Densely Connected Convolutional Networks with Attention LSTM for Crowd Flows Prediction
IEEE Access(2019)
摘要
With the rapid progress of urbanization, predicting citywide crowd flows has become increasingly significant in many fields, such as traffic management and public security. However, influenced by the complex spatiotemporal relations in raw data and other factors, such as events and weather, obtaining a precise prediction is challenging. Some previous works attempted to address this problem using various ways, such as autoregressive integrated moving average, vector auto-regression and some deep learning models. However, seldom can these methods comprehensively capture the spatiotemporal correlations. In this paper, we propose a novel spatio-temporal prediction model that is based on densely connected convolutional networks and attention long short-term memory (ST-DCCNAL), to simultaneously predict the inflow and outflow of the crowds in regions divided within a specific city. The ST-DCCNAL model consists of three parts: spatial part, external factors part and temporal part. In the spatial part, we employ densely connected convolutional networks to extract spatial characteristics at different levels. The external factors part utilizes a fully connected network to extract features from auxiliary information. In the last part, an attention-based long short-term memory module is leveraged to capture the temporal pattern. To demonstrate the practicality and effectiveness of the proposed model, we evaluate it using two separate real-world datasets of taxis in Beijing and bikes in New York. The experimental results confirm that the performance of our model is better than that of other baseline methods.
更多查看译文
关键词
Spatiotemporal phenomena,Correlation,Feature extraction,Predictive models,Urban areas,Meteorology,Time series analysis,Data mining,spatiotemporal modeling,crowd flow prediction,densely connected convolutional network,long short-term memory,attention mechanism
AI 理解论文
溯源树
样例
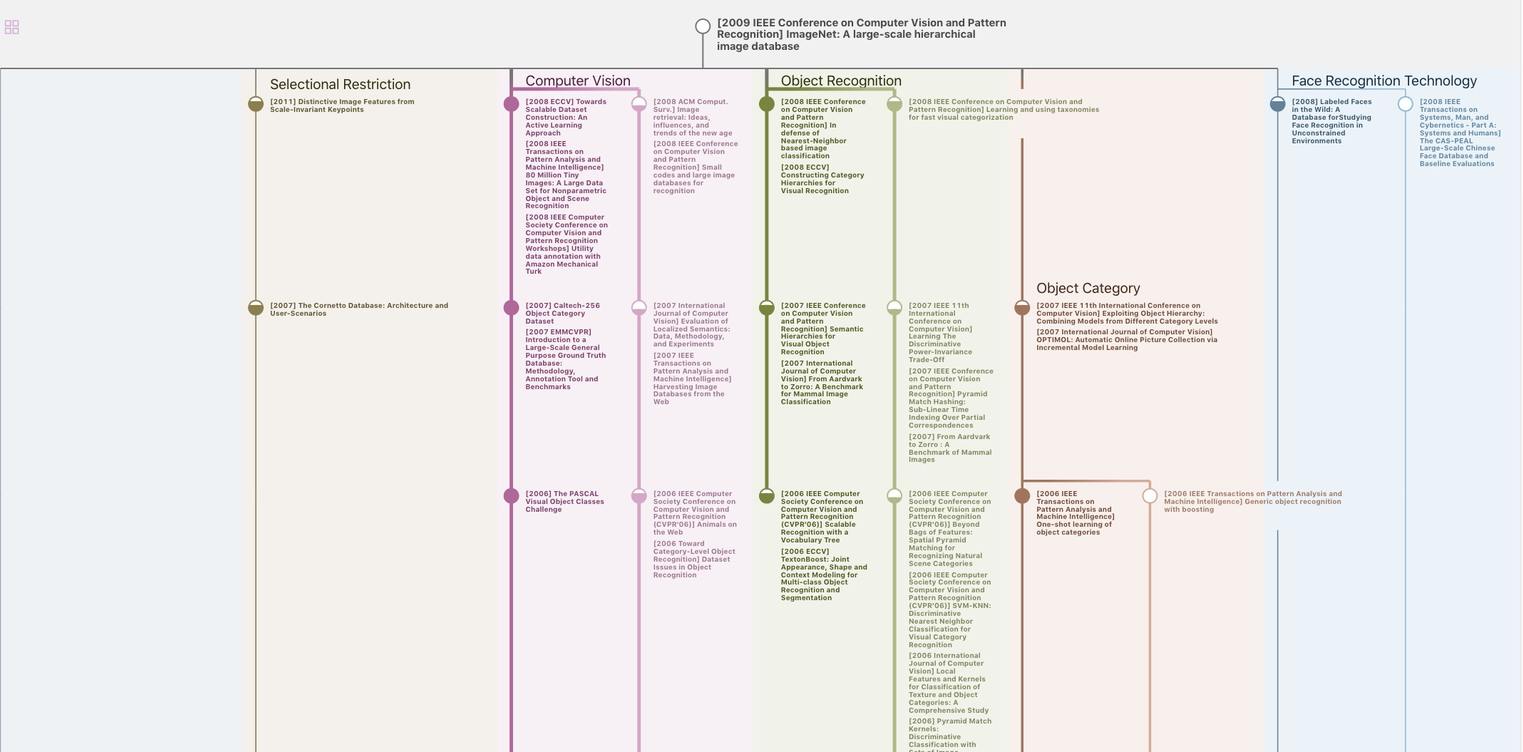
生成溯源树,研究论文发展脉络
Chat Paper
正在生成论文摘要