Composite Quantile Regression Long Short-Term Memory Network
ARTIFICIAL NEURAL NETWORKS AND MACHINE LEARNING - ICANN 2019: TEXT AND TIME SERIES, PT IV(2019)
摘要
Based on quantile long short-term memory (Q-LSTM), we consider the comprehensive utilization of multiple quantiles, proposing a simultaneous estimation version of Q-LSTM, composite quantile regression LSTM (CQR-LSTM). The method simultaneously estimates multiple quantile functions instead of estimating them separately. It makes sense that simultaneous estimation allows multiple quantiles to share strength among them to get better predictions. Furthermore, we also propose a novel approach, noncrossing composite quantile regression LSTM (NCQR-LSTM), to solve the quantile crossing problem. This method uses an indirect way as follows. Instead of estimating multiple quantiles directly, we estimate the intervals between adjacent quantiles. Since the intervals are guaranteed to be positive by using exponential functions, this completely avoids the problem of quantile crossing. Compared with the commonly used constraint methods for solving the quantile crossing problem, this indirect method makes model optimization easier and more suitable for deep learning. Experiments on a real wind speed dataset show that our methods improve the probabilistic prediction performance and reduce the training cost. In addition, our methods are simple to implement and highly scalable.
更多查看译文
关键词
Quantile regression, Long short-term memory network, Noncrossing
AI 理解论文
溯源树
样例
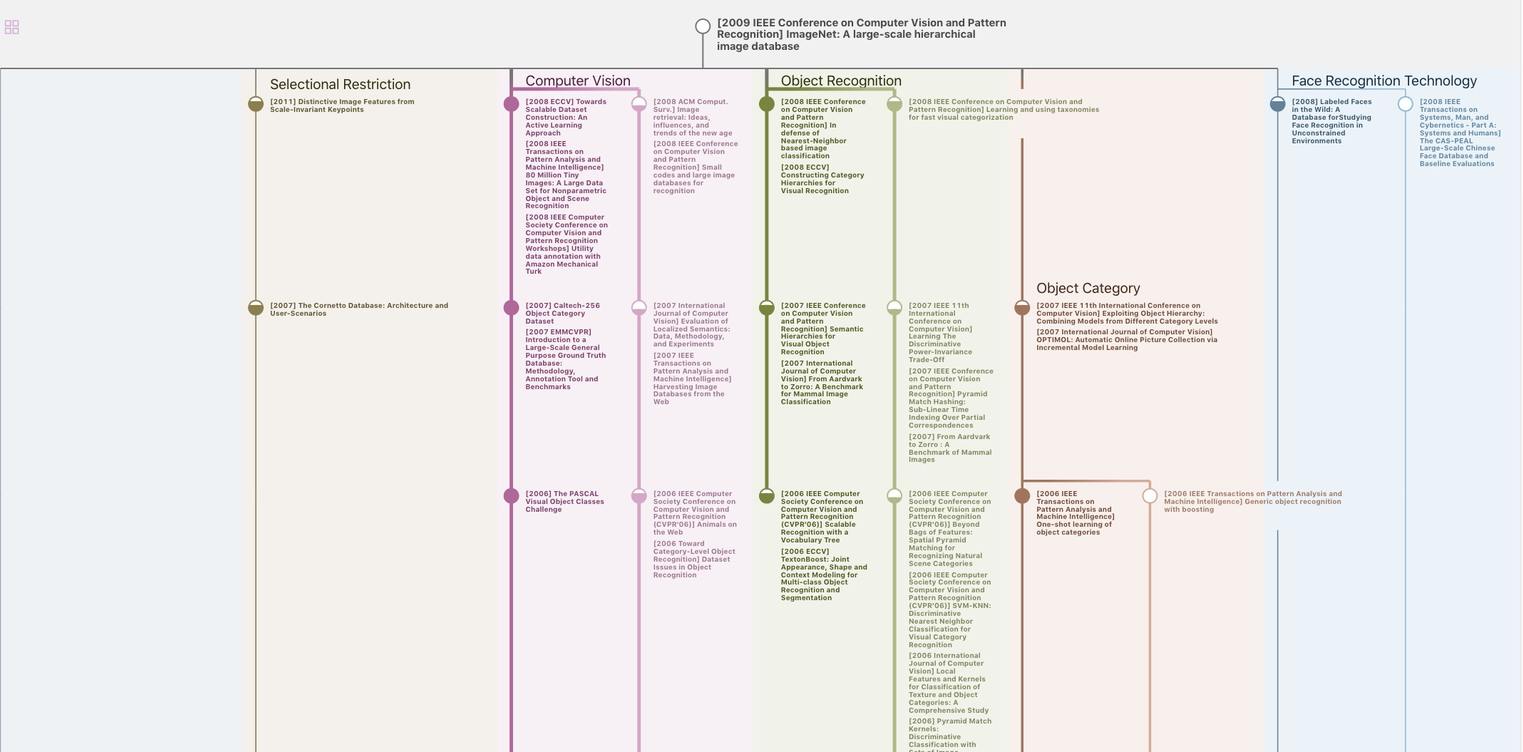
生成溯源树,研究论文发展脉络
Chat Paper
正在生成论文摘要