A Non-Causal FFTNet Architecture for Speech Enhancement
INTERSPEECH(2019)
摘要
In this paper, we suggest a new parallel, non-causal and shallow waveform domain architecture for speech enhancement based on FFTNet, a neural network for generating high quality audio waveform. In contrast to other waveform based approaches like WaveNet, FFTNet uses an initial wide dilation pattern. Such an architecture better represents the long term correlated structure of speech in the time domain, where noise is usually highly non-correlated, and therefore it is suitable for waveform domain based speech enhancement. To further strengthen this feature of FFTNet, we suggest a non-causal FFTNet architecture, where the present sample in each layer is estimated from the past and future samples of the previous layer. By suggesting a shallow network and applying non-causality within certain limits, the suggested FFTNet for speech enhancement (SE-FFTNet) uses much fewer parameters compared to other neural network based approaches for speech enhancement like WaveNet and SEGAN. Specifically, the suggested network has considerably reduced model parameters: 32% fewer compared to WaveNet and 87% fewer compared to SEGAN. Finally, based on subjective and objective metrics, SE-FFTNet outperforms WaveNet in terms of enhanced signal quality, while it provides equally good performance as SEGAN. A Tensorflow implementation of the architecture is provided at 1 .
更多查看译文
关键词
speech enhancement, computational complexity, dilation width, FFTNet, WaveNet
AI 理解论文
溯源树
样例
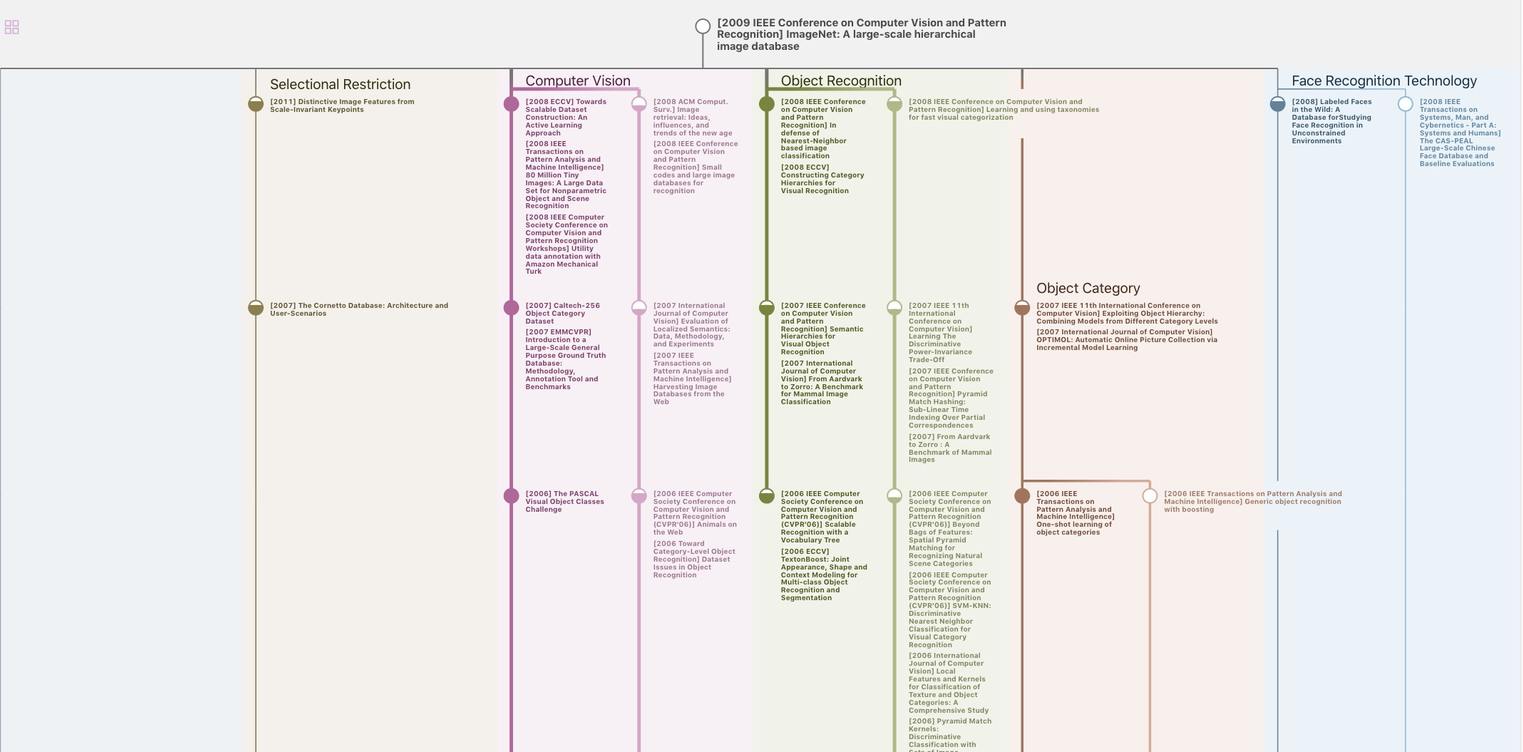
生成溯源树,研究论文发展脉络
Chat Paper
正在生成论文摘要