Leveraging Domain Knowledge For Reinforcement Learning Using Mmc Architectures
ARTIFICIAL NEURAL NETWORKS AND MACHINE LEARNING - ICANN 2019: DEEP LEARNING, PT II(2019)
摘要
Despite the success of reinforcement learning methods in various simulated robotic applications, end-to-end training suffers from extensive training times due to high sample complexity and does not scale well to realistic systems. In this work, we speed up reinforcement learning by incorporating domain knowledge into policy learning. We revisit an architecture based on the mean of multiple computations (MMC) principle known from computational biology and adapt it to solve a "reacher task". We approximate the policy using a simple MMC network, experimentally compare this idea to end-to-end deep learning architectures, and show that our approach reduces the number of interactions required to approximate a suitable policy by a factor of ten.
更多查看译文
AI 理解论文
溯源树
样例
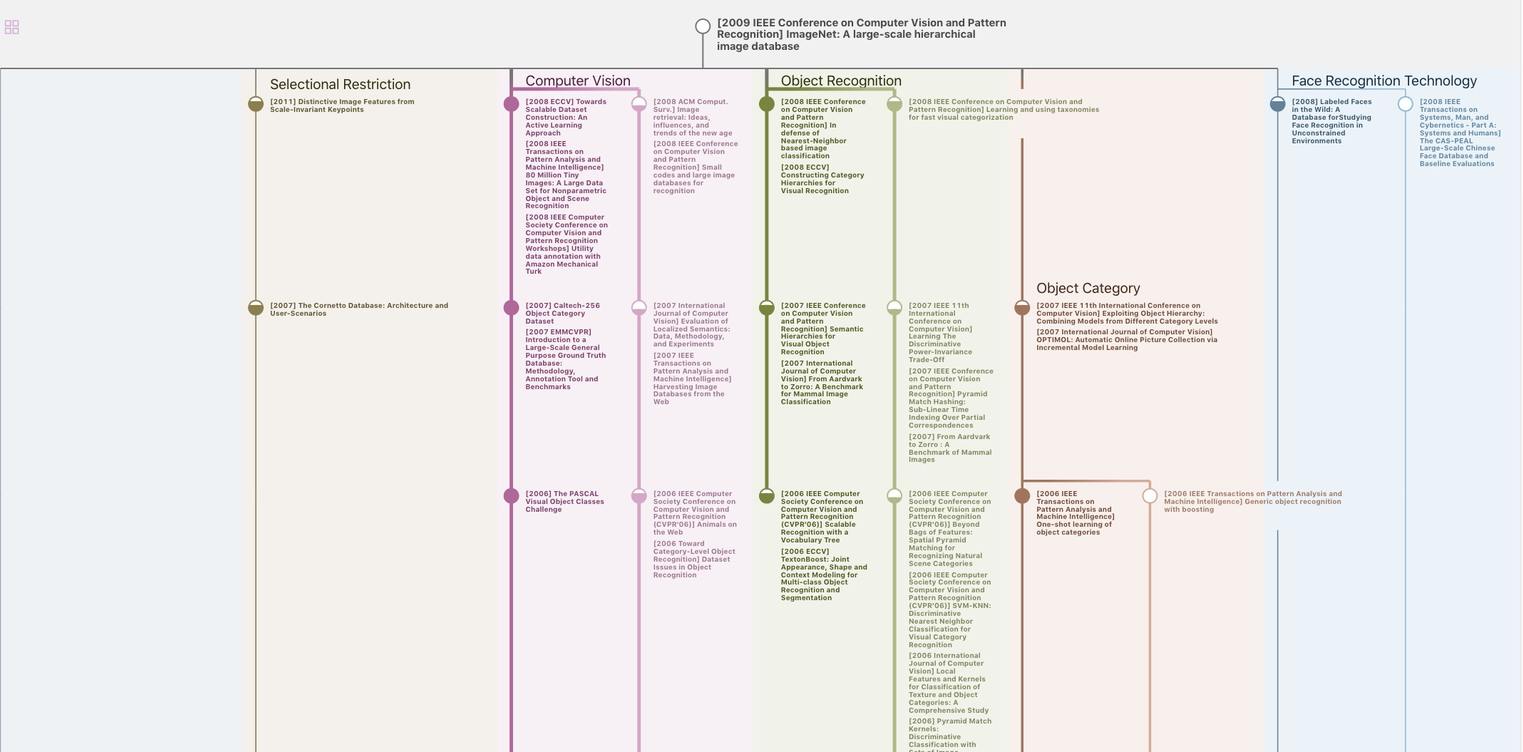
生成溯源树,研究论文发展脉络
Chat Paper
正在生成论文摘要