Robust Generalized Fuzzy Systems Training From High-Dimensional Time-Series Data Using Local Structure Preserving PLS
IEEE Transactions on Fuzzy Systems(2020)
摘要
Establishing fuzzy models from time-series data with predictive capabilities for numerical targets typically requires dimension reduction techniques to overcome the severe curse of dimensionality effects. Linear projection methods are promising candidates in this context as they-unlike nonlinear dimension reduction techniques-preserve interpretability of the resulting models. However, linear projections do not reveal the inherent (nonlinear, local) cluster structure of the data and are thus not ideally suited for the identification of fuzzy rule bases. To overcome this limitation, in this article, we present a new fuzzy modeling approach that combines generalized fuzzy systems modeling with a local structure preserving variant of partial least squares (PLS). In contrast to ordinary PLS, our approach maps a weighted (adjacency) graph on the directions associated with high covariance with the response in order to emphasize local data structures when constructing the latent variable (LV) space. This operates into the direction from which the (training of the) fuzzy model benefits, as therein local regions are represented by submodels in form of generalized Takagi-Sugeno (TS) fuzzy rules. The local structure preserving LV space is obtained by solving a new penalized objective function, which assures global optimality of the solutions by virtue of the specific properties of the Laplacian matrix. Local regions are characterized in two ways: through nearest neighbor points (assuming fixed local region sizes) and through density regions identified by clustering (achieving variable local region sizes). To establish a robust time-series-based forecast model, the training of a generalized TS fuzzy model is conducted in the LV space with reduced dimensionality. It is realized by an iterative robust version of Gen-Smart-EFS, allowing multiple passes over the complete datasets until convergence of the antecedent space. Consequent parameters are estimated by a fuzzily weighted elastic net approach, embedding a convex combination of ridge regression and Lasso to achieve robust solutions also in case of ill-posed problems and meeting the (more stable and interpretable) local learning spirit. The new approach is termed as LS-PLS-Fuzzy, short for local structure preserving PLS fuzzy regression. It was successfully evaluated on three real-world application scenarios including a total of 11 time-series-based prediction modeling problems with different proportions between the number of training samples and original input dimensionality. Our results show significantly improved model accuracy compared to related State-of-the-Art (SoA) modeling approaches, an alternative dimension reduction technique, and when using conventional PLS and/or nongeneralized fuzzy rules.
更多查看译文
关键词
Fuzzily weighted elastic net,generalized fuzzy rules and systems,Laplacian matrix,local structure preserving,partial least squares (PLS) regression,time-series-based forecast models,variable local region sizes
AI 理解论文
溯源树
样例
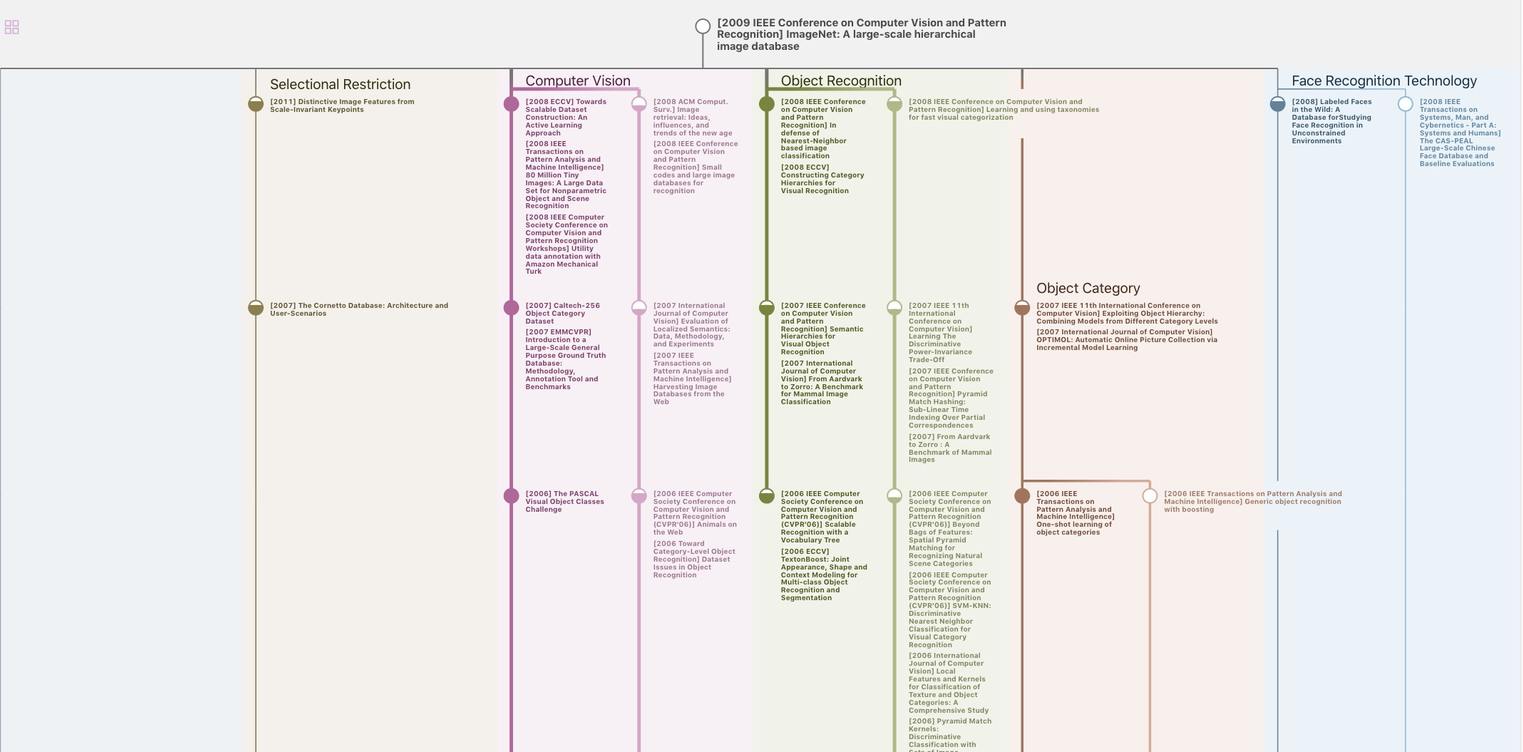
生成溯源树,研究论文发展脉络
Chat Paper
正在生成论文摘要