A cross-media heterogeneous transfer learning for preventing over-adaption
Applied Soft Computing(2019)
摘要
Cross-media heterogeneous transfer learning aims to transfer knowledge from the source media domain to the target media domain, which promotes the performance of the learned model for the target media domain. Existing cross-media heterogeneous transfer learning methods usually attempt to learn the latent feature space with a large amount of co-occurrence data. However, there is a significant challenge: domain over-adaption. In this paper, we propose a Cross-Media Heterogeneous Transfer Learning for Preventing Over-adaption (CMHTL-PO) to address this challenge. The divergence between the different media feature spaces is very large. Each media space has some weak correlation features which have no semantic corresponding features in other media. When the co-occurrence data are not enough, if the weak correlation features are compulsively mapped into the common features in the latent space, it will lead to over-adaption. CMHTL-PO divides the features into the strong correlation features and the weak correlation features, which are respectively mapped into the common features and the peculiar features in the latent space. Extensive experiments are conducted on two benchmark datasets widely adopted in transfer learning to verify the superiority of our proposed CMHTL-PO over existing state-of-the-art Heterogeneous Transfer Learning methods.
更多查看译文
关键词
Cross-media heterogeneous transfer learning,Canonical correlation analysis,Sparse coding
AI 理解论文
溯源树
样例
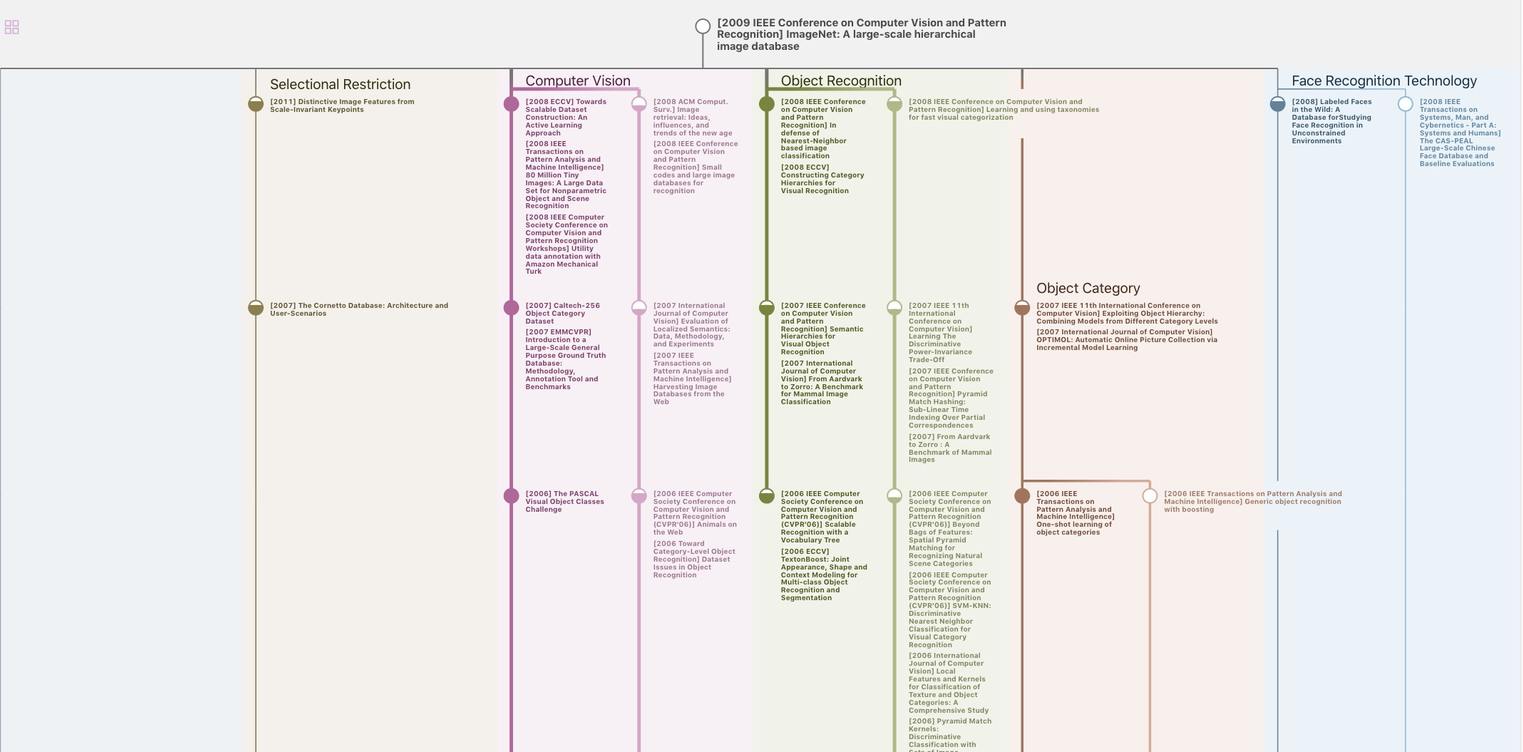
生成溯源树,研究论文发展脉络
Chat Paper
正在生成论文摘要