Deep artifact-free residual network for single-image super-resolution
Signal, Image and Video Processing(2019)
摘要
Recently, convolutional neural networks have shown promising performance for single-image super-resolution. In this paper, we propose deep artifact-free residual (DAFR) network which uses the merits of both residual learning and usage of ground-truth image as target. Our framework uses a deep model to extract the high-frequency information which is necessary for high-quality image reconstruction. We use a skip connection to feed the low-resolution image to network before the image reconstruction. In this way, we are able to use the ground-truth images as target and avoid misleading the network due to artifacts in difference image. In order to extract clean high-frequency information, we train network in two steps. First step is a traditional residual learning which uses the difference image as target. Then, the trained parameters of this step are transferred to the main training in second step. Our experimental results show that the proposed method achieves better quantitative and qualitative image quality compared to the existing methods.
更多查看译文
关键词
Super-resolution, Deep learning, Convolutional neural networks
AI 理解论文
溯源树
样例
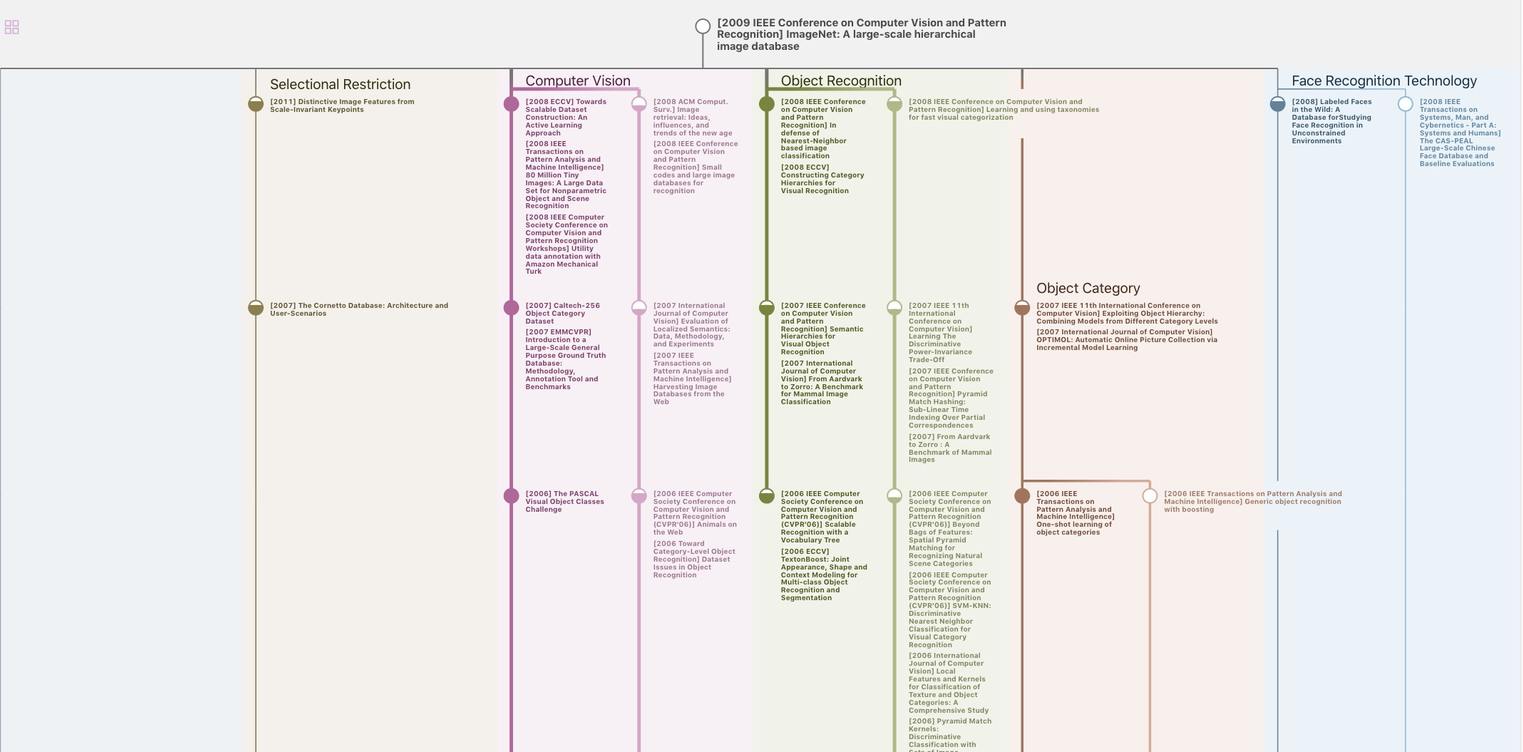
生成溯源树,研究论文发展脉络
Chat Paper
正在生成论文摘要