Clustering Geospatial Data For Multiple Reference Points
IEEE ACCESS(2019)
摘要
Data clustering plays a significant role in geospatial data management and analytics. In this light, we propose and study a novel geospatial data clustering method for multiple reference points. Given a set Q of geospatial data points, a candidate set O of reference points, and a threshold k, each data point q will be matched to its closest reference point o. The multi-reference clustering (MRC) method finds a subset A (A subset of O boolean AND vertical bar A vertical bar <= k) reference points from O which define the minimum global travel distance (Sigma(for all q is an element of Q,o is an element of A) d(q, o)), hence the data are grouped into vertical bar A vertical bar clusters. We believe that the MRC method may benefit a lot of applications including geospatial data clustering, data classification, and data analytics iPn general. The MRC problem is challenging due to its high computation complexity, and there exist Sigma(k)(i=1) C-vertical bar O vertical bar(i) = Sigma(k)(i=1) vertical bar O vertical bar!/i!(vertical bar O vertical bar-i)! possibilities for subset A. Because the exact solution cannot be computed in real time, we develop a heuristic method to select subset A from O efficiently. The experimental results show that the accuracy of A is very close to the optimal solution. In addition, we also develop a set of optimization techniques to further enhance the efficiency. Finally, we conduct extensive experiments to study the efficiency and accuracy of the heuristic method.
更多查看译文
关键词
Multi-reference clusters, approximation, efficiency, accuracy, geospatial data
AI 理解论文
溯源树
样例
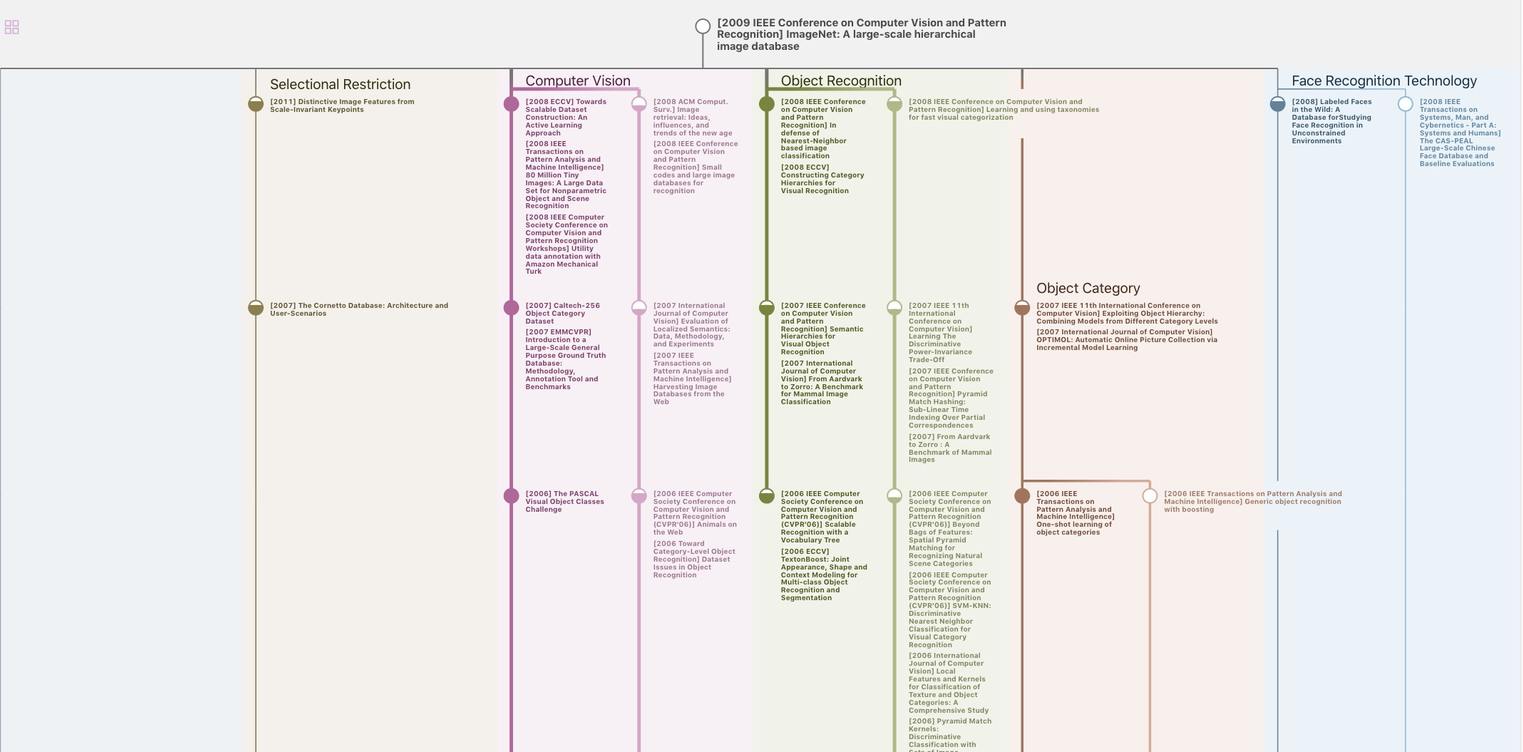
生成溯源树,研究论文发展脉络
Chat Paper
正在生成论文摘要