Evaluation of CT-based Radiomics in Patients with Renal Cell Carcinoma
Annals of oncology(2019)
摘要
Background Although the personalized medicine has always focused on the genomic or proteomic characterization of tumor, medical imaging is still one of the major factors to guide therapy and to monitor the progression of the tumor. Radiomics is an emerging field that converts the medical image data into the mineable quantitative features via the automatically algorithms, and can server as a bridge between medical image, genomics and clinical-parameters. Serval studies have demonstrated that the radiomic-based model can predict outcome of RCC, but the correlation between radiomic features and histological subtypes of RCC is still unknown. The aim of this letter is to focus on the ability of radiomics to identify the histological subtypes and metastasis of RCC. Methods This study included Forty-four patients diagnosed with renal tumor. For each renal lesion, The CT images of volume of interest (VOI) were obtained semi-automatically by two experienced nuclear medicine physician, 85 texture features were extracted from each VOI using the first-order statistics features, Shape Based Features, Gray Level Neighboring Gray Level Dependence Matrix and Neighboring Gray Tone Difference Matrix. Results To investigate the value of radiomic features to capture phenotypic differences of RCC, we performed Unsupervised Clustering of patients with similar radiomic expression patterns. We analyzed the two main clusters of patients with clinical parameters, and found that the tumor clusters were statistically and significantly associated with primary tumor stage (P Conclusions The radiomic features from medical images could be helpful in deciphering T-stages, metastasis and benign of RCC and may have potential as imaging biomarker for prediction of RCC overall survival. Legal entity responsible for the study Zhejiang Cancer Hospital. Funding National Natural Science Foundation of China (No 81402117, 81671775), Natural Science Foundation of Zhejiang Province (No LY17H160043) and Qianjiang talent plan of Zhejiang Province (No QJD1602025). Disclosure All authors have declared no conflicts of interest.
更多查看译文
AI 理解论文
溯源树
样例
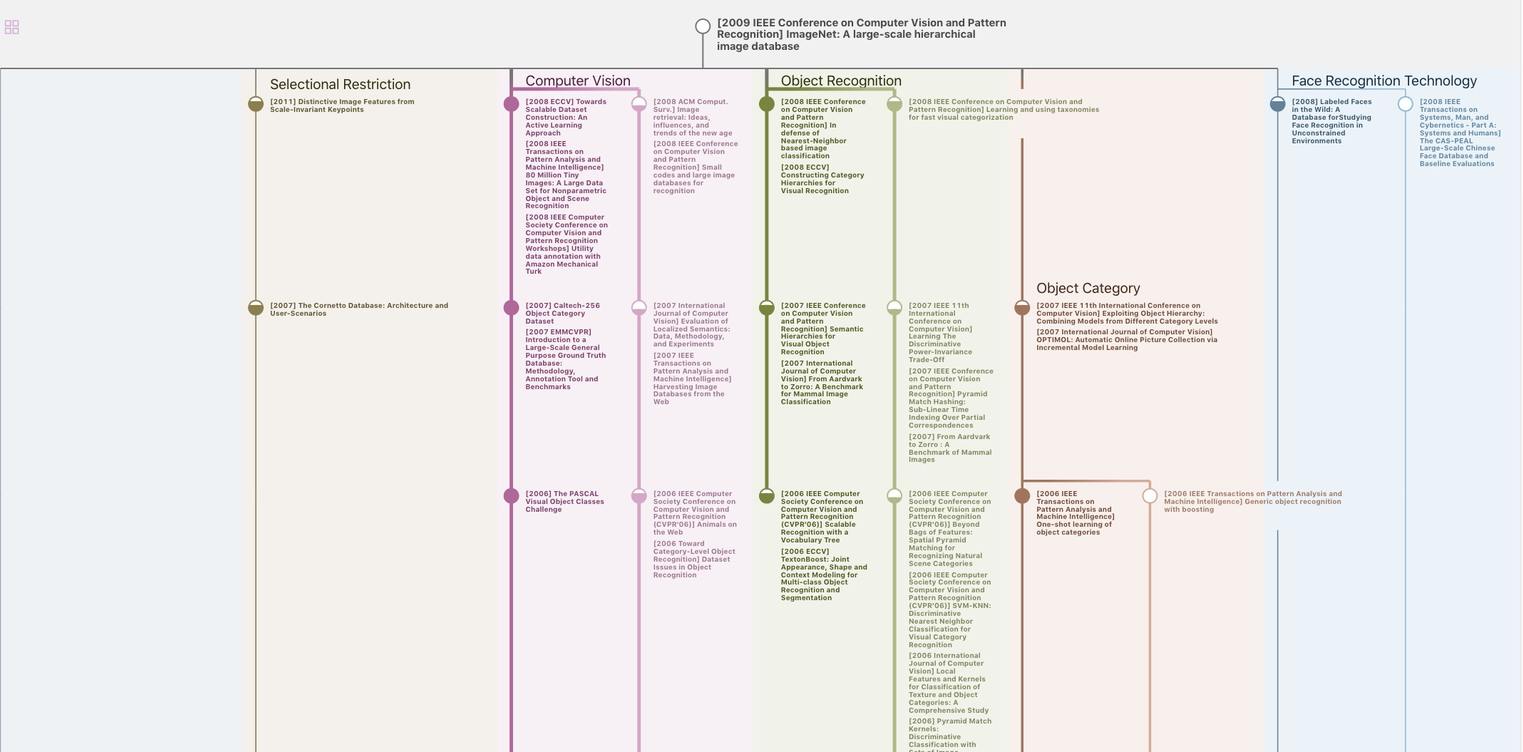
生成溯源树,研究论文发展脉络
Chat Paper
正在生成论文摘要