Phonetically-Aware Embeddings, Wide Residual Networks with Time-Delay Neural Networks and Self Attention Models for the 2018 NIST Speaker Recognition Evaluation
INTERSPEECH(2019)
摘要
Very often, speaker recognition systems do not take into account phonetic information explicitly. In order to gain insight along this line of research, we have studied the use of phonetic information in the embedding extraction process for automatic speaker verification systems in two different ways: on the one hand using the well-known i-vector paradigm and, on the other hand, using Wide Residual Networks (WRN) with Time Delay Neural Networks (TDNN) and Self-Attention Mechanisms. The phonetic information is provided by a WRN with TDNN using 1D convolutional layers specifically trained for this purpose. These two approaches along with the widely used x-vector system based on the Kaldi toolkit were submitted to the 2018 NIST speaker recognition evaluation. As back-end, these representations used a standard PLDA classifier with ad-hoc configurations for each system and in-domain adaptation. The results obtained in the NIST SRE 2018 show that our methods are very promising and it is worth continuing to work on them to improve their performance.
更多查看译文
关键词
NIST-SRE, speaker verification, Wide Residual Networks, Time-Delay Neural Networks, phonetically-aware embeddings
AI 理解论文
溯源树
样例
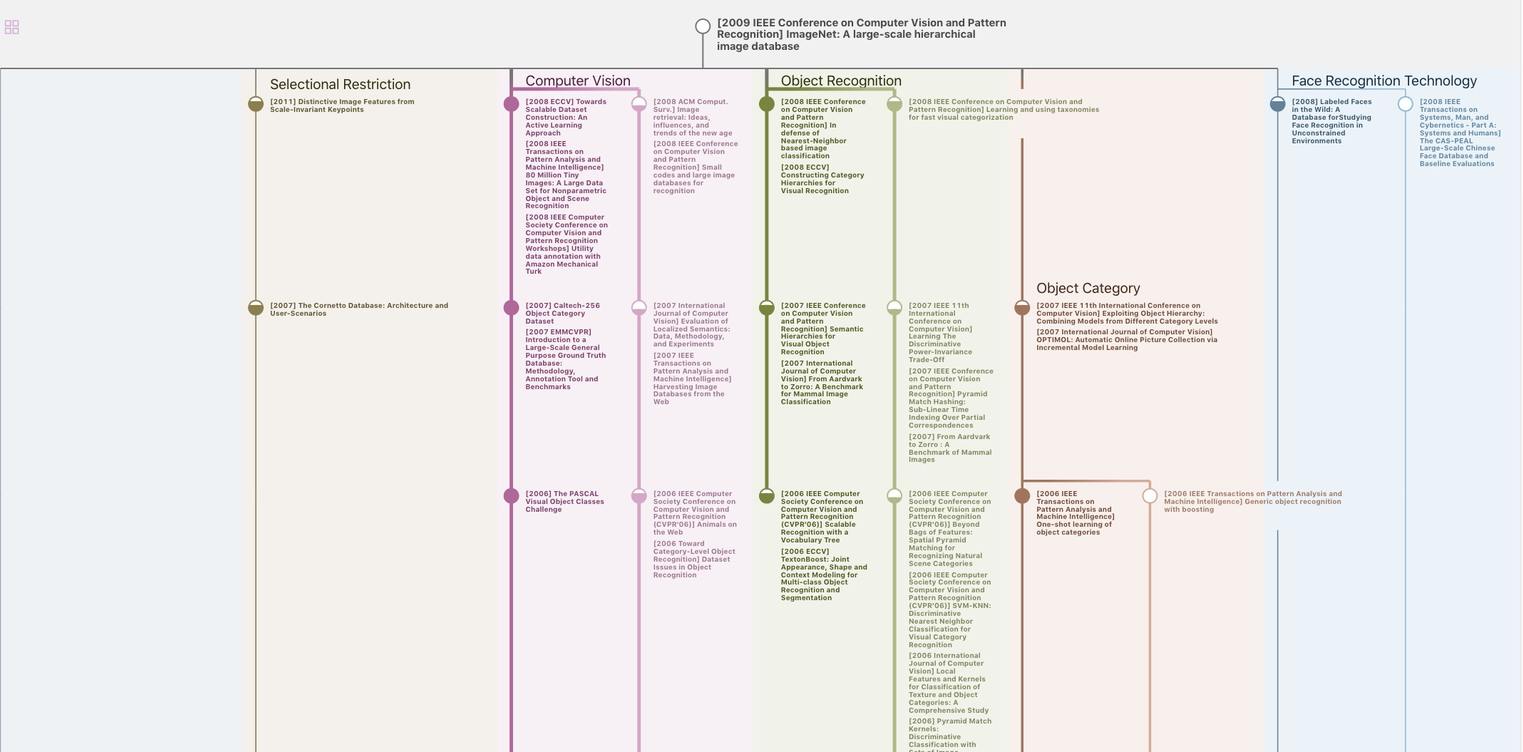
生成溯源树,研究论文发展脉络
Chat Paper
正在生成论文摘要