Learning 3d Semantic Reconstruction On Octrees
PATTERN RECOGNITION, DAGM GCPR 2019(2019)
摘要
We present a fully convolutional neural network that jointly predicts a semantic 3D reconstruction of a scene as well as a corresponding octree representation. This approach leverages the efficiency of an octree data structure to improve the capacities of volumetric semantic 3D reconstruction methods, especially in terms of scalability. At every octree level, the network predicts a semantic class for every voxel and decides which voxels should be further split in order to refine the reconstruction, thus working in a coarse-to-fine manner. The semantic prediction part of our method builds on recent work that combines traditional variational optimization and neural networks. In contrast to previous networks that work on dense voxel grids, our network ismuch more efficient in terms of memory consumption and inference efficiency, while achieving similar reconstruction performance. This allows for a high resolution reconstruction in case of limited memory. We perform experiments on the SUNCG and ScanNetv2 datasets on which our network shows comparable reconstruction results to the corresponding dense network while consuming less memory.
更多查看译文
关键词
3d semantic reconstruction
AI 理解论文
溯源树
样例
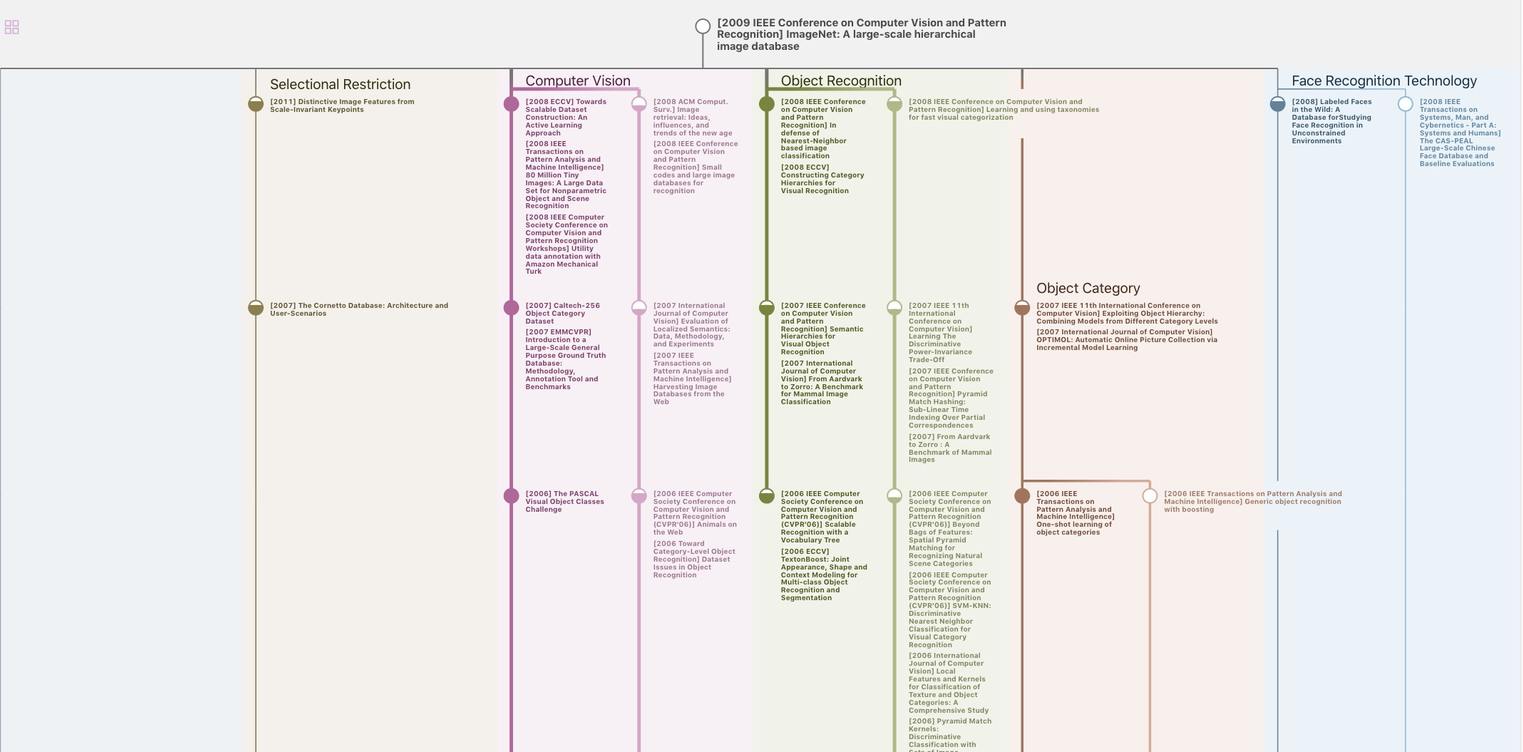
生成溯源树,研究论文发展脉络
Chat Paper
正在生成论文摘要