Eye Diagram Measurement-Based Joint Modulation Format, OSNR, ROF, and Skew Monitoring of Coherent Channel Using Deep Learning
Journal of lightwave technology(2019)
摘要
In this work, deep learning is used to monitor coherent channel performance with eye diagram measurement. Experiments show that the proposed technique can determine the modulation format, optical signal to noise ratio (OSNR), roll-off factor (ROF), and timing skew of a quadrature amplitude modulation (QAM) transmitter with high accuracy. Trained vanilla convolutional neural network (CNN) and MobileNet can be utilized to jointly monitor above four parameters with >98% prediction accuracy for 32 GBd coherent channels with quadrature phase shift keying (QPSK), 8-QAM or 16-QAM formats, under OSNR from 15 to 40 dB, IQ skew from −15 to 15 ps, ROF from 0.05 to 1. Our proposed deep learning approach outperforms many traditional machine learning methods, such as decision tree, k-nearest neighbor algorithm (KNN), and histogram of oriented gradient (HOG) based support vector machine (SVM). Unlike other optical performance monitoring approaches, the use of eye diagram measurement combined with deep learning could enable joint monitoring of multiple system performance parameters with reduced hardware implementation complexity. Comparing with vanilla CNN, MobileNet has relatively simplified iteration algorithm, thus reduces the requirement on the computational power, while still maintaining high accuracy for classification issues.
更多查看译文
关键词
Coherent communications,convolutional neural networks,deep learning,optical performance monitoring
AI 理解论文
溯源树
样例
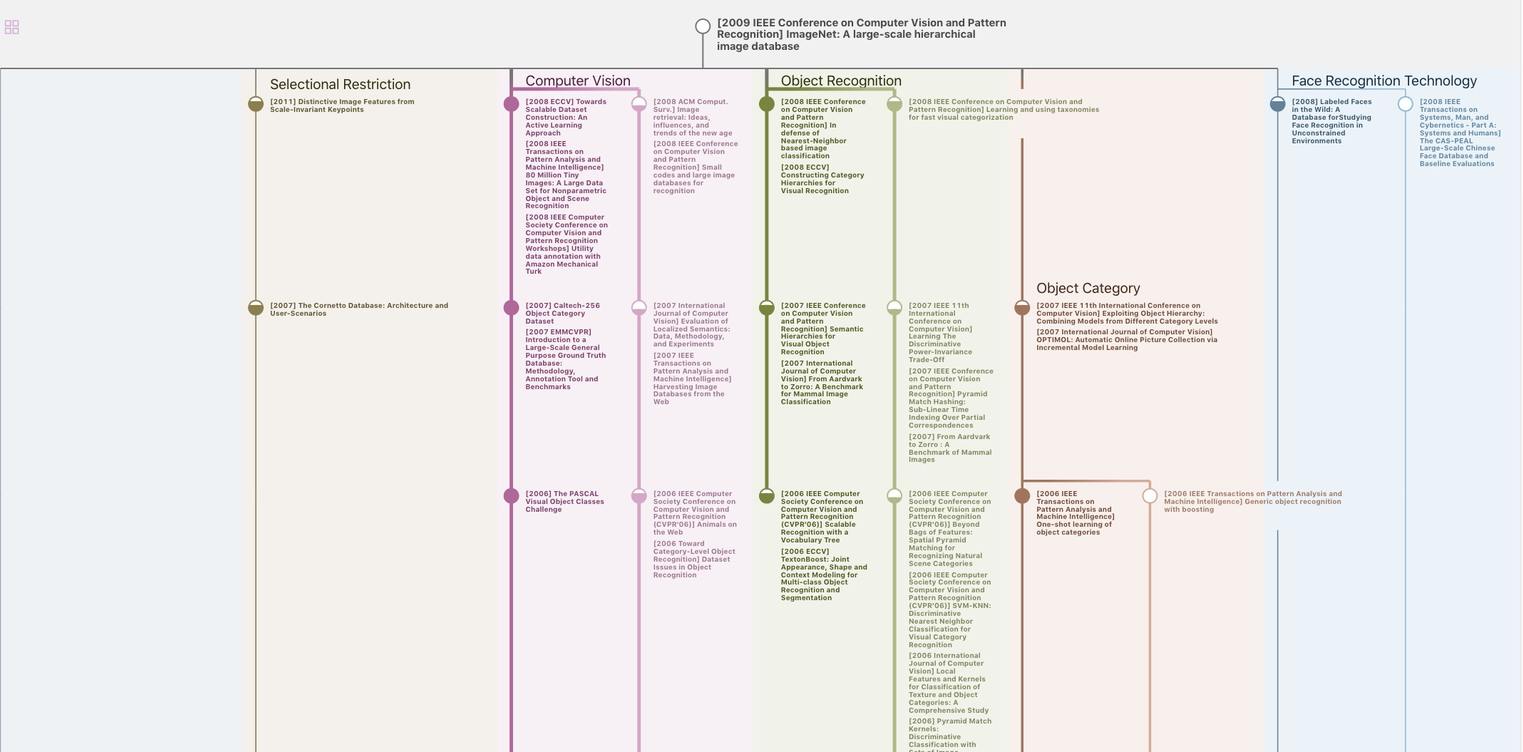
生成溯源树,研究论文发展脉络
Chat Paper
正在生成论文摘要