DEPA: Self-Supervised Audio Embedding for Depression Detection
International Multimedia Conference(2021)
摘要
ABSTRACTDepression detection research has increased over the last few decades, one major bottleneck of which is the limited data availability and representation learning. Recently, self-supervised learning has seen success in pretraining text embeddings and has been applied broadly on related tasks with sparse data, while pretrained audio embeddings based on self-supervised learning are rarely investigated. This paper proposes DEPA, a self-supervised, pretrained dep ression a udio embedding method for depression detection. An encoder-decoder network is used to extract DEPA on in-domain depressed datasets (DAIC and MDD) and out-domain (Switchboard, Alzheimer's) datasets. With DEPA as the audio embedding extracted at response-level, a significant performance gain is achieved on downstream tasks, evaluated on both sparse datasets like DAIC and large major depression disorder dataset (MDD). This paper not only exhibits itself as a novel embedding extracting method capturing response-level representation for depression detection but more significantly, is an exploration of self-supervised learning in a specific task within audio processing.
更多查看译文
关键词
depression,audio,self-supervised
AI 理解论文
溯源树
样例
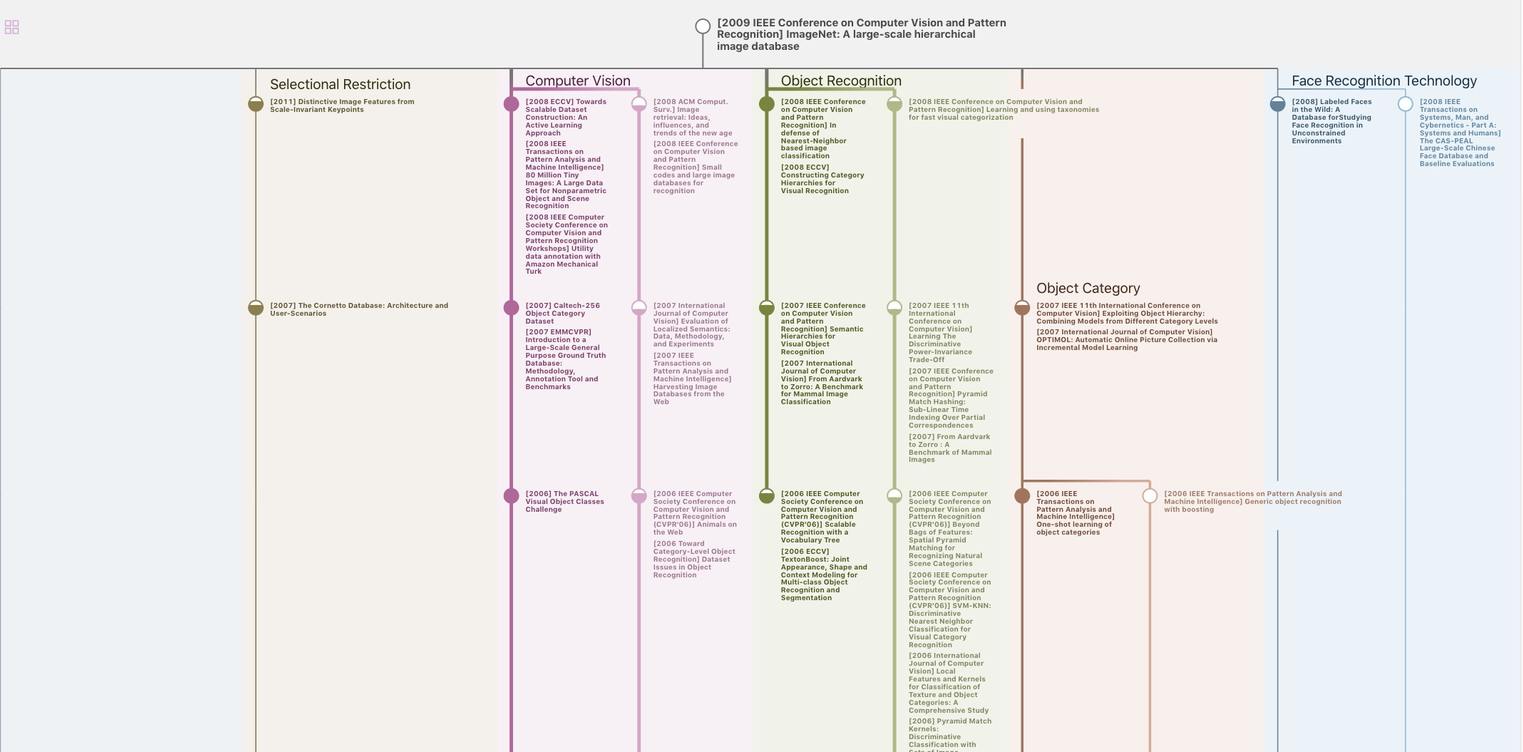
生成溯源树,研究论文发展脉络
Chat Paper
正在生成论文摘要