Select the Best Translation from Different Systems Without Reference
Lecture Notes in Artificial Intelligence(2019)
摘要
In recent years, neural machine translation (NMT) has made great progress. Different models, such as neural networks using recurrence, convolution and self-attention, have been proposed and various online translation systems can be available. It becomes a big challenge on how to choose the best translation among different systems. In this paper, we attempt to tackle this task and it can be intuitively considered as the Quality Estimation (QE) problem that requires enough human-annotated data in which each translation hypothesis is scored by human. However, we do not have rich data with high-quality human annotations in practice. To solve this problem, we resort to bilingual training data and propose a new method of mixed MT metrics to automatically score the translation hypotheses from different systems with their references so as to construct the pseudo human-annotated data. Based on the pseudo training data, we further design a novel QE model based on Multi-BERT and Bi-RNN with a joint-encoding strategy. Extensive experiments demonstrate that our proposed method can achieve promising results for the task to select the best translation from various systems.
更多查看译文
关键词
Machine translation,Evaluation,Deep learning
AI 理解论文
溯源树
样例
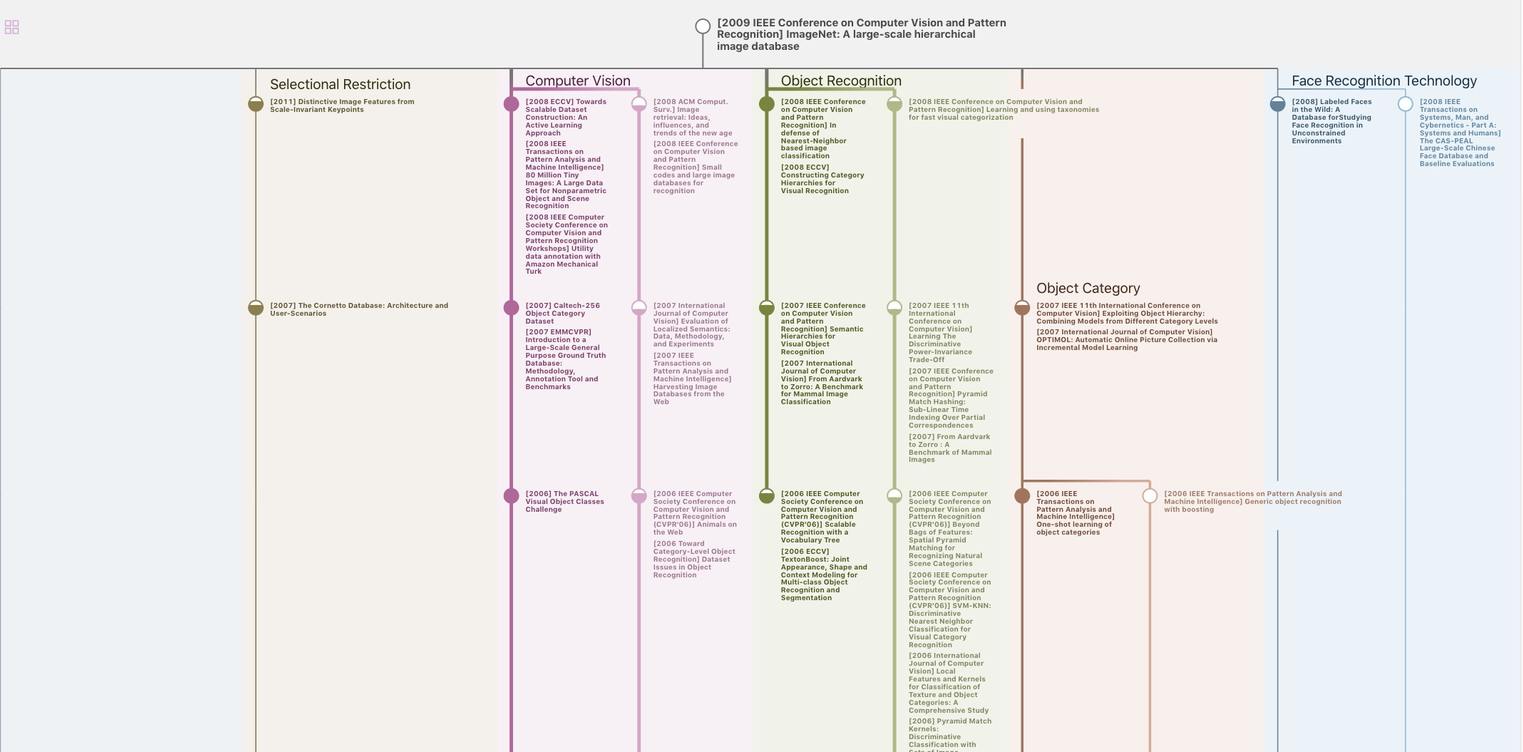
生成溯源树,研究论文发展脉络
Chat Paper
正在生成论文摘要