Imaging-driven Biophysical Model for the Differentiation of Tumor Progression from Radiation Necrosis
International journal of radiation oncology, biology, physics(2019)
摘要
This project validates the ability of a novel clinical imaging-driven biophysical model to predict the etiology of enhancing lesions following stereotactic radiosurgery (SRS) for brain metastasis (BM). These lesions present enormous clinical challenges as clinical symptoms and radiographic findings for radiation necrosis (RN) and tumor progression are often indistinguishable. We hypothesized that our model for differentiating RN from tumor progression could be validated in a large-scale retrospective cohort study. A prospectively maintained database at our institution with 73 patients with 78 BM treated with SRS and histologically confirmed RN or tumor progression were retrospectively assessed using our biophysical model. Briefly, a reaction-diffusion logistic growth model mechanically coupled to the surrounding tissue was used to extract tumor cell proliferation rate and diffusion coefficients based on fitting areas of post-contrast T1-weighted MR enhancement observed during serial imaging time points. The model was then used to calculate mass effect due to the mechanical stress field incurred during lesion expansion. The Dice similarity coefficient was used to quantify the similarity of the model-estimated stress field with the edema front visualized in FLAIR imaging. These metrics for prediction of tumor progression versus RN were evaluated using a receiver operating characteristic curve and compared to standard radiographic morphometric analysis including the change in the longest dimension of the lesion, change in the volume of the lesion, and FLAIR/T1 lesion quotient. Standard radiographic morphometric analysis of the serial post-contrast T1-weighted enhanced and FLAIR images reflected poor ability to differentiate between tumor progression and RN for the change in the longest dimension of the lesion (ROC AUC = 0.73, 95% CI: 0.61 – 0.85, p = 0.0009, 74% sensitivity and 63% specificity), change in lesion volume (ROC AUC 0.61, 95% CI: 0.47 – 0.75, p = 0.1262, 43% sensitivity and 64% specificity), and FLAIR/T1 lesion quotient (ROC AUC = 0.55, 95% CI: 0.41 – 0.69, p = 0.4723, 77% sensitivity and 43% specificity). Conversely, parameters derived from our imaging-driven model were able to differentiate lesion etiology with excellent accuracy for tumor cell proliferation rate (ROC AUC = 0.86, 95% CI: 0.76 – 0.95, p < 0.0001, 74% sensitivity and 95% specificity) and Dice similarity coefficient associated with high model-estimated mechanical stresses (ROC AUC = 0.93, 95% CI: 0.86 – 0.99, p < 0.0001, 81% sensitivity and 95% specificity). In patients with BM treated with SRS, our model demonstrated excellent accuracy for differentiating enhancing lesions and significantly outperforms standard radiographic assessment of image morphometric features.
更多查看译文
AI 理解论文
溯源树
样例
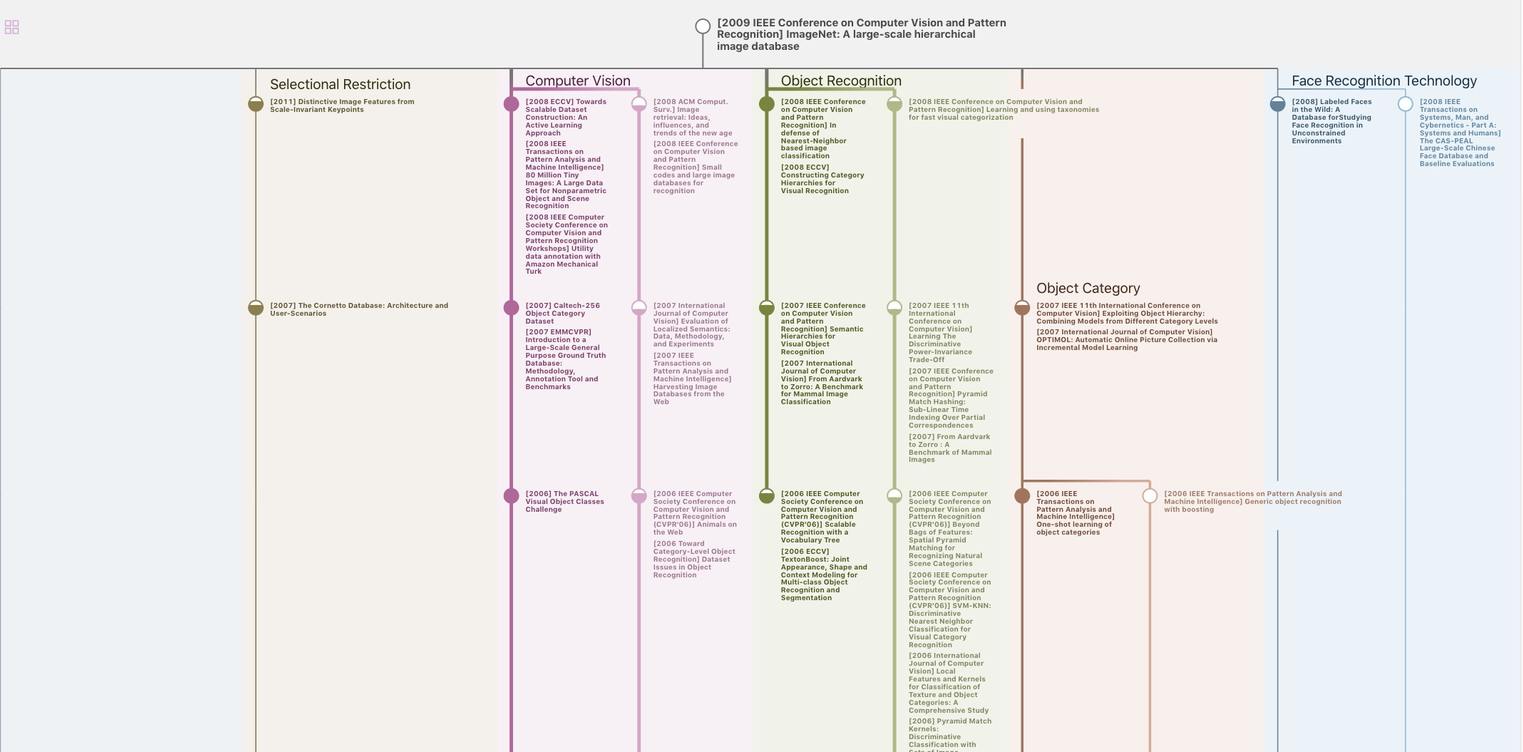
生成溯源树,研究论文发展脉络
Chat Paper
正在生成论文摘要