Analysis of Deep Learning Architectures for Cross-Corpus Speech Emotion Recognition
INTERSPEECH(2019)
摘要
Speech Emotion Recognition (SER) is an important and challenging task for human-computer interaction. In the literature deep learning architectures have been shown to yield state-of-the-art performance on this task when the model is trained and evaluated on the same corpus. However, prior work has indicated that such systems often yield poor performance on unseen data. To improve the generalisation capabilities of emotion recognition systems one possible approach is cross-corpus training, which consists of training the model on an aggregation of different corpora. In this paper we present an analysis of the generalisation capability of deep learning models using cross-corpus training with six different speech emotion corpora. We evaluate the models on an unseen corpus and analyse the learned representations using the t-SNE algorithm, showing that architectures based on recurrent neural networks are prone to overfit the corpora present in the training set, while architectures based on convolutional neural networks (CNNs) show better generalisation capabilities. These findings indicate that (1) cross-corpus training is a promising approach for improving generalisation and (2) CNNs should be the architecture of choice for this approach.
更多查看译文
关键词
speech emotion recognition, neural networks
AI 理解论文
溯源树
样例
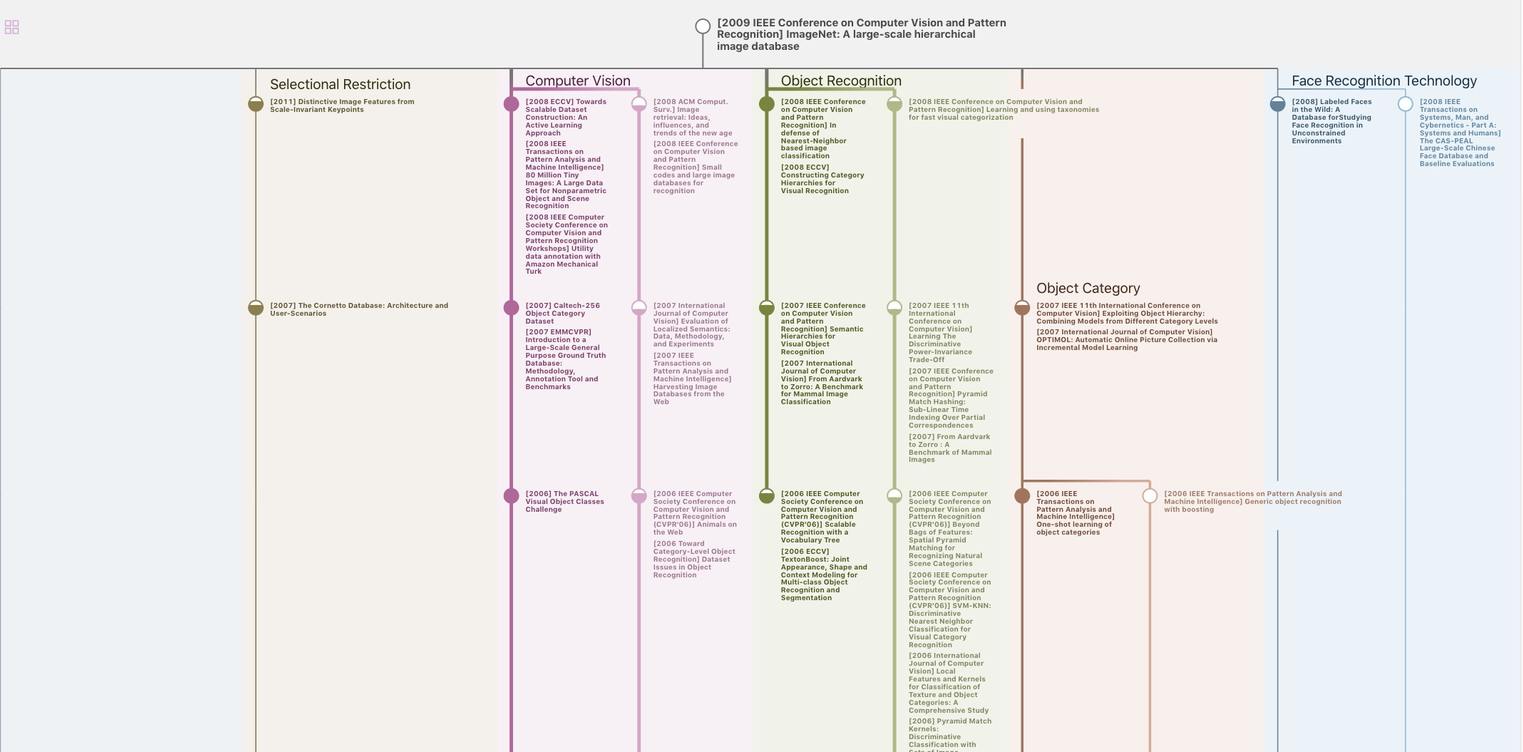
生成溯源树,研究论文发展脉络
Chat Paper
正在生成论文摘要