Non-Stationary Spatial Regression For Modelling Monthly Precipitation In Germany
SPATIAL STATISTICS(2020)
摘要
It is widely accepted that spatial dependencies have to be acknowledged appropriately in data that are spatially aligned. However, most spatial models still assume that the dependence structure does not vary over space, i.e., it is stationary. While assuming stationarity considerably facilitates estimation, it is often too restrictive when describing atmospheric phenomena such as precipitation. Nonetheless, the applicability of non-stationary models is often hindered, as their use reveals to be cumbersome and improvements over stationary models can be hard to identify. The stochastic partial differential equation (SPDE) approach to spatial modelling allows for flexible specifications of non-stationary models. In particular, given the German orographic diversity, it makes sense to investigate potential non-stationarity in precipitation patterns. Taking such potential non-stationarities into account may, in particular, leads to improved smoothing of the observed precipitation pattern taken on a finite set of measurement stations, and therefore to improved inputs to hydrological models. We suggest an SPDE-based model where the mean and dependence structure are allowed to vary with elevation, as well as a more flexible non-parametric alternative based on multivariate B-Splines. As factors such as wind may cause higher dependence in a given direction, we include anisotropy in the model. Results show that, according to the widely applicable Bayesian information criterion, a non-stationary model provides a better fit to the data. Taking German monthly precipitation as a motivation, we set up a simulation study to explore the ability of the elevation and spline-based models to correctly identify non-stationarity. (C) 2019 Elsevier B.V. All rights reserved.
更多查看译文
关键词
Gaussian (Markov) random fields, Integrated nested Laplace approximations, Monthly precipitation, Non-stationary spatial modelling, Stochastic partial differential equation
AI 理解论文
溯源树
样例
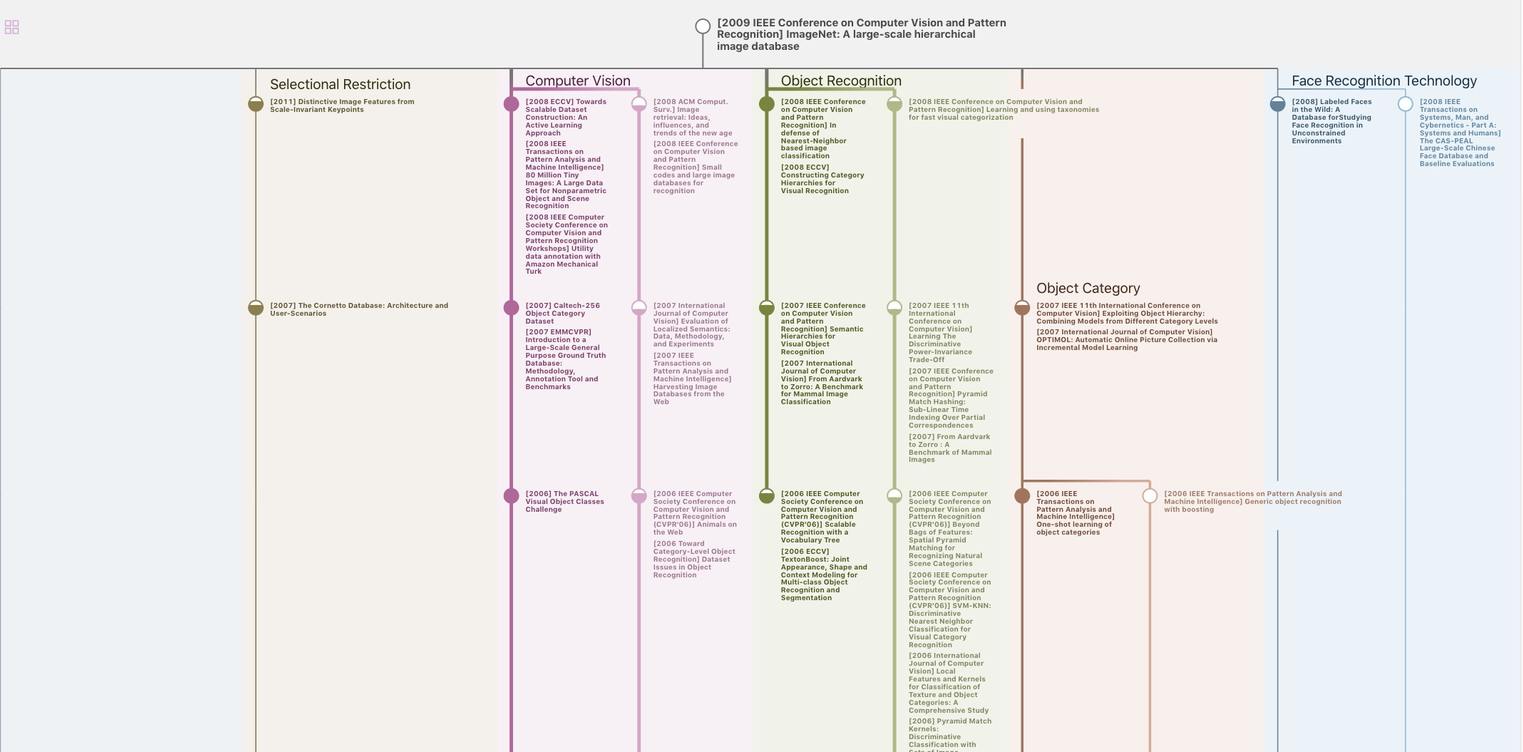
生成溯源树,研究论文发展脉络
Chat Paper
正在生成论文摘要