Dr.VOT: Measuring Positive and Negative Voice Onset Time in the Wild
INTERSPEECH(2019)
摘要
Voice Onset Time (VOT), a key measurement of speech for basic research and applied medical studies, is the time between the onset of a stop burst and the onset of voicing. When the voicing onset precedes burst onset the VOT is negative; if voicing onset follows the burst, it is positive. In this work, we present a deep-learning model for accurate and reliable measurement of VOT in naturalistic speech. The proposed system addresses two critical issues: it can measure positive and negative VOT equally well, and it is trained to be robust to variation across annotations. Our approach is based on the structured prediction framework, where the feature functions are defined to be RNNs. These learn to capture segmental variation in the signal. Results suggest that our method substantially improves over the current state-of-the-art. In contrast to previous work, our Deep and Robust VOT annotator, Dr.VOT, can successfully estimate negative VOTs while maintaining state-of-the-art performance on positive VOTs. This high level of performance generalizes to new corpora without further retraining. Index Terms: structured prediction, multi-task learning, adversarial training, recurrent neural networks, sequence segmentation.
更多查看译文
关键词
negative voice onset time
AI 理解论文
溯源树
样例
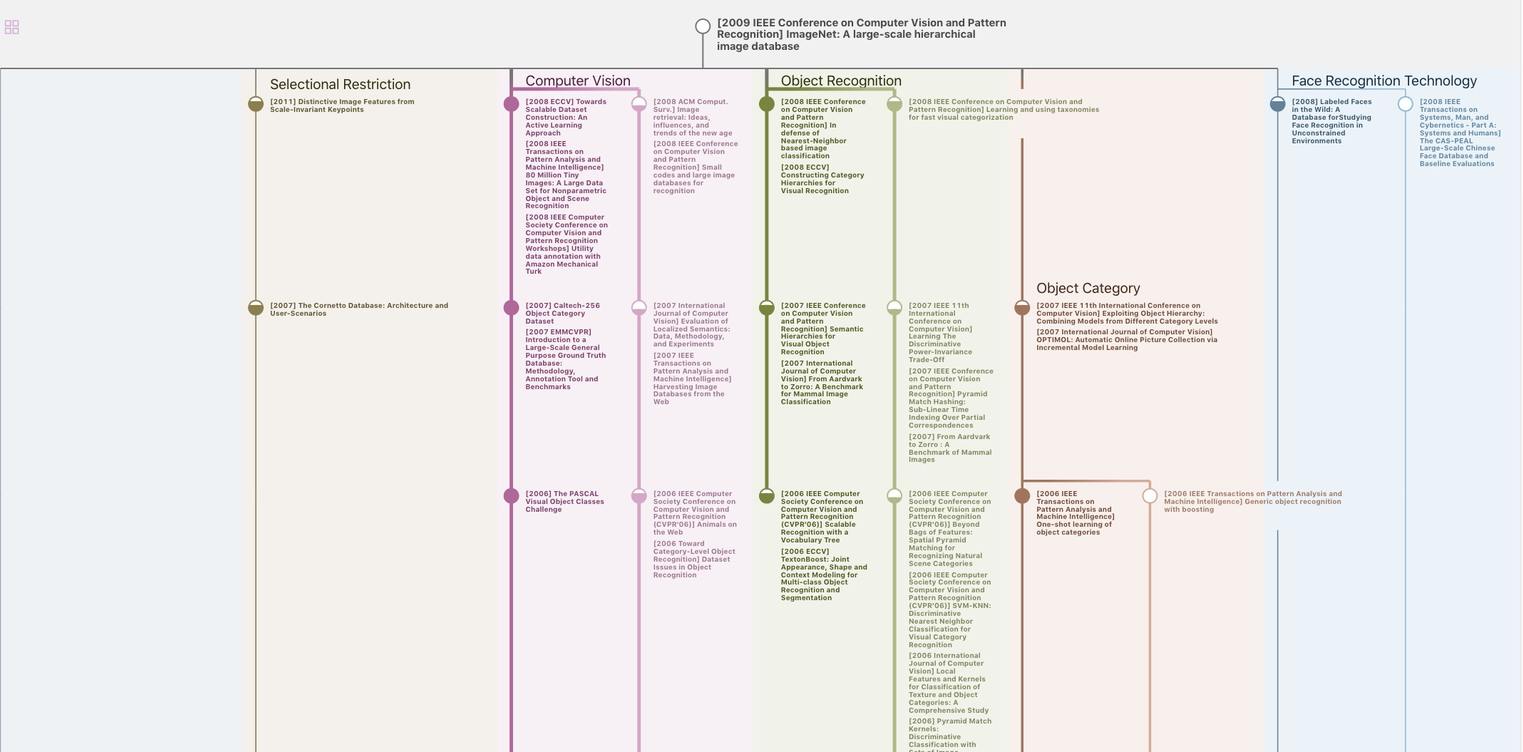
生成溯源树,研究论文发展脉络
Chat Paper
正在生成论文摘要