CCFR2: A more efficient cooperative co-evolutionary framework for large-scale global optimization
Information Sciences(2020)
摘要
Cooperative co-evolution (CC) is an explicit means of divide-and-conquer strategy in evolutionary algorithms for solving large-scale optimization problems. The subcomponents generated by CC may have different characteristics. When optimizing the subcomponents, the settings of the subpopulations should take the characteristics into account. CCFR is a previously published CC framework which allocates computational resources among subpopulations according to the contributions of subpopulations to the improvement of the best overall objective value. In this paper, we propose an improved version of CCFR named CCFR2, which can specify unequal-sized subpopulations for optimizing different subcomponents of variables. CCFR2 computes the average improvement of the best overall objective value per fitness evaluation as the contribution of a subpopulation, which considers the subpopulation size in the contribution computation. A control parameter is adopted by CCFR2 to balance the effects of the historical and real-time improvements of the best overall objective value on the contribution computation. Compared with CCFR, CCFR2 is able to save computational resources from obtaining the best overall solution before the evolution starts and evaluating individuals in co-evolutionary cycles. Our experimental results and analysis suggest that CCFR2 improves the performance of CCFR and is a more efficient CC framework for solving large-scale optimization problems.
更多查看译文
关键词
Cooperative co-evolution,Large-scale global optimization,Decomposition,Resource allocation
AI 理解论文
溯源树
样例
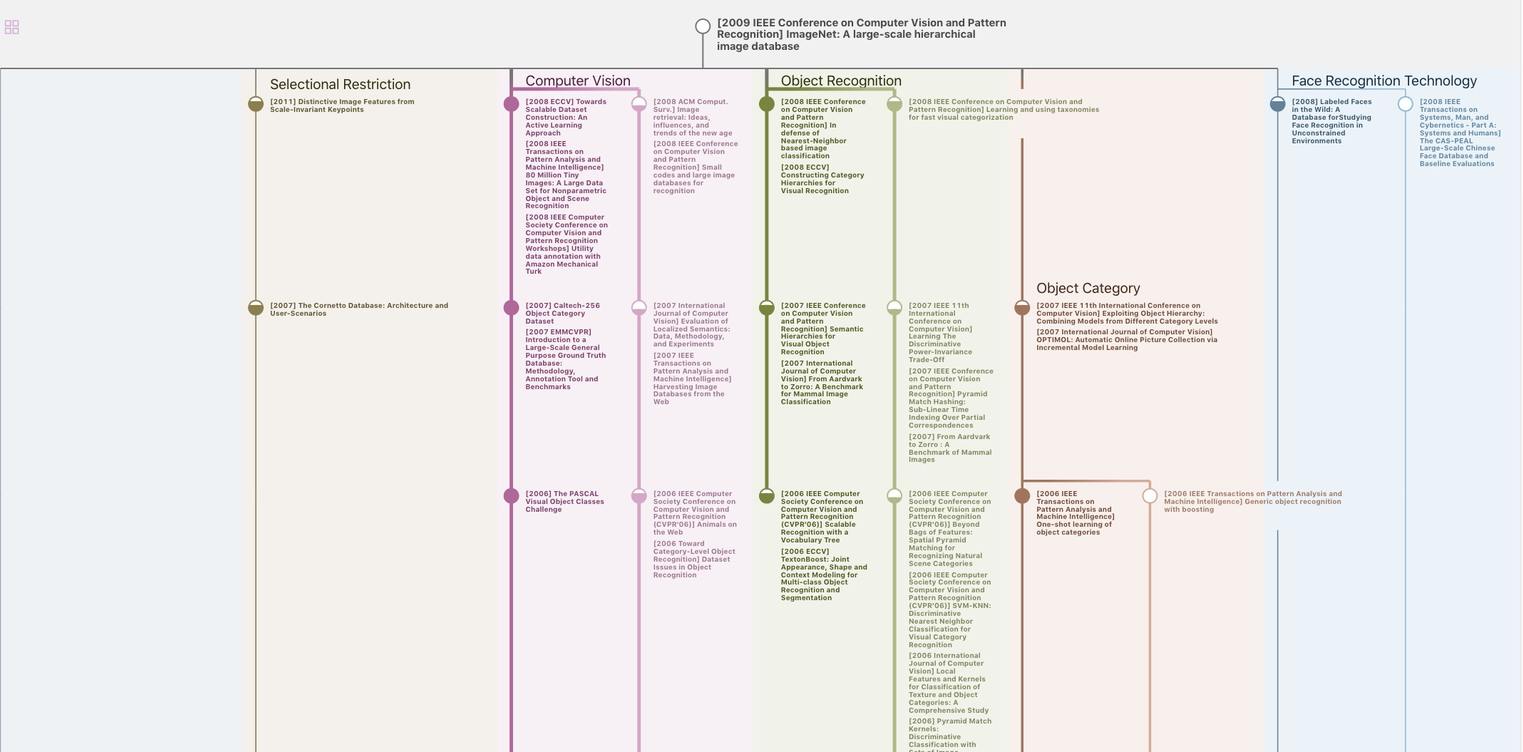
生成溯源树,研究论文发展脉络
Chat Paper
正在生成论文摘要