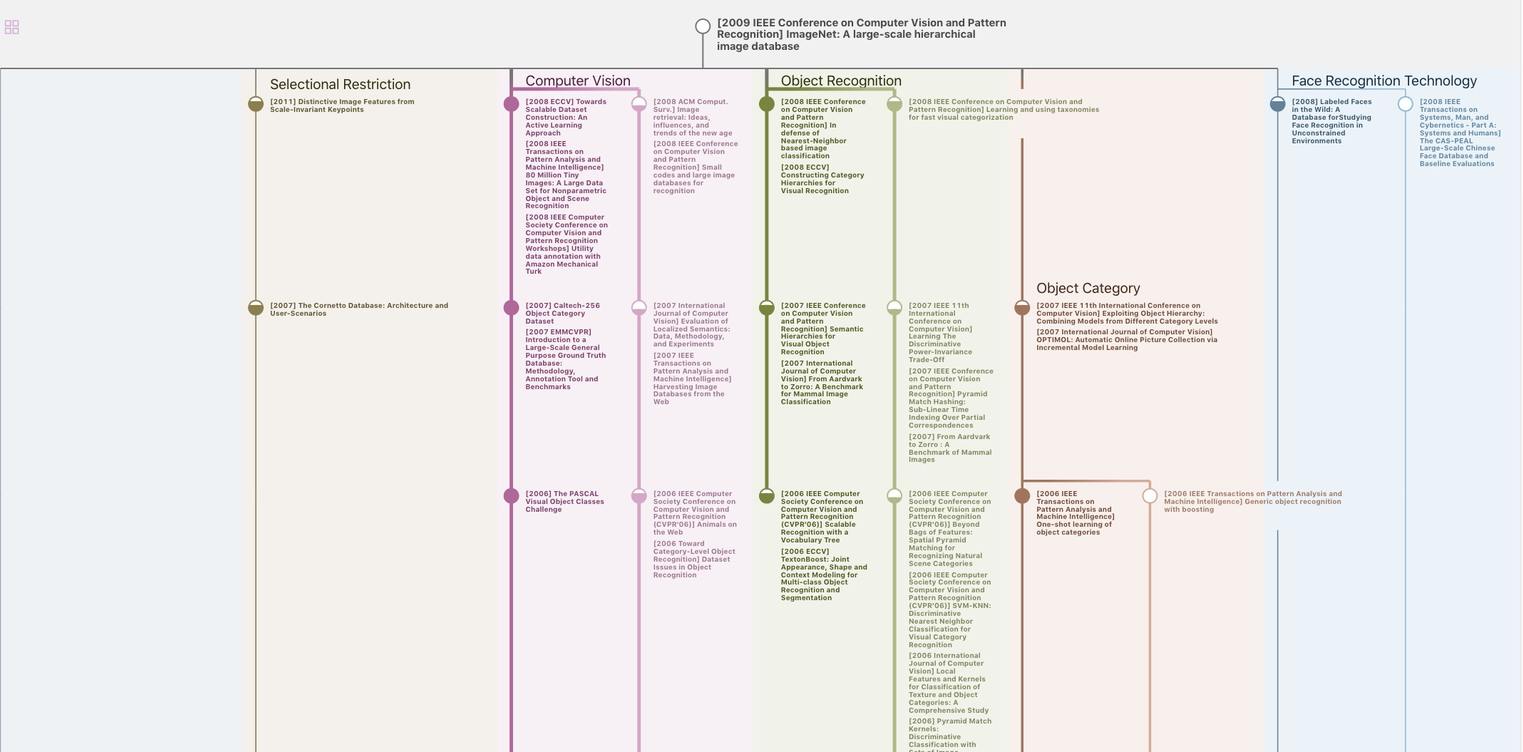
生成溯源树,研究论文发展脉络
Chat Paper
正在生成论文摘要