Correction Learning for Medical Image Segmentation
IEEE Access(2019)
摘要
Breast tumor segmentation is useful to diagnose breast cancer. However, challenges, such as intensity inhomogeneity and shadowing artifacts arise in this task. To address these two issues, this paper proposes a robust ultrasound image segmentation method based on correction learning. At first, a novel idea of correction learning is introduced. In contrast to traditional methods that develop the complex models to obtain accurate segmentation results, correction learning aims to detect the erroneous segmentation in advance and correct this automatically by only using simple method. The proposed correction learning method mainly involves two steps: coarse segmentation and correction learning. First, an active contour model is firstly constructed for coarse segmentation by introducing assumption of bias field and local intensity clustering property. Then, correction learning is developed to address the erroneous segmentation and to improve the segmentation performance. In this paper, correction learning mainly contains Internal Tumor Block Correction (ITBC) and Boundary Block Correction(BBC). In order to correct the erroneous segmentation caused by intensity inhomogeneity, the internal tumor blocks detection model based on Simple Linear Iterative Clustering(SLIC) and Support Vector Machine (SVM) is learned to detect these segmented blocks. Based on the detection result, the incorrect segmented blocks can be corrected. In addition, BBC is proposed to correct the erroneous segmentation of boundary blocks which are caused by shadowing artifacts. Our experiment results on the constructed database demonstrate the effectiveness and robustness of the proposed method.
更多查看译文
关键词
Image segmentation, correction learning, internal tumor block correction, boundary block correction
AI 理解论文
溯源树
样例
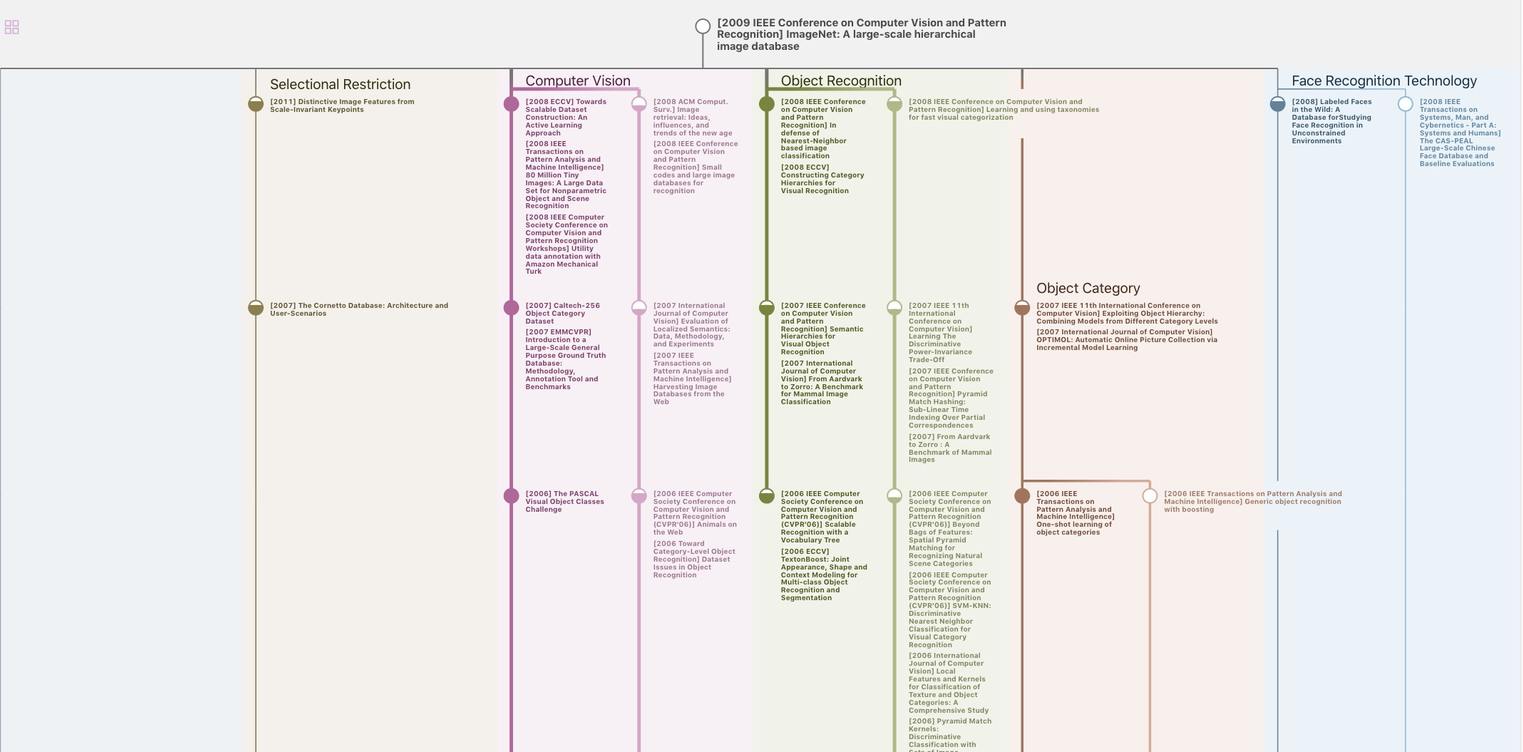
生成溯源树,研究论文发展脉络
Chat Paper
正在生成论文摘要