Symmetrical Adversarial Training Network: A Novel Model For Text Generation
ARTIFICIAL NEURAL NETWORKS AND MACHINE LEARNING - ICANN 2019: TEXT AND TIME SERIES, PT IV(2019)
摘要
Text generation has always been the core issue in the field of natural language processing. Over the past decades, Generative Adversarial network (GAN) has proven its great potential in generating realistic synthetic data, performing competitively in various domains like computer vision. However, the characteristics of text discretization limit the application of GANs in natural language processing. In this paper, we proposed a novel Symmetrical Adversarial Training Network (SATN) which employed symmetrical text comparison mechanism for the purpose of generating more realistic and coherent text samples. In the SATN, a Deep Attention Similarity Model (DASM) was designed to extract fine-grained original-synthetic sentence feature match loss for improving the performance of generative network. With DASM, the SATN can identify the difference between sentences in word level and pay attention to relevant meaningful words. Meanwhile, we utilize the DASM loss to compensate for the defect of the objective function in adversarial training. Our experiments demonstrated significant improvement in evaluation.
更多查看译文
关键词
Adversarial training, Attention mechanism, Symmetrical comparison
AI 理解论文
溯源树
样例
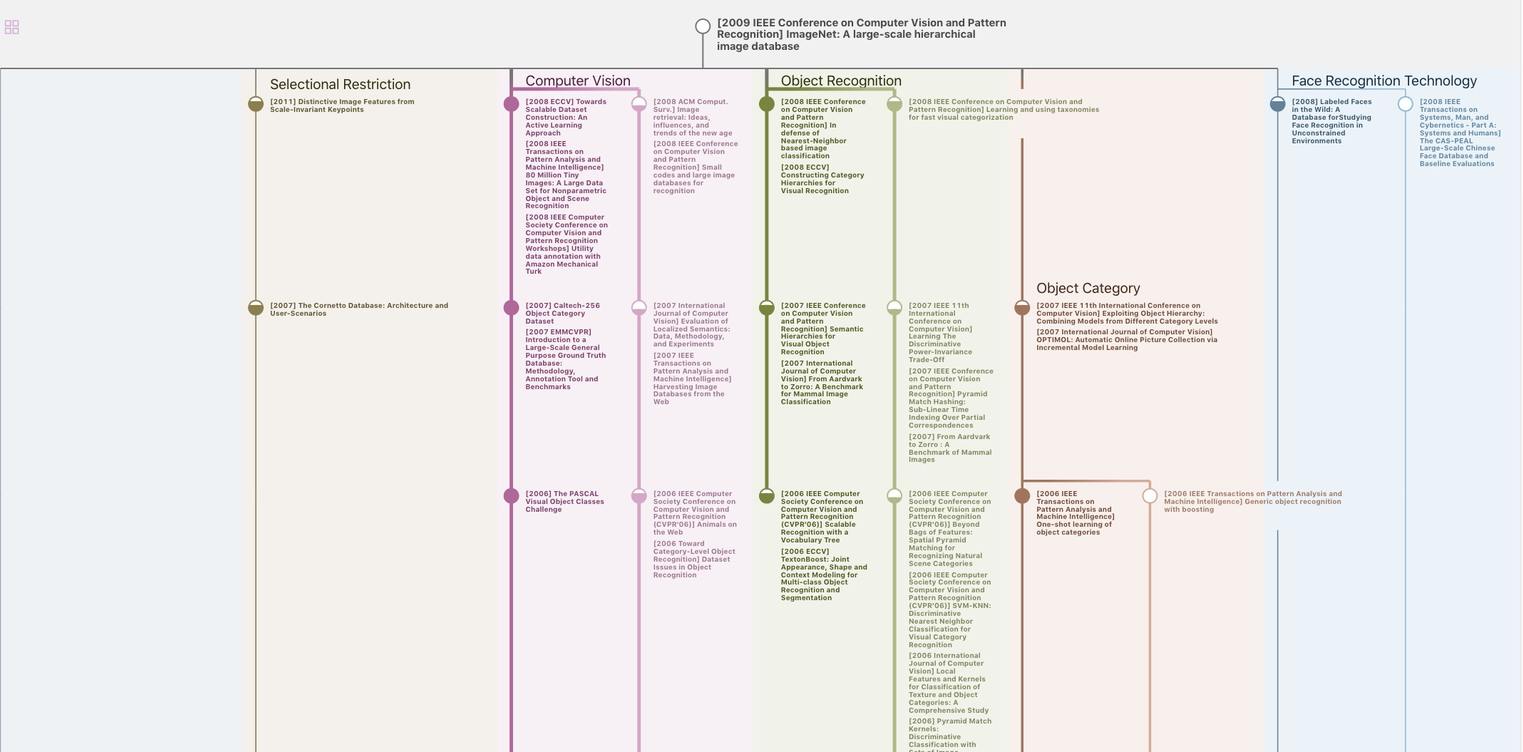
生成溯源树,研究论文发展脉络
Chat Paper
正在生成论文摘要