Using Bidirectional Transformer-CRF for Spoken Language Understanding
Lecture Notes in Artificial Intelligence(2019)
摘要
Spoken Language Understanding (SLU) is a critical component in spoken dialogue systems. It is typically composed of two tasks: intent detection (ID) and slot filling (SF). Currently, most effective models carry out these two tasks jointly and often result in better performance than separate models. However, these models usually fail to model the interaction between intent and slots and ties these two tasks only by a joint loss function. In this paper, we propose a new model based on bidirectional Transformer and introduce a padding method, enabling intent and slots to interact with each other in an effective way. A CRF layer is further added to achieve global optimization. We conduct our experiments on benchmark ATIS and Snips datasets, and results show that our model achieves state-of-the-art on both tasks.
更多查看译文
关键词
SLU,Transformer,CRF,Joint method
AI 理解论文
溯源树
样例
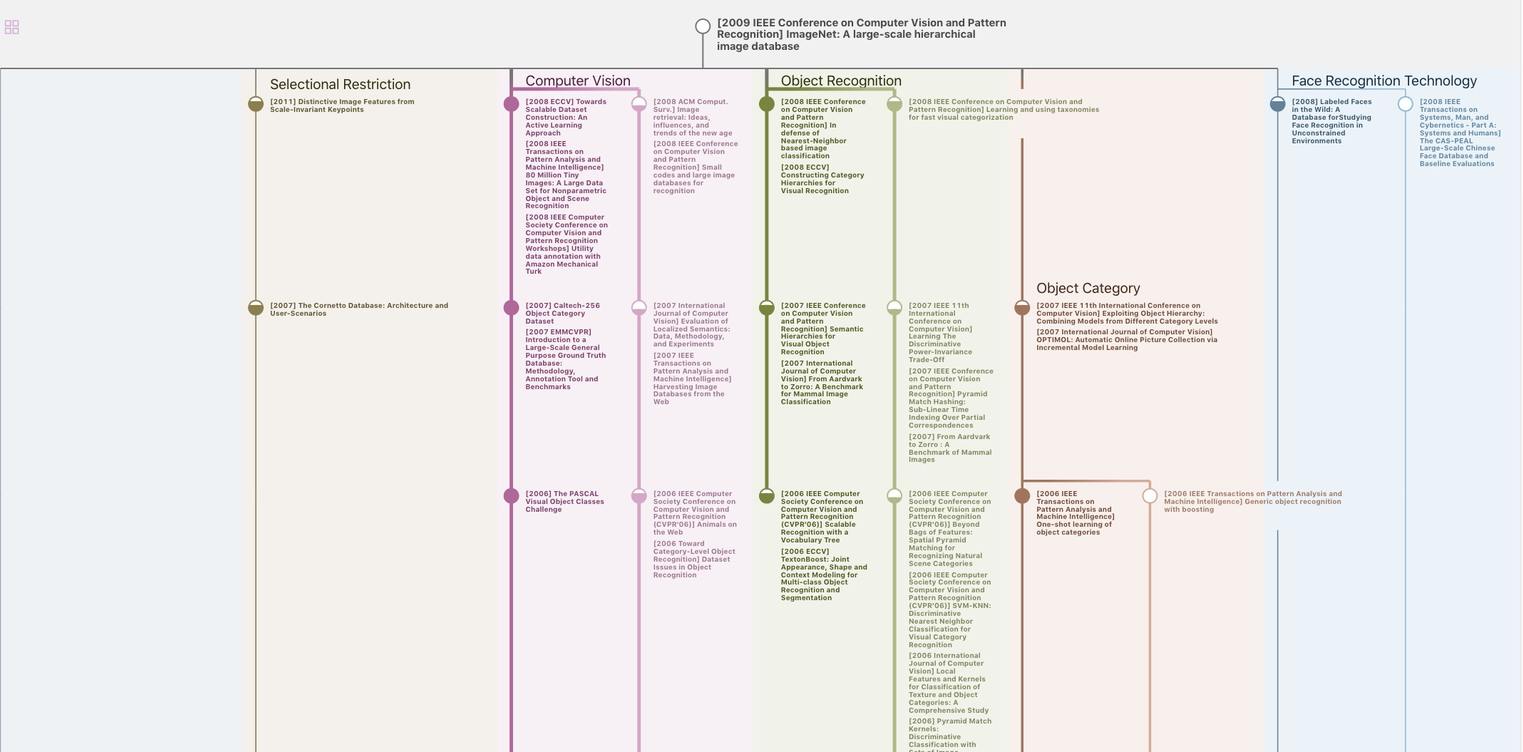
生成溯源树,研究论文发展脉络
Chat Paper
正在生成论文摘要